Decentralized Graphical-Representation-Enabled Multi-Agent Deep Reinforcement Learning for Robust Control of Cyber-Physical Systems
IEEE TRANSACTIONS ON RELIABILITY(2024)
摘要
Frequent and sizable voltage fluctuation, a common issue faced by the modern distribution system (DS), could lead to potential equipment failures and power interruption. This brings huge negative impact on the power supply reliability of the DS. Existing voltage regulation methods typically rely on the precise physical parameters, complete measurements, and perfect communication, all of these premises are difficult to meet in practice. To this end, a decentralized control method that is robust to measurement acquisition errors is developed for DS in this article. Specifically, a graph learning-based surrogate network is first built to simulate the power flow computing procedure and capture the structural characteristics of the DS. The centralized surrogate model is, then, divided into several decentralized representation networks according to the network partition results to obtain the robust embedding of the regional information of each subnetwork. Subsequently, the representation networks are embedded in the front of the actor networks of the multi-agent soft actor-critic algorithm, the agents of which are learned in a centralized fashion according to the reward value estimated by the centralized surrogate model. The systematic integration of the three components allows us to achieve cooperation between different subregions and robustness against anomalous measurements without the reliance on precise circuit parameters. Comparative studies on IEEE test system illustrate the robustness of the proposed approach.
更多查看译文
关键词
Cyber-physical system (CPS),graphical learning,multi-agent learning,power supply reliability,representation network
AI 理解论文
溯源树
样例
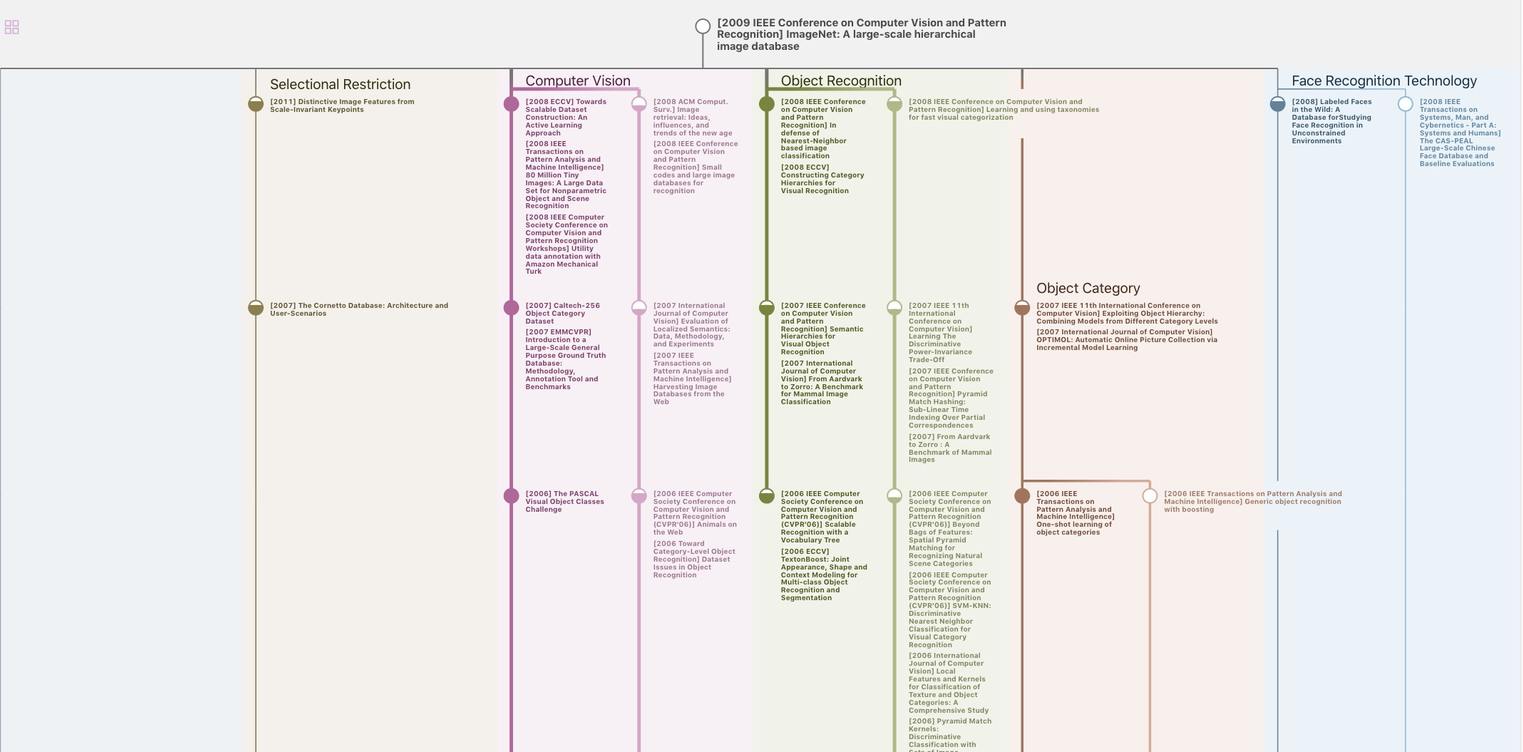
生成溯源树,研究论文发展脉络
Chat Paper
正在生成论文摘要