ARFN: An Attention-Based Recurrent Fuzzy Network for EEG Mental Workload Assessment
IEEE TRANSACTIONS ON INSTRUMENTATION AND MEASUREMENT(2024)
摘要
Assessing mental workload using electroencephalogram (EEG) signals is a significant research avenue within the brain-computer interface (BCI) domain. However, due to the low signal-to-noise ratio in EEG signals and the interindividual variability in EEG data acquisition, achieving high accuracy and generalization in feature extraction and classification for mental workload assessment is still challenging. We propose a novel deep-learning framework named attention-based recurrent fuzzy network (ARFN) for EEG mental workload assessment. In ARFN, we adopt a fuzzy recursive module that employs a feature attention mechanism and a fuzzy rule attention mechanism, respectively, to flexibly extract EEG features related to mental workload. The former can extract the frequency-domain features of EEG signals, while the latter is used to represent the membership degrees within the distribution of frequency features, to find effective fuzzy rules for classification. Subsequently, the output of the fuzzy recursive module is directed into the long short-term memory (LSTM) to further extract temporal features of the EEG, followed by a fully connected layer and the Softmax function for classification. The experimental results on three public datasets show that ARFN outperforms other state-of-the-art models in EEG mental workload assessment.
更多查看译文
关键词
Feature extraction,Electroencephalography,Brain modeling,Fuzzy neural networks,Human factors,Fatigue,Data models,Attention mechanism,electroencephalogram (EEG),fuzzy neural network (FNN),mental workload
AI 理解论文
溯源树
样例
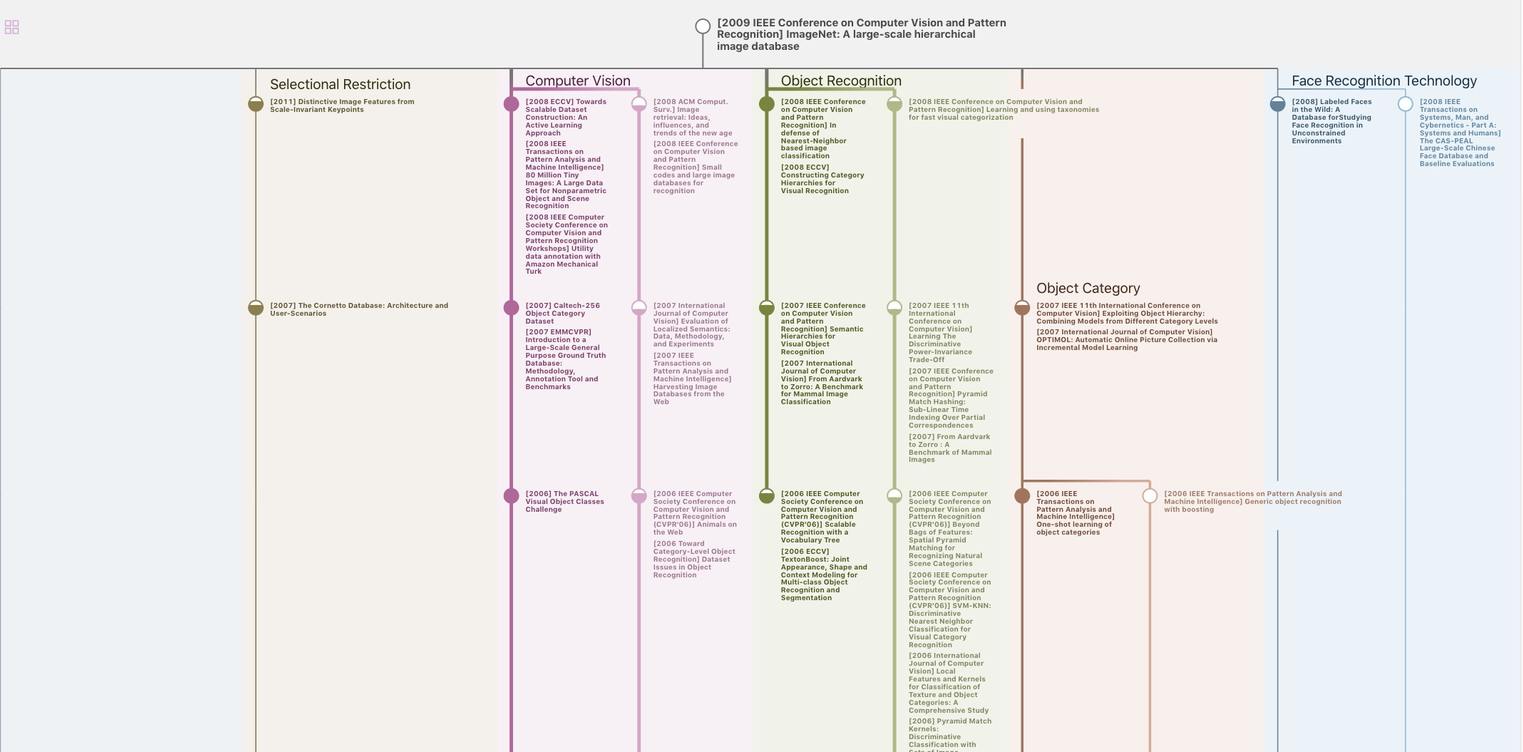
生成溯源树,研究论文发展脉络
Chat Paper
正在生成论文摘要