Maximizing Energy Efficiency of Mobile Biomechanical Decoding with Spatial Variability Mining.
IEEE International Conference on Consumer Electronics(2024)
摘要
Mobile sensing and data analytics usually take a substantial amount of energy, which limits the durability of the wearable devices. Especially, when deep learning is applied for data mining, the energy need is even more hungry. In this study, we take a special interest in wearable biomechanical data analytics, and propose to leverage data mining to tackle this challenge. More specifically, we leverage deep learning to mine the spatial variability of motion sensors embedded in mobile devices placed on the forearm and upper arm. In addition to compare the sensor locations, we further compare six different channels of each sensor location, thereby making twelve different configurations. Ultimately, we determine the optimal sensor location and the optimal sensor channel, which indicates the minimized data processing need in the real-world applications. The results indicate that the upper arm location and Y-axis of the accelerometer is the optimal configuration. This study will advance the field of maximizing energy efficiency of mobile biomechanical monitors, towards continuous data-driven precision medicine.
更多查看译文
关键词
Mobile Devices,Deep Learning,Spatial Variability,Big Data
AI 理解论文
溯源树
样例
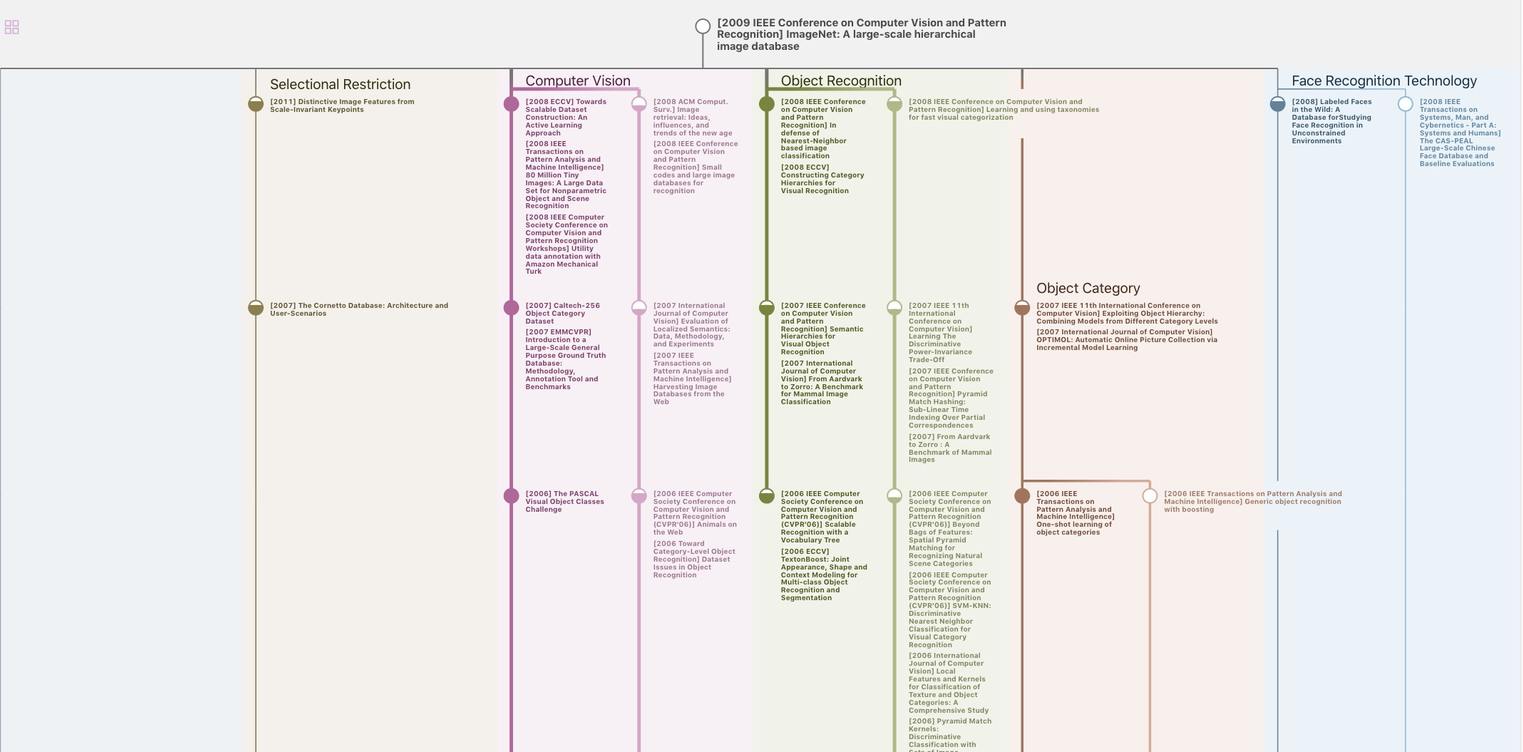
生成溯源树,研究论文发展脉络
Chat Paper
正在生成论文摘要