An Interpretable CNN With Wavelet Group Policy Embedded for Intelligent Fault Diagnosis.
IEEE Trans. Instrum. Meas.(2024)
摘要
Interpretable convolutional neural networks (CNNs) are key to reliable industrial fault diagnosis by elucidating model decision-making processes and extracting high-dimensional data features. Presently, interpretable CNNs include time-frequency transformation methods in convolutional layers, but their hyperparameter setting (e.g., window size, overlap, and type of wavelet) depends largely on expert knowledge. This article focuses on the problem of wavelet type selection, we propose a wavelet shrinkage convolutional network (GP-WSCN) based on a group policy to solve this problem. Initially, GP-WSCN creates a pretrained network with wavelet convolution layers and soft-threshold learning, quickly providing a basic prior for diverse wavelet waveforms. This prior knowledge is combined with reinforcement learning (RL), allowing GP-WSCN to independently select suitable wavelet convolution kernels, reducing expert dependency. The pretrained network is then repurposed and fine-tuned to selected kernels for swift GP-WSCN deployment in fault diagnosis tasks. Experimental results confirm the diagnostic precision and interpretability of GP-WSCN, as proven through multiple trials.
更多查看译文
关键词
Convolutional neural network (CNN),intelligent fault diagnosis,interpretability,wavelet group policy
AI 理解论文
溯源树
样例
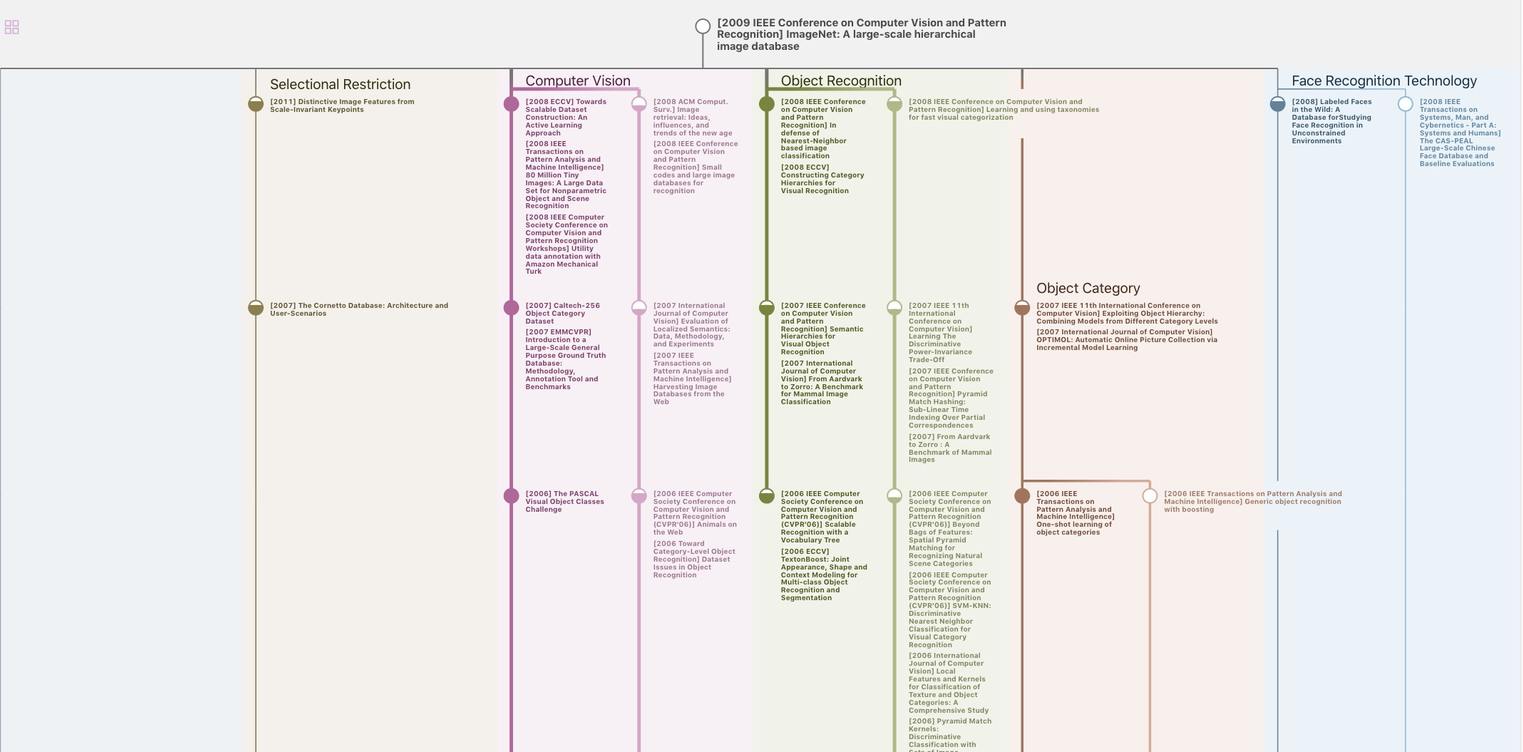
生成溯源树,研究论文发展脉络
Chat Paper
正在生成论文摘要