MGFEEN: a multi-granularity feature encoding ensemble network for remote sensing image classification
Neural Computing and Applications(2024)
摘要
Deep convolutional neural networks (DCNNs) have emerged as powerful tools in diverse remote sensing domains, but their optimization remains challenging due to their complex nature and the large number of parameters involved. Researchers have been exploring more sophisticated methodologies to improve image classification accuracy. In this paper, we introduce a multi-granularity feature encoding ensemble network (MGFEEN) that is designed to fine-tune features at different levels of granularity. The network is trained in a two-step process: First, the output of granularity level i is used as the input for the next level; then, a fully connected layer is added to the pre-trained network to advance to the next level. The effectiveness of the MGFEEN’s feature extraction is evaluated by feeding the globally extracted features to a softmax classifier for classification. By applying ensemble learning principles, our proposed MGFEEN achieves more accurate final predictions. We evaluate our model on three widely recognized benchmark datasets: UC-Merced, SIRIWHU, and EAC-Dataset. Notably, on the EAC-Dataset, our results show a significant 0.54% improvement in accuracy over a single-training-network setup, resulting in an impressive 98.70% accuracy level.
更多查看译文
关键词
Multi-granularity feature representation,Convolution neural network,Feature ensemble network,Remote sensing image classification
AI 理解论文
溯源树
样例
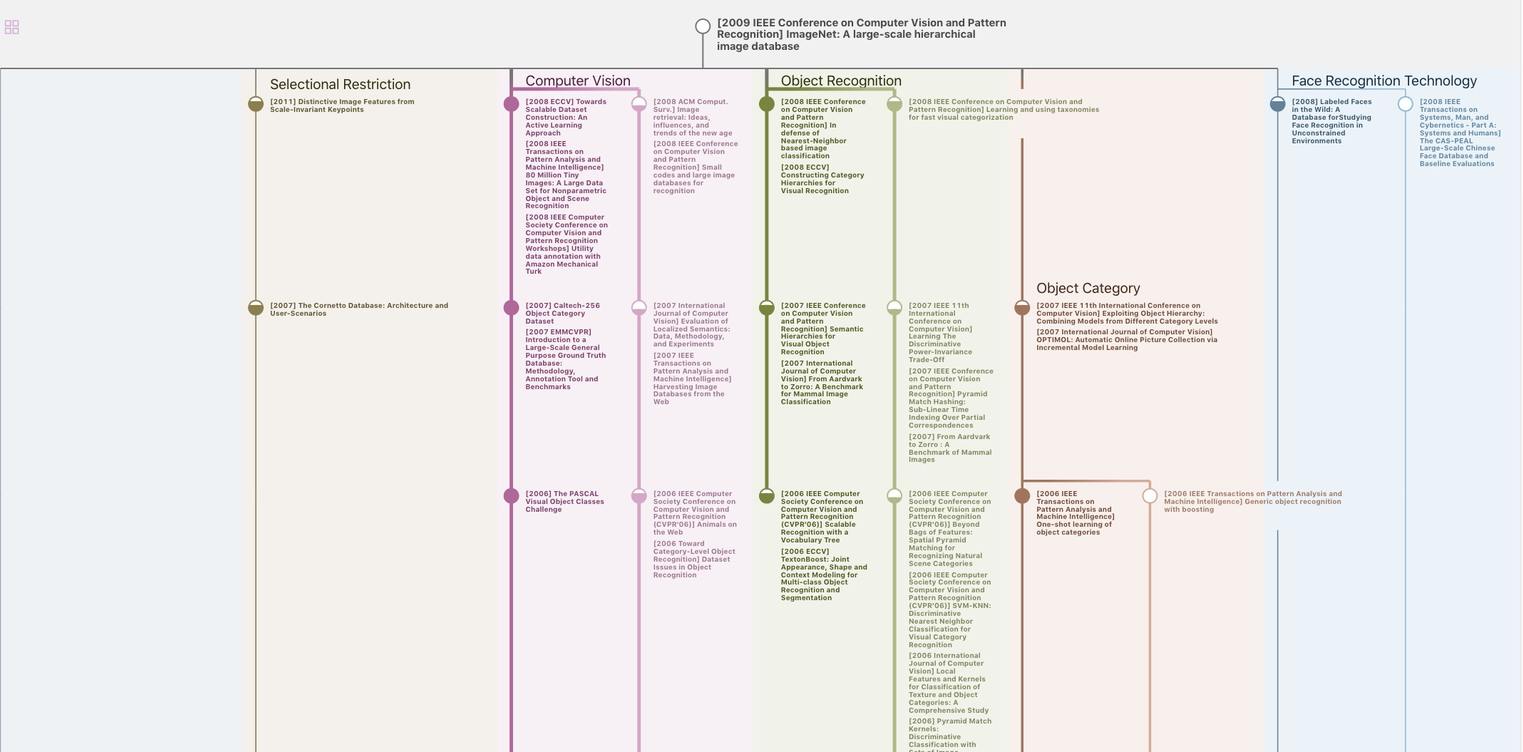
生成溯源树,研究论文发展脉络
Chat Paper
正在生成论文摘要