Deep Learning Based CFO Estimation for Multi-User Massive MIMO With One-Bit ADCs
IEEE Wireless Communications Letters(2024)
摘要
Low-resolution architectures represent a compelling and power-efficient approach for high-bandwidth communication in massive multiple-input multiple-output (MIMO) systems. In this letter, we present a novel residual convolutional neural network (CNN) with recurrent neural network (RNN) called ResR model to tackle the carrier frequency offset (CFO) problem in multi-user massive MIMO with one-bit analog-to-digital converters (ADCs). Leveraging the combined strengths of residual CNN and RNN, the ResR model can extract frequency-spatial characteristics of all users for CFO estimation. Moreover, it effectively addresses the vanishing gradient problem in CNN-based model while delivering superior accuracy with fewer parameters compared to exiting CNN or RNN models. Through extensive experimental evaluations, we consistently demonstrate the efficiency and robustness of the ResR model in multi-user CFO estimation for one-bit ADCs massive MIMO.
更多查看译文
关键词
carrier frequency offset (CFO),deep learning,multiple-input multiple-output (MIMO),one-bit analog-to-digital converter (ADCs),residual network
AI 理解论文
溯源树
样例
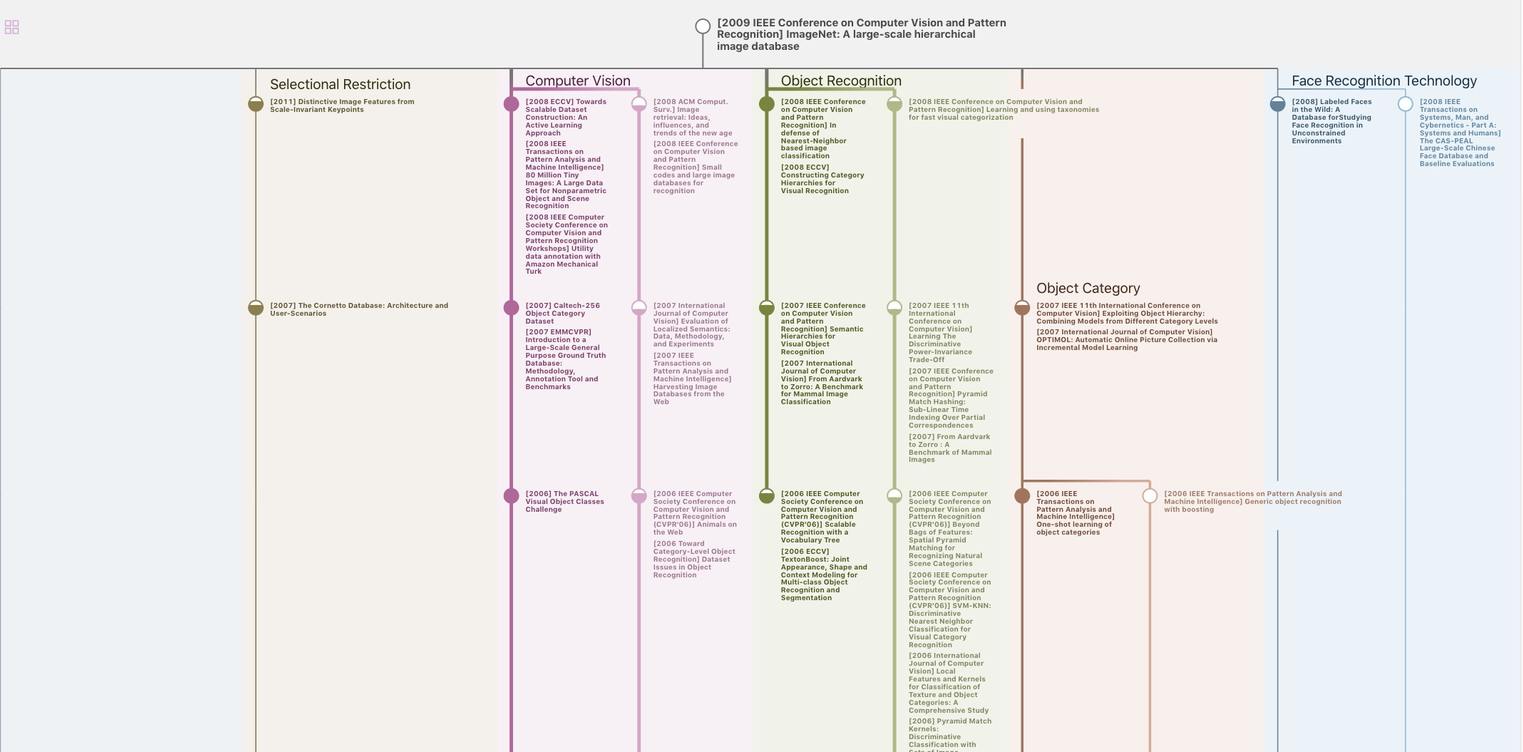
生成溯源树,研究论文发展脉络
Chat Paper
正在生成论文摘要