Wasserstein Distance-assisted Variational Bayesian Kalman Filter with Statistical Similarity Measure for GNSS/INS Integrated Navigation
IEEE Sensors Journal(2024)
Abstract
Kalman filters are affected by outliers and inaccurate measurement noise covariance matrices (MNCMs). However, a robust adaptive filter cannot rapidly track sudden changes in the statistical characteristics of noise. Moreover, robust estimation introduces amplified MNCMs into adaptive estimation. These factors will lead to an estimation delay. Thus, we propose a Wasserstein distance (W distance)-assisted variational Bayesian Kalman filter with a statistical similarity measure (VBSSM) that aims to provide a unified algorithm for adaptive robust filters and enhance a filter’s ability to identify and respond to outliers and abnormal MNCMs. By employing the windowing method, the states of both measurements and time-varying MNCMs are scalarized using the W distance. Subsequently, parallel processing of outliers and MNCMs is achieved based on a threshold. Simulation and field test results demonstrate the adaptability and robustness of the proposed algorithm, which not only avoids the impact of outliers but also rapidly tracks the time-varying MNCMs. Finally, it improves global navigation satellite system (GNSS) and inertial navigation system (INS) positioning performance.
MoreTranslated text
Key words
Integrated navigation,variational Bayesian,Wasserstein distance,Statistical similarity measure
AI Read Science
Must-Reading Tree
Example
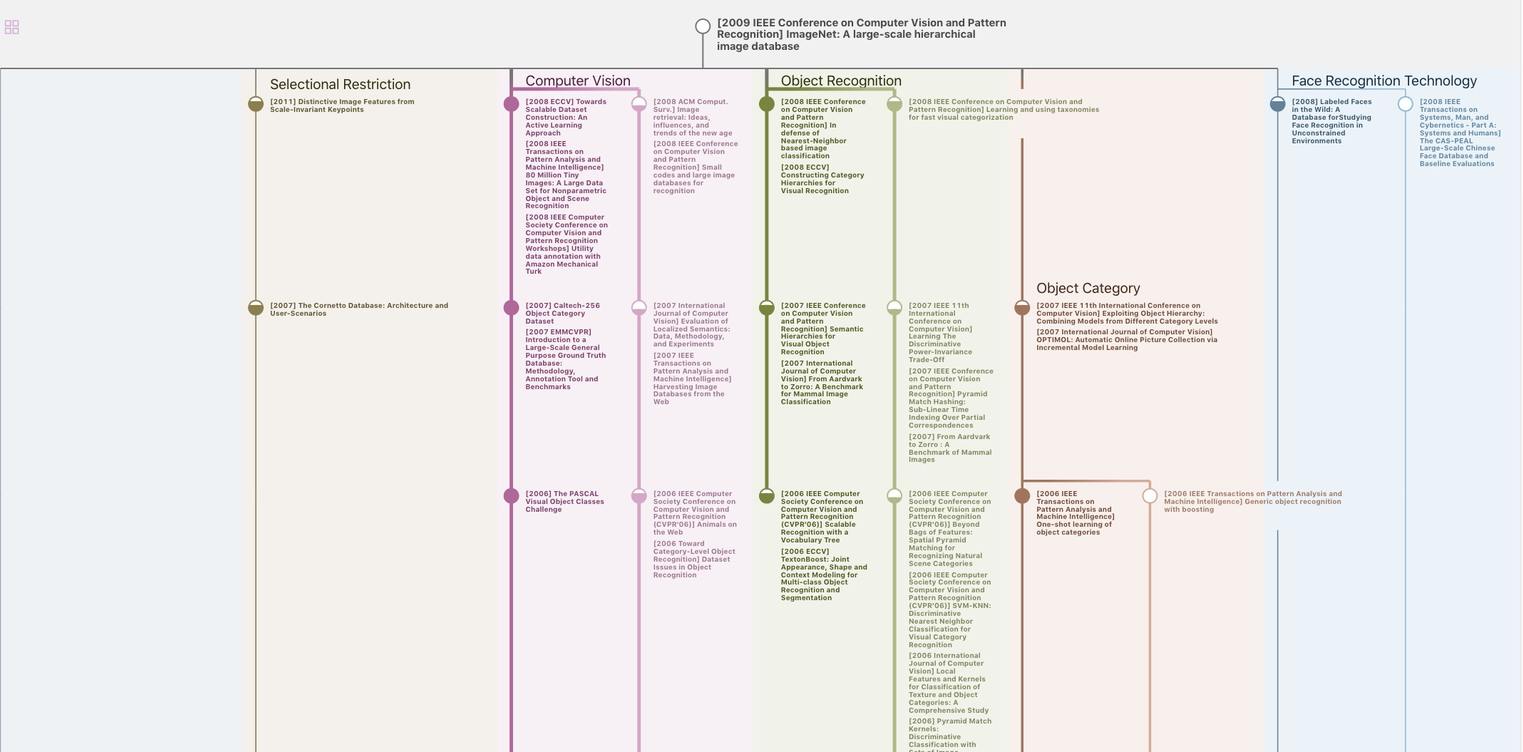
Generate MRT to find the research sequence of this paper
Chat Paper
Summary is being generated by the instructions you defined