Multi-fidelity error-estimate-based model management
Structural and Multidisciplinary Optimization(2024)
摘要
This paper presents a novel multi-fidelity model-management framework based on the estimated error between the low-fidelity and high-fidelity models. The optimization algorithm is similar to classical multi-fidelity trust-region model-management approaches, but it replaces the trust-radius constraint with a bound on the estimated error between the low- and high-fidelity models. This enables globalization without requiring the user to specify non-intuitive parameters such as the initial trust radius, which have a significant impact on the cost of the optimization yet can be hard to determine a priori. We demonstrate the framework on a simple one-dimensional optimization problem, a series of analytical benchmark problems, and a realistic electric-motor optimization. We show that for low-fidelity models that accurately capture the trends of the high-fidelity model, the developed framework can significantly improve the efficiency of obtaining high-fidelity optima compared to state-of-the-art multi-fidelity optimization methods and a direct high-fidelity optimization.
更多查看译文
关键词
Multi-fidelity optimization,Electric-motor optimization,Error estimates
AI 理解论文
溯源树
样例
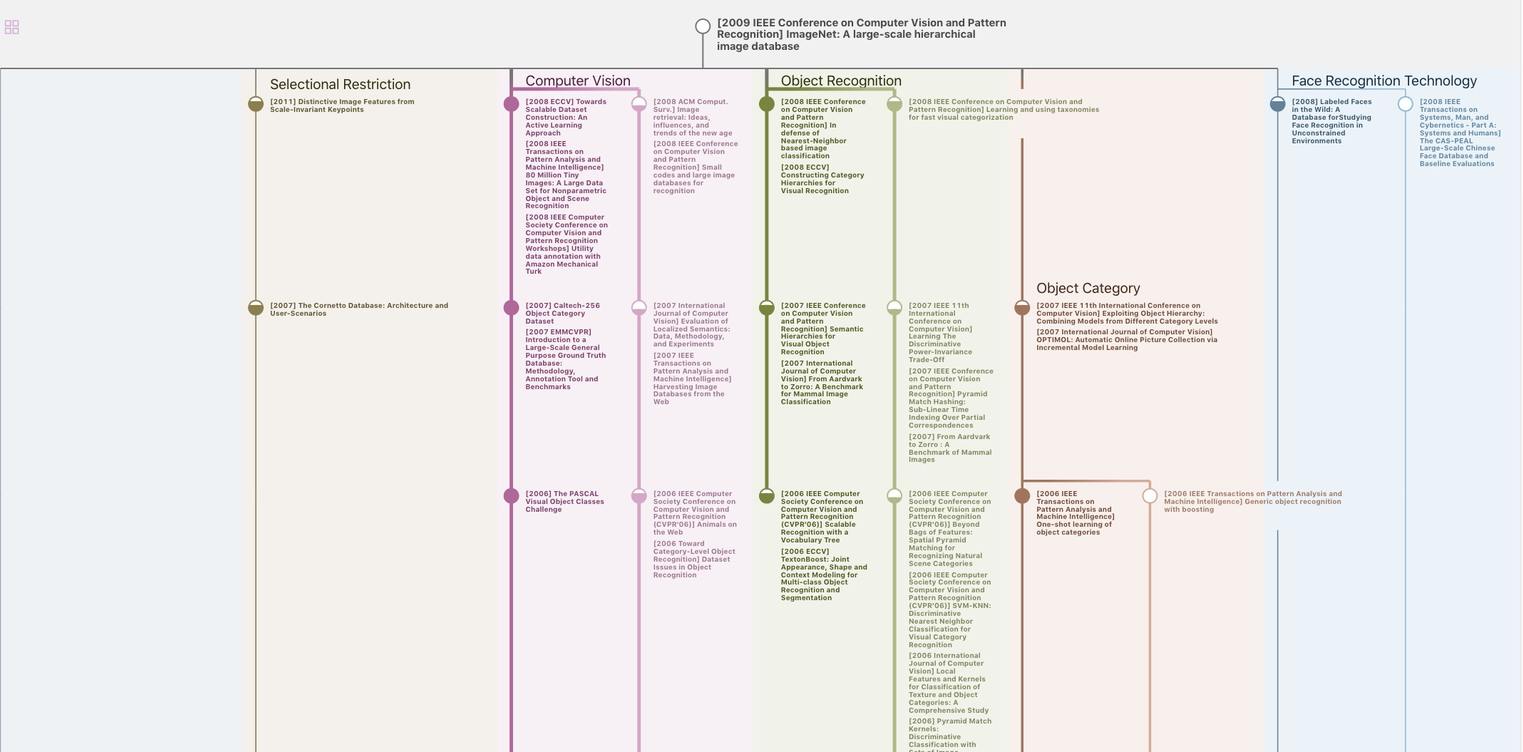
生成溯源树,研究论文发展脉络
Chat Paper
正在生成论文摘要