Simulated Breathing Patterns Classification Using Convolutional Neural Network with Deep Embedded Features.
IEEE International Conference on Consumer Electronics(2024)
Abstract
The global outbreak of the coronavirus pandemic in 2019, specifically the COVID-19 disease, underscored the necessity for remote methods to detect and monitor patients with infectious respiratory disease without direct contact. Infectious respiratory infection patients commonly exhibit symptoms such as coughing, fever, and shallow and rapid breathing. Various methods using CT images, X-rays, depth camera, and so on have been proposed to monitor these symptoms. However, these methods typically cannot perform automatic monitoring around the clock. In this study, we present a novel classification model designed to classify breathing patterns based on data obtained through a wearable near-infrared spectroscopy (NIRS) device. The proposed method uses a deep autoencoder to generate embedded represented features, and these features are used in a convolutional neural network (CNN)-based classification model to help learn the parameters of the deep neural network. In the experimental results, the proposed model achieved a classification accuracy of 92.34%.
MoreTranslated text
Key words
convolutional neural network,respiratory disease,NIRS
AI Read Science
Must-Reading Tree
Example
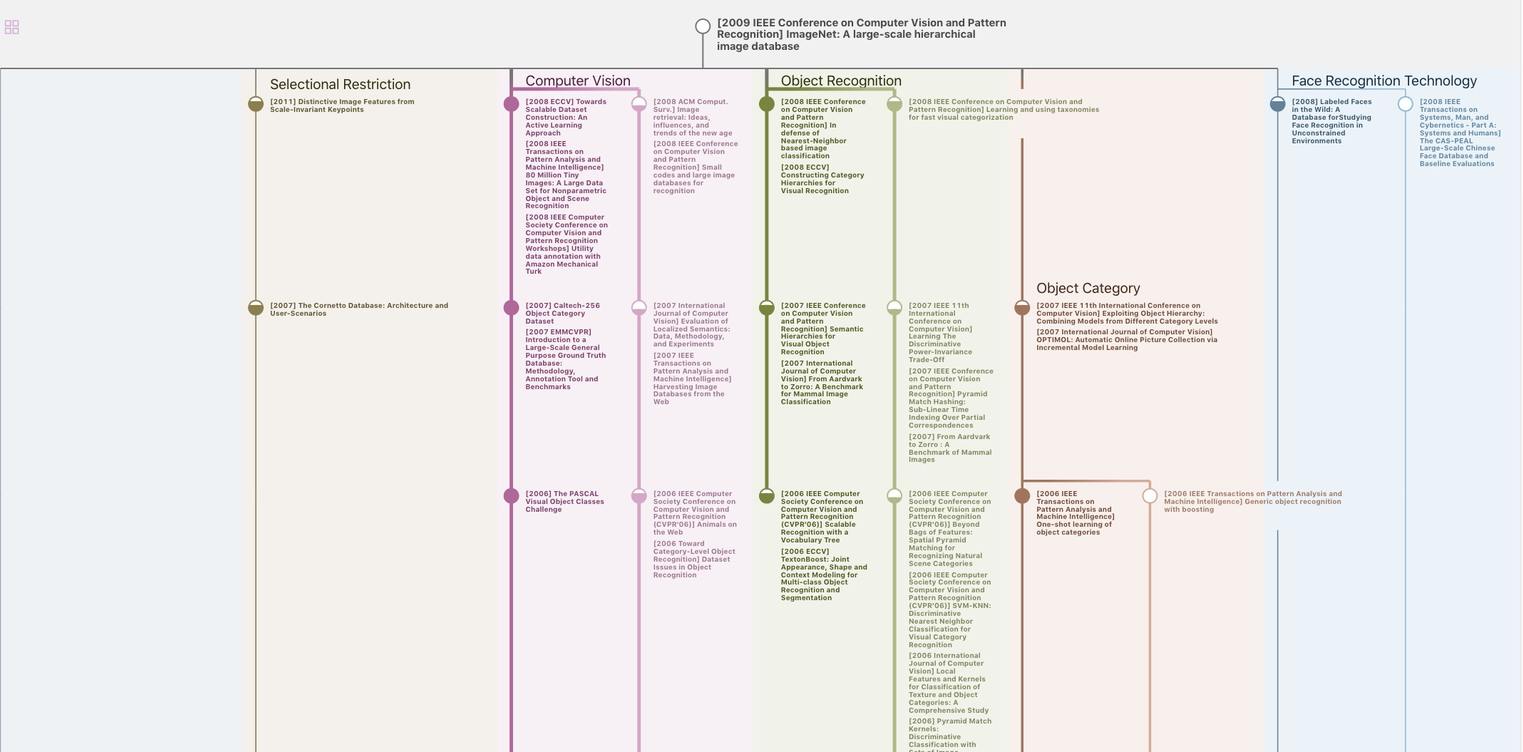
Generate MRT to find the research sequence of this paper
Chat Paper
Summary is being generated by the instructions you defined