Evaluation of Defects Depth for Metal Sheets Using 4-coil Excitation Array Eddy Current Sensor and Improved ResNet18 Network
IEEE Sensors Journal(2024)
摘要
Lift-off effect, resulting in detection error, which is a significant challenge that needs to be addressed in defect detection using eddy current testing (ECT). A novel array eddy current sensor, with each unit composing of 4 excitation coils and a receiving coil, is proposed to implement fast and large-area defect scanning for metal sheet without lift-off interference. In addition, theoretical analysis based on Biot-Savart law is applied to illustrate the feasibility of the proposed sensor in eliminating lift-off effect. However, the proposed array sensor has also an angular sensitivity which means there are different signal responses for different defect orientations, but the feature is not conducive to evaluate the defect depths without knowing the angle difference as a prior, which can be addressed by deep learning (DL) algorithm. Both simulation and experiment are implemented to verify the reliability and feasibility of proposed array sensor. The proposed preprocessed method is utilized to attain significant signal feature differences for various defect depths. In addition, the improved ResNet18 network is proposed to train acquired experimental data, and its superior performance is demonstrated. The results show that the improved ResNet18 network with preprocessed data achieves fastest training speed compared to other methods in this paper. At same time, the proposed DL network achieves high accuracy of 98.1% and 95.4% for defects depth for aluminum sheet and stainless-steel sheet, respectively. Additionally, when considering 16 labels combinations of lift-off distances and angle differences during the process of inspection, the accuracy of lift-off classification for two materials are 97.2% and 96.9%, respectively.
更多查看译文
关键词
Eddy current testing (ECT),Lift-off,Array sensor,ResNet18,Deeping learning
AI 理解论文
溯源树
样例
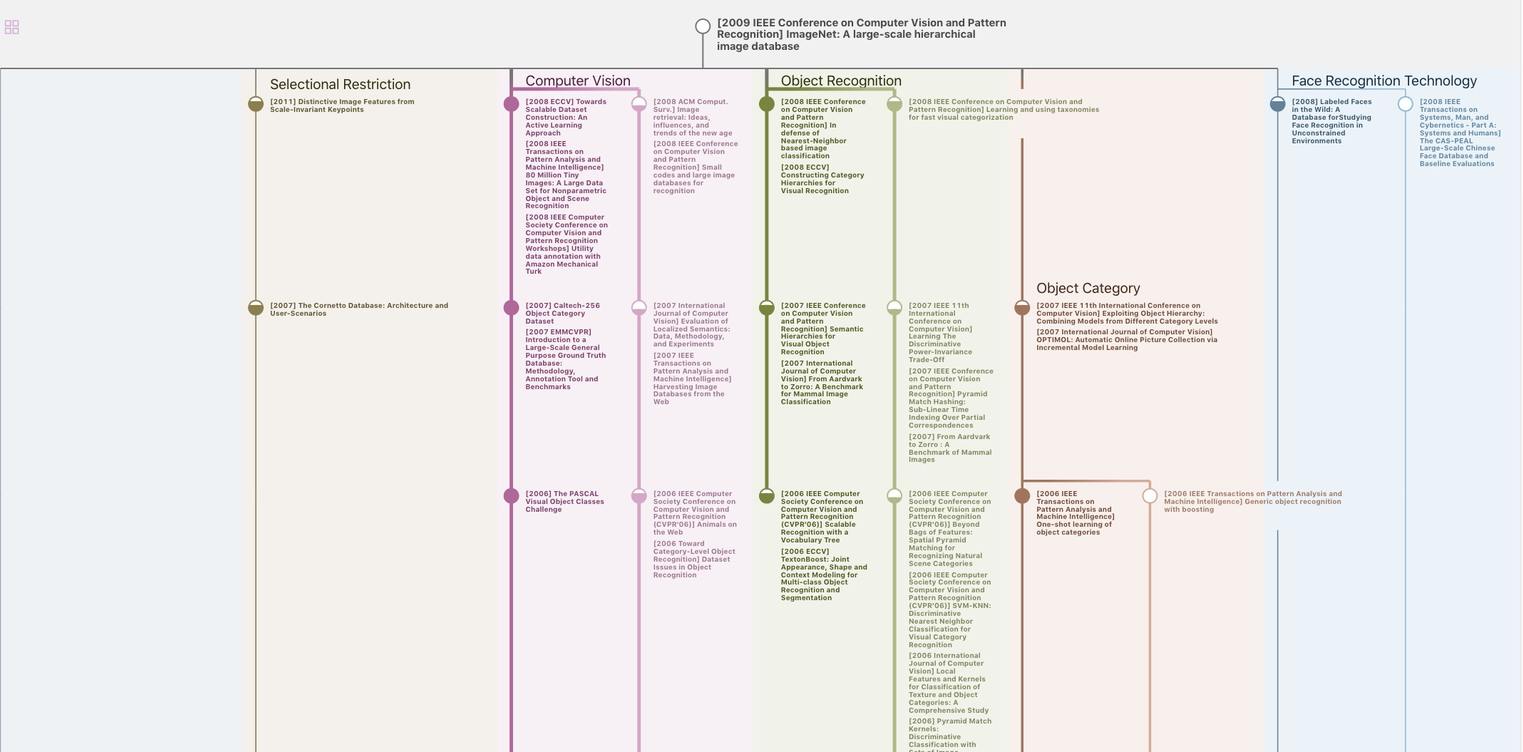
生成溯源树,研究论文发展脉络
Chat Paper
正在生成论文摘要