Observer-Based Adaptive Finite-Time Neural Control for Constrained Nonlinear Systems With Actuator Saturation Compensation.
IEEE Trans. Instrum. Meas.(2024)
摘要
This brief designs an observer-based adaptive finite-time neural control for a class of constrained nonlinear systems with external disturbances, and actuator saturation. First, a neural network (NN) state observer is developed to estimate the unmeasurable states. Combining the improved Gaussian function and an auxiliary compensation system, the actuator saturation can be solved. The ”
explosion of complexity
” problem is tackled by the finite-time command filter, and the filtering-error compensation system is constructed to resolve the filtering error. Moreover, the barrier Lyapunov function is incorporated into the controller design to satisfy the state constraints. By integrating the NN technique and the virtual parameter learning to approximate the bound of the lumped disturbance, the number of learning parameters is decreased. It can be proved that all the states do not transgress the predefined bounds and the tracking errors converge to bounded regions in finite time. Eventually, we provide comparative results to show the feasibility of the obtained results.
更多查看译文
关键词
Actuator saturation,full-state constraints,finite-time control,neural networks,state observer
AI 理解论文
溯源树
样例
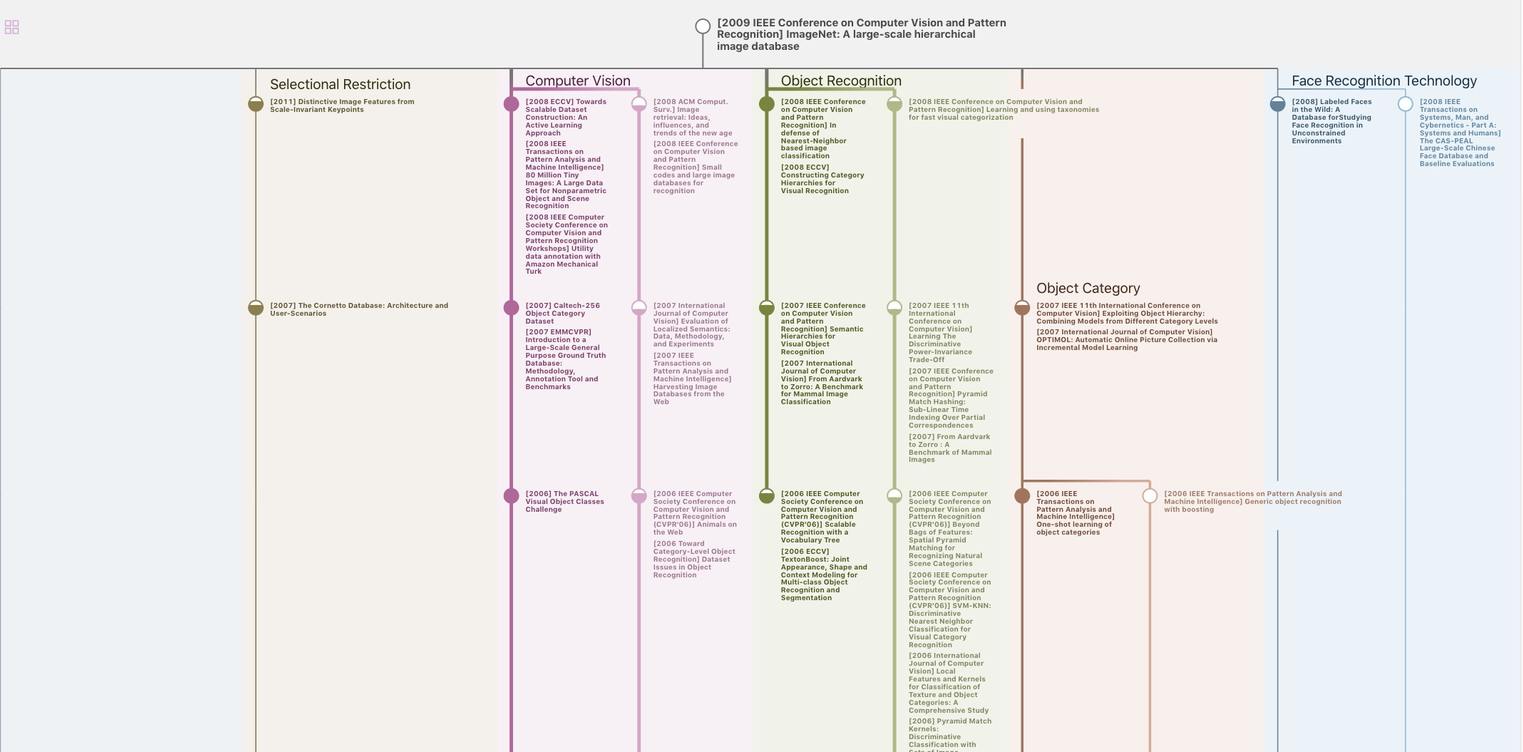
生成溯源树,研究论文发展脉络
Chat Paper
正在生成论文摘要