Joint Principal Component Analysis and Supervised k Means Algorithm via Non-Iterative Analytic Optimization Approach.
IEEE Trans. Signal Process.(2024)
摘要
It is worth noting that the traditional methods for performing both the dimensional reduction and the classification are via the two steps iterative approaches. In this case, performing the dimensional reduction does not consider the classification. On the other hand, the classification is performed in the original feature domain and it does not consider the dimensional reduction. Here, the transform matrix only takes an effect on the dimensional reduction, but not on the classification. The synergy between the dimensional reduction and the classification has been ignored. As a result, the overall performance is not optimal. To address this issue, this paper proposes a joint principal component analysis (PCA) and supervised
k
means approach for performing the dimensional reduction and the classification simultaneously. In particular, both the reconstruction error due to the dimensional reduction as well as the total distance between the cluster centers and the feature vectors in the transformed domain are minimized subject to the unitary condition of the transform matrix. Here, we have two decision variables. They are the transform matrix and the cluster centers, instead of a single decision variable in each iteration in the traditional iterative method. To find the analytical solution of the optimization problem, the first order derivative condition of the optimization problem is first expressed as the matrix equations. However, there is a structure deficiency on the matrix equation. To address this issue, this paper employs the property of the singular matrices of the symmetric matrix for solving these matrix equations with the guarantee of the satisfaction of the structural deficiency. As a result, the analytical form of the solutions is derived. The proposed method is evaluated via performing the mental arithmetic classification based on the electroencephalograms (EEGs) downloaded from the PhysioNet database. The comparisons to the state of the art algorithms for performing the mental arithmetic classification and the conventional methods for finding the solutions of the constrained optimization problems are conducted. The results demonstrate that our proposed method achieves the higher accuracy and requires the lower execution time. This validates the effectiveness and the efficiency of our proposed method.
更多查看译文
关键词
Joint PCA and supervised k means algorithm,noniterative analytic optimization,singular value decomposition,symmetric matrix,mental arithmetic classification,EEG
AI 理解论文
溯源树
样例
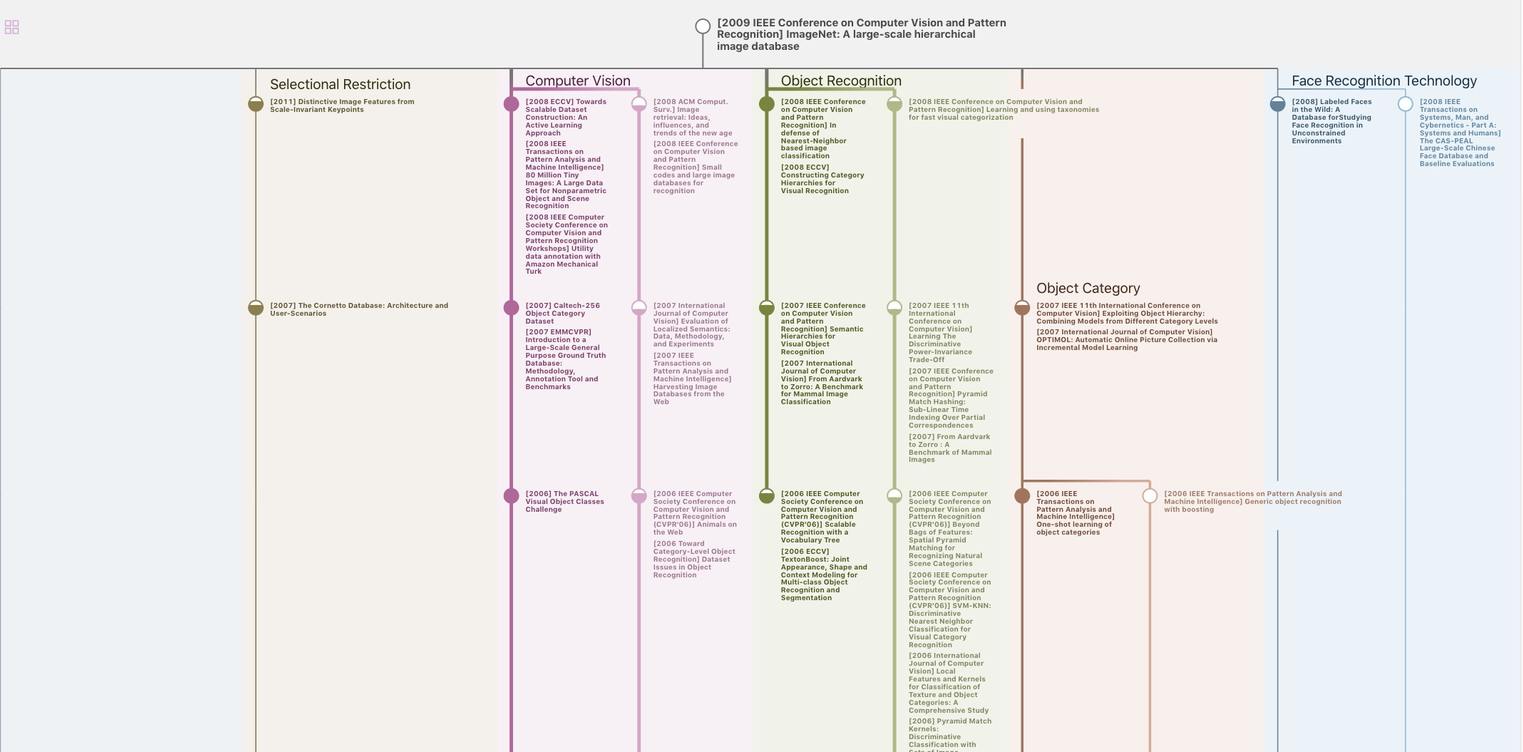
生成溯源树,研究论文发展脉络
Chat Paper
正在生成论文摘要