An Information-Expanding Network for Water Body Extraction Based on U-Net
IEEE GEOSCIENCE AND REMOTE SENSING LETTERS(2024)
摘要
Water body extraction is an important issue in flood surveillance and environmental protection. With the development of neural network, deep learning has been widely used in the water body extraction task because of its powerful feature extraction ability. Even so, most of existing deep learning networks only take into account the translation equivariance of convolution kernel for water body extraction. Actually, in terms of water body, its orientations imaged by optical sensor are usually various. So, when the orientated images are not well contained in the training set, the networks may yield some unsatisfactory extraction results. To solve this problem, in this letter, we propose an information-expanding network IE-Unet based on the traditional network U-net, where the rotation equivariant convolution, rotation-based channel attention mechanism, and the optimized Batchnorm are adopted jointly. To quantitatively evaluate its edge extraction capability, a new edge index average offset distance (AOD) is proposed as well. The experimental results on one public dataset of water body demonstrate the effectiveness of IE-Unet. Compared with the original U-net, the intersection over union (IOU) value of IE-Unet is increased by 7%, and the AOD value is reduced by 0.76.
更多查看译文
关键词
Convolution,Feature extraction,Data mining,Training,Indexes,Kernel,Image edge detection,Average offset distance (AOD),IE-Unet,rotation equivariant convolution,rotation-based channel attention mechanism,U-net
AI 理解论文
溯源树
样例
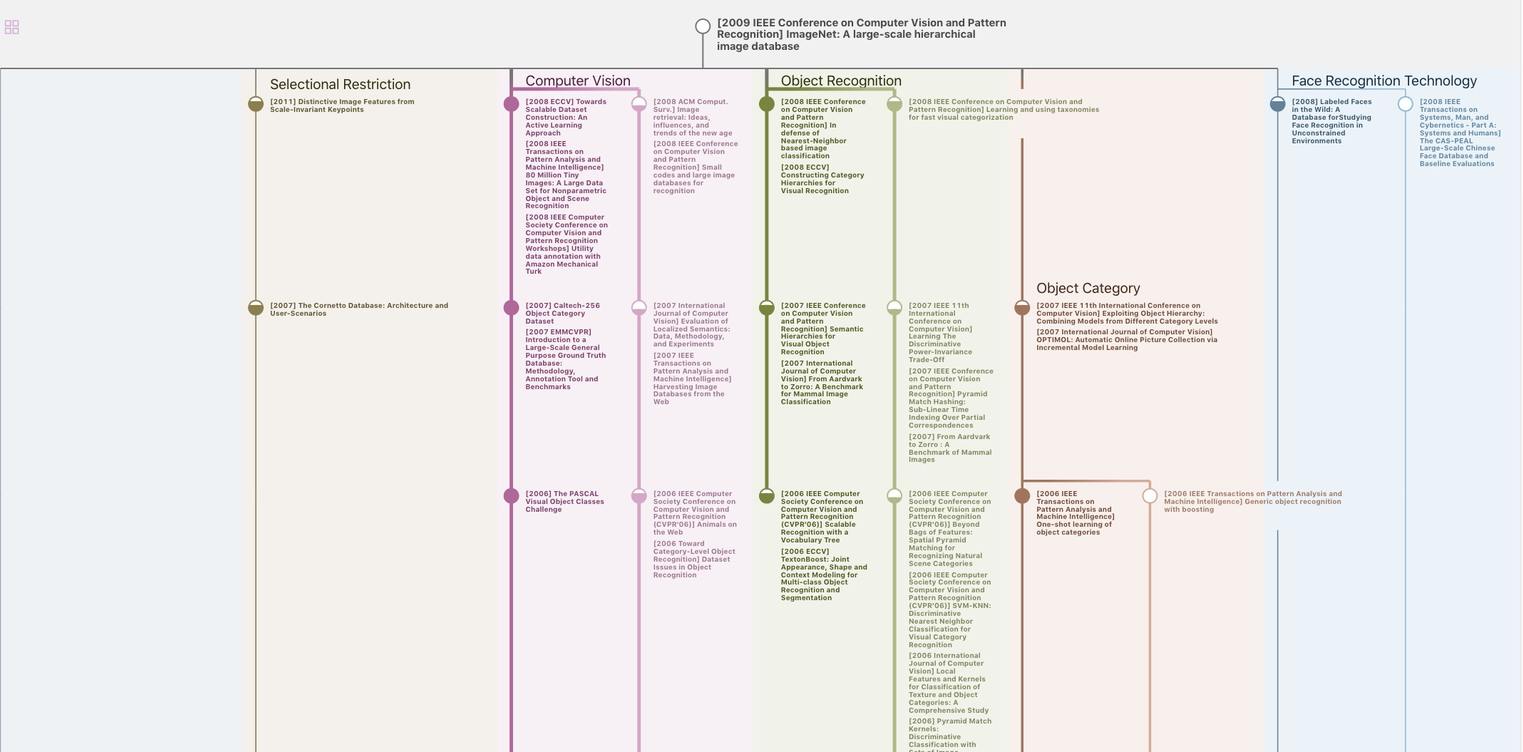
生成溯源树,研究论文发展脉络
Chat Paper
正在生成论文摘要