Dual-Driven Learning for Channel Estimation of Massive MIMO Systems With One-Bit ADCs
IEEE Wireless Communications Letters(2024)
摘要
This letter proposes a dual-driven learning network for channel estimation of massive multiple-input-multiple-output (MIMO) systems with one-bit analog-to-digital converters (ADCs) utilizing the combination of a data-driven quantization correction network and a model-driven residual learned approximate message passing (RLAMP) network. The data-driven network can reduce quantization errors to reduce residual errors passed in each iteration of the model-driven network when the whole dual-driven network has been trained jointly. Numerical results demonstrate that the dual-driven estimator outperforms conventional data-driven or model-driven approaches. The proposed dual-driven estimator can save approximately 60% of the pilots needed when the signal noise ratio (SNR) is 20 dB.
更多查看译文
关键词
Channel estimation,dual-driven,one-bit analog-to-digital converters (ADCs),massive multiple-input-multiple-output (MIMO)
AI 理解论文
溯源树
样例
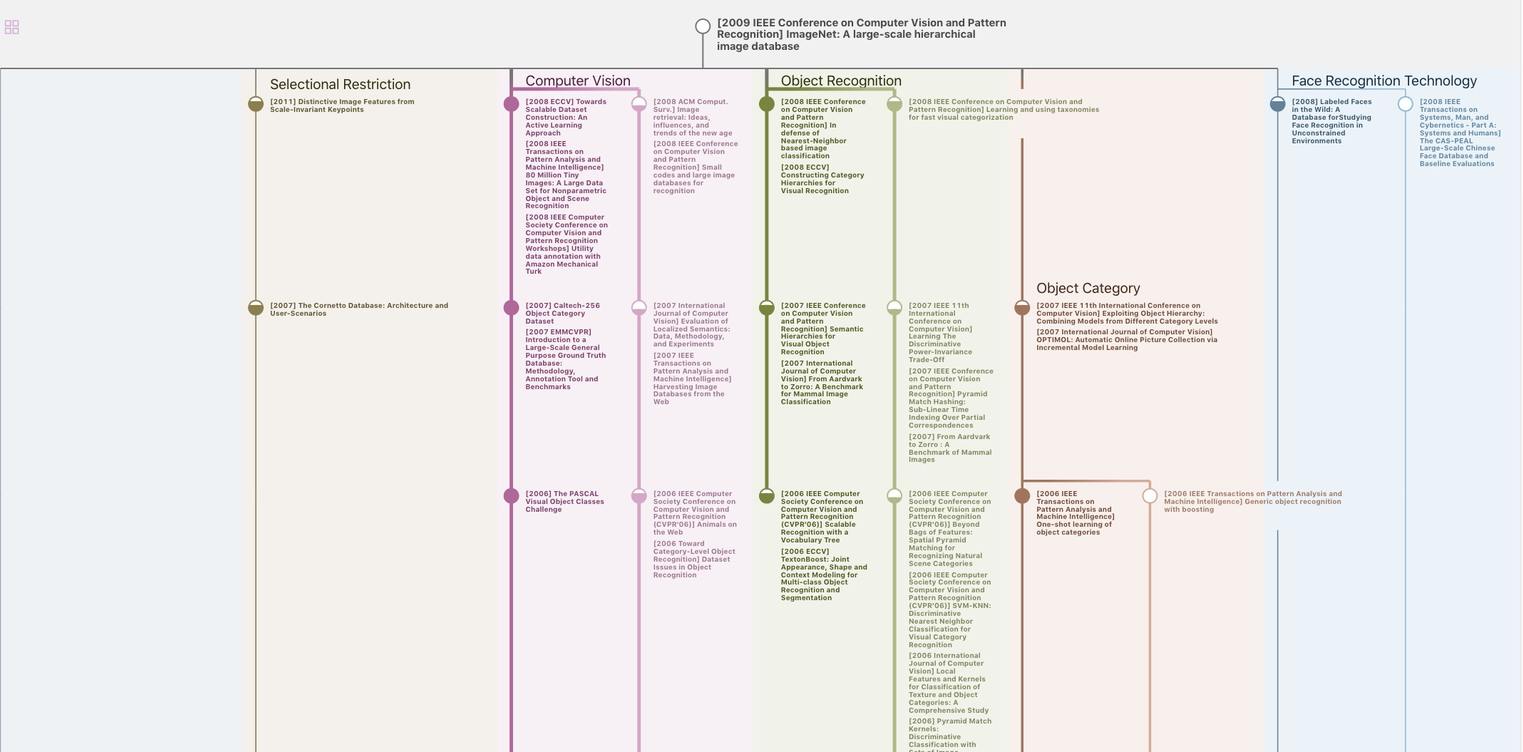
生成溯源树,研究论文发展脉络
Chat Paper
正在生成论文摘要