A deep learning approach to censored regression
Pattern Analysis and Applications(2024)
摘要
In censored regression, the outcomes are a mixture of known values (uncensored) and open intervals (censored), meaning that the outcome is either known with precision or is an unknown value above or below a known threshold. The use of censored data is widespread, and correctly modeling it is essential for many applications. Although the literature on censored regression is vast, deep learning approaches have been less frequently applied. This paper proposes three loss functions for training neural networks on censored data using gradient backpropagation: the tobit likelihood, the censored mean squared error, and the censored mean absolute error. We experimented with three variations in the tobit likelihood that arose from different ways of modeling the standard deviation variable: as a fixed value, a reparametrization, and an estimation using a separate neural network for heteroscedastic data. The tobit model yielded better results, but the other two losses are simpler to implement. Another central idea of our research was that data are often censored and truncated simultaneously. The proposed losses can handle simultaneous censoring and truncation at arbitrary values from above and below.
更多查看译文
关键词
Censored regression,Truncation,Deep learning,Neural network,Tobit
AI 理解论文
溯源树
样例
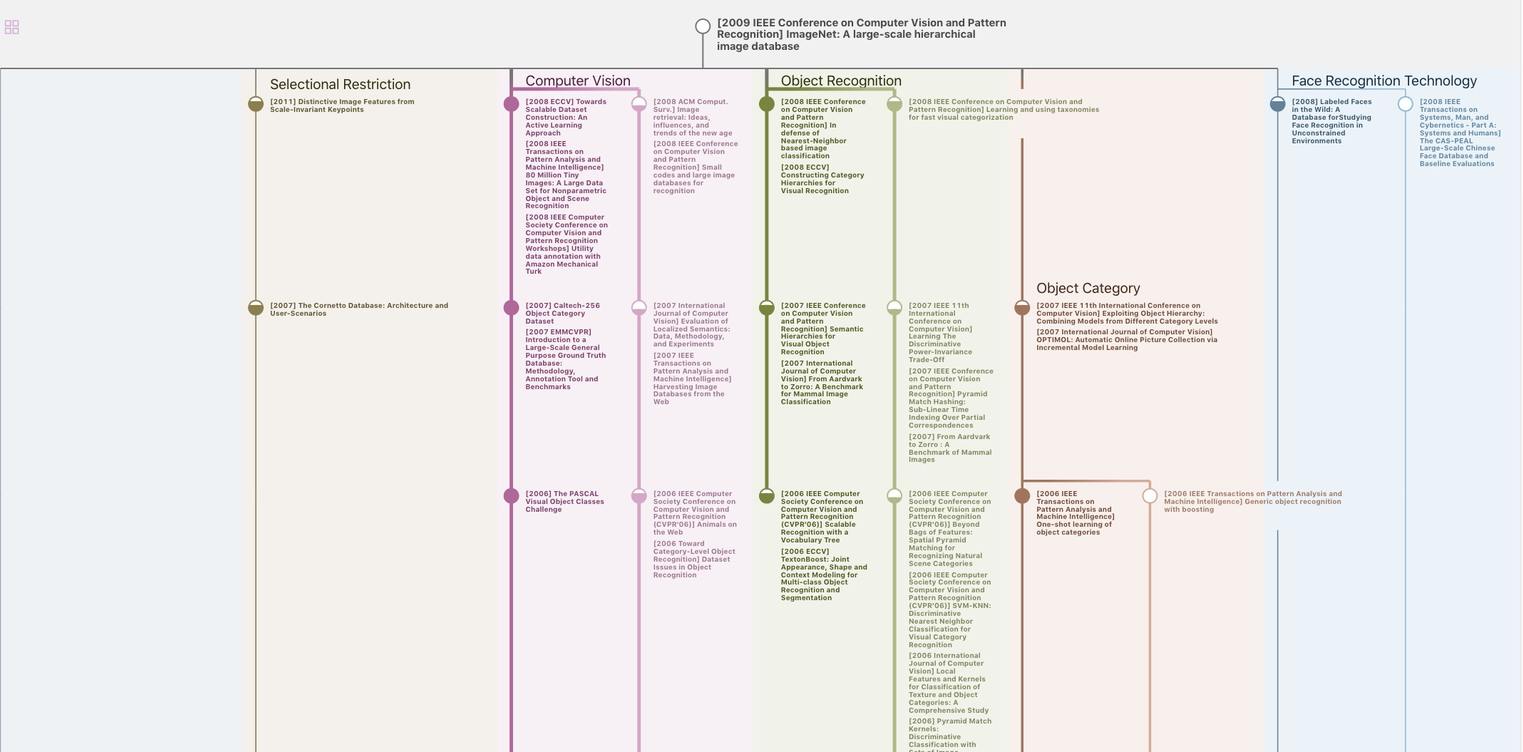
生成溯源树,研究论文发展脉络
Chat Paper
正在生成论文摘要