Joint Client Scheduling and Quantization Optimization in Energy Harvesting-Enabled Federated Learning Networks
IEEE Transactions on Wireless Communications(2024)
摘要
A vital challenge in the deployment of federated learning (FL) over wireless networks is the high energy consumption incurred for the local computation and model update upload on energy-constrained devices such as IoT sensors. Equipping with energy harvesting (EH) modules is a promising solution that allows the devices to work in a self-sustainable manner. Moreover, quantizing the model updates can further improve the energy efficiency during the upload. In this paper, we propose an EH-enabled FL system with model quantization in which EH devices act as clients and client scheduling, model quantization, and transmit energy are jointly optimized to minimize the training loss while satisfying energy causality constraints and guaranteeing fairness in client selection. We formulate a non-convex mixed-integer nonlinear programming (MINLP) problem for the optimization. Then, by recasting the product of a continuous variable and a 0-1 variable in an equivalent linear form, we transform this non-convex MINLP problem into a convex problem and solve it. We present numerical evaluations on various datasets to show that our proposed system is stable and achieves high performance regardless of whether the loss function is convex or non-convex and whether the data distributions are independent and identically distributed (i.i.d.) or non-i.i.d.
更多查看译文
关键词
Federated learning,client scheduling,quantization,fairness,energy harvesting
AI 理解论文
溯源树
样例
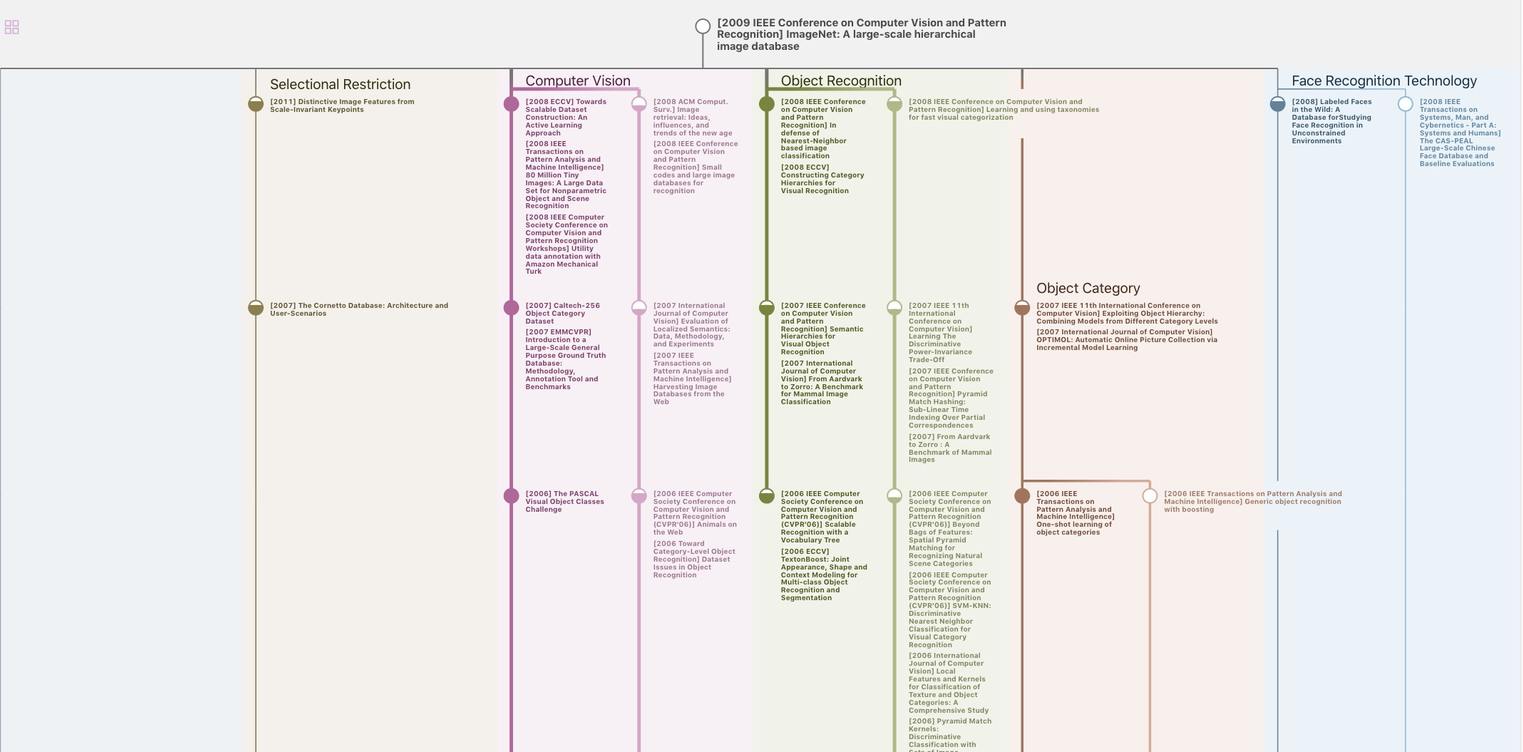
生成溯源树,研究论文发展脉络
Chat Paper
正在生成论文摘要