Intelligent Anti-jamming based on Deep Reinforcement Learning and Transfer Learning
IEEE Transactions on Vehicular Technology(2024)
摘要
One of the security issues in a wireless network is jamming attacks, where the jammer causes congestion and significant decrement in the network throughput by obstructing channels and disrupting user signals. Recent works have proposed using a deep-reinforcement learning (DRL) model to confront the jamming attacker due to its capability in predicting the jammer decisions and pattern recognition. Training a DRL model from scratch may take a long time. We first propose a recurrent neural network architecture to minimize the number of parameters for training a DRL model. We further propose a transfer learning (TL) approach to enable the DRL agent to learn fast in dynamic wireless networks to confront jamming attacks effectively. To make our proposed TL method adaptive to different network environments, we propose a novel method to quantitatively measure the difference between the source and target domains, using an integrated feature extractor. Afterward, based on the measured difference, we can choose an optimal setting for the TL model. We also show that the proposed TL method can effectively reduce the training time for the DRL model and outperforms other existing TL methods.
更多查看译文
关键词
Transfer Learning,Reinforcement Learning,Wireless Network Security,explainable artificial intelligence,explainable reinforcement learning
AI 理解论文
溯源树
样例
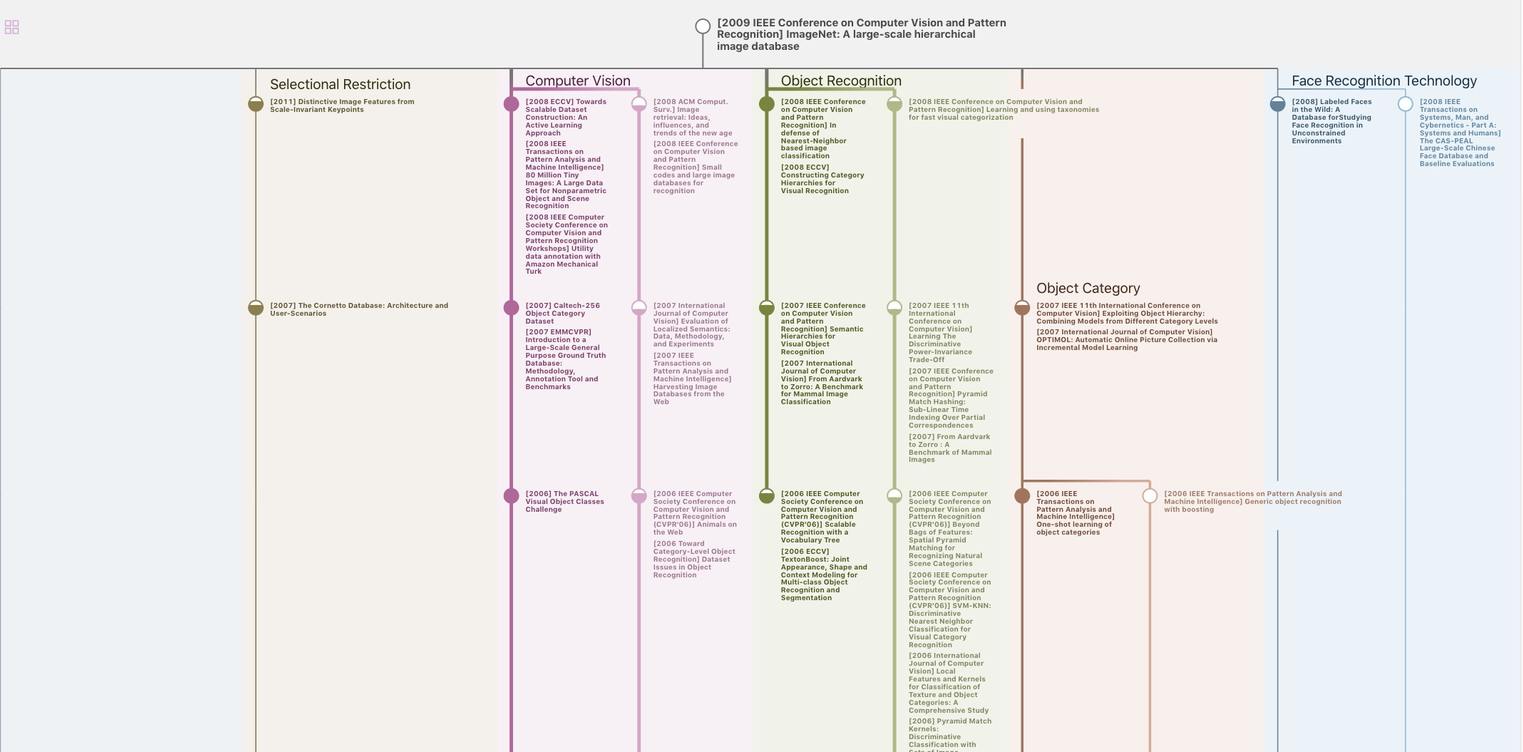
生成溯源树,研究论文发展脉络
Chat Paper
正在生成论文摘要