Intrinsic Property and Common Information Extraction for Unsupervised Multiplex Graph Learning
2023 IEEE International Conference on Electrical, Automation and Computer Engineering (ICEACE)(2023)
摘要
Unsupervised multiplex graph learning (UMGL) has emerged as an important research topic, but previous UMGL methods continue to face the following challenges: (i) contrastive learning-based methods necessitate negative samples, which can be costly, and the selection of negative samples substantially affects performance, and (ii) traditional UMGL methods often prioritize structural information excessively while neglecting node characteristics. This imbalance makes them susceptible to noise caused by incorrect links. To tackle these challenges, we introduce a potent and efficient UMGL method aimed at extracting the intrinsic properties and common information of the multiplex graph simultaneously, while reducing redundant information of node representations. We introduce the attention mechanism to fuse local structure and node characteristics with no need to build negative samples. Comprehensive experiments on four multiplex graph datasets demonstrate that our method excels in comparison to the other multiplex graph embedding models in the node classification task.
更多查看译文
关键词
Knowledge Graph,Representation Learning,Multiplex Graph
AI 理解论文
溯源树
样例
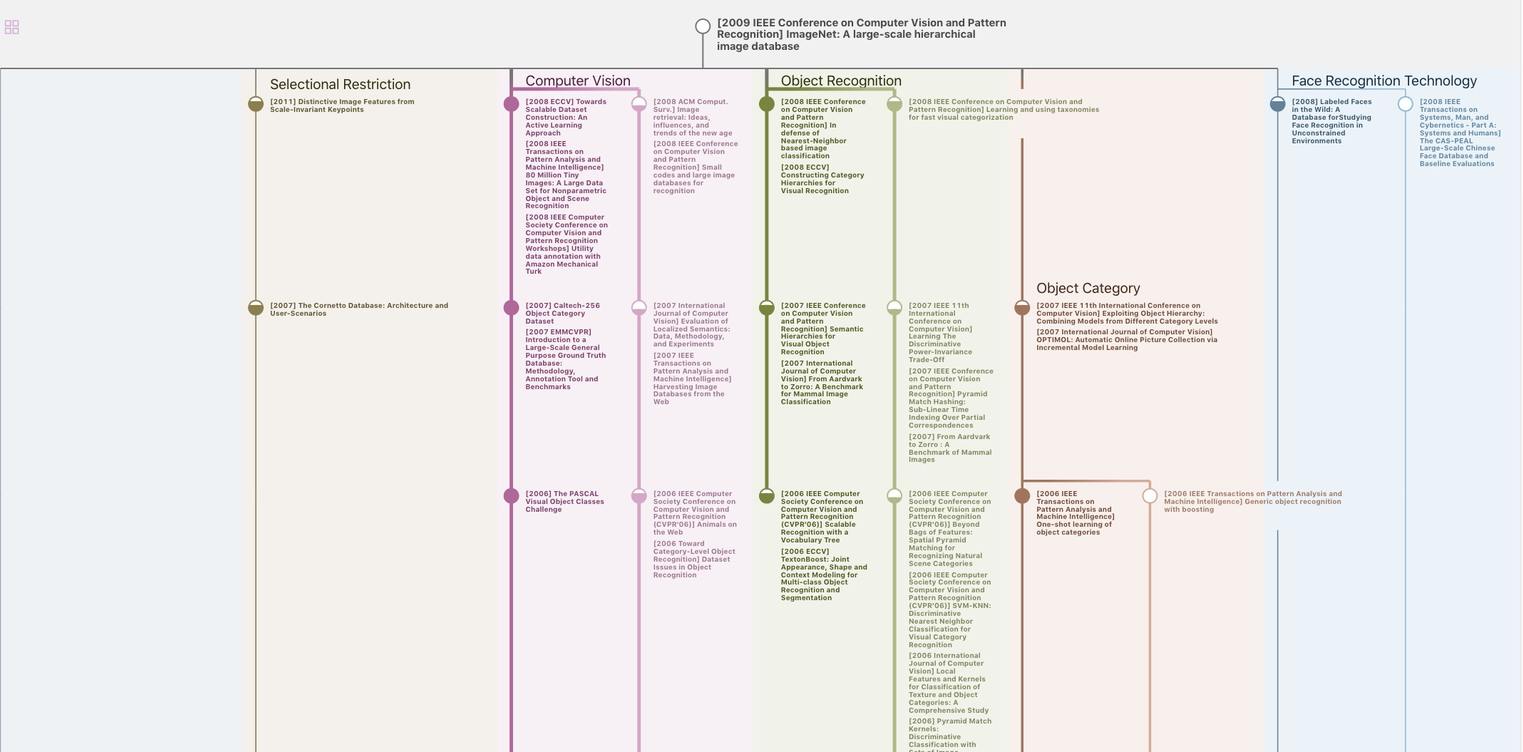
生成溯源树,研究论文发展脉络
Chat Paper
正在生成论文摘要