Spatial and Temporal Detection With Attention for Real-Time Video Analytics At Edges
IEEE Transactions on Mobile Computing(2024)
摘要
The detection of objects via neural networks plays a key role in various video analytics, but consumes huge resources. Due to the limited computing capability at edges, such real-time detections should be precisely used for the objects that need the most attention. Unfortunately, as the target objects keep moving, existing systems fail to consider both the distribution of objects and object movements over regions, and existing tracking mechanisms are easily affected by background content. Therefore, we propose spatial and temporal detection with attention for analytics, to increase the quality of detections for those targets. However, the attention shift over regions, the uncertainty of detections, and the constrained edge resources essentially hamper us from efficient analytics. We propose an adaptive partition planner to divide the frame into regions to achieve spatial attention. Afterwards, we design a detection planner to orchestrate the detection model temporally for each region by an online mechanism, via a queue-based adaptation. The spatial and temporal attention are integrated to maximize the accumulative detection accuracy. Via rigorous proof, both dynamic regret regarding detection accuracy and the real-time requirement for the video analytics are ensured. The testbed experiments confirm the superiority of our approach over multiple state-of-the-art algorithms.
更多查看译文
关键词
Image partition,object detection,online learning,real-time video analytics,resource provisioning
AI 理解论文
溯源树
样例
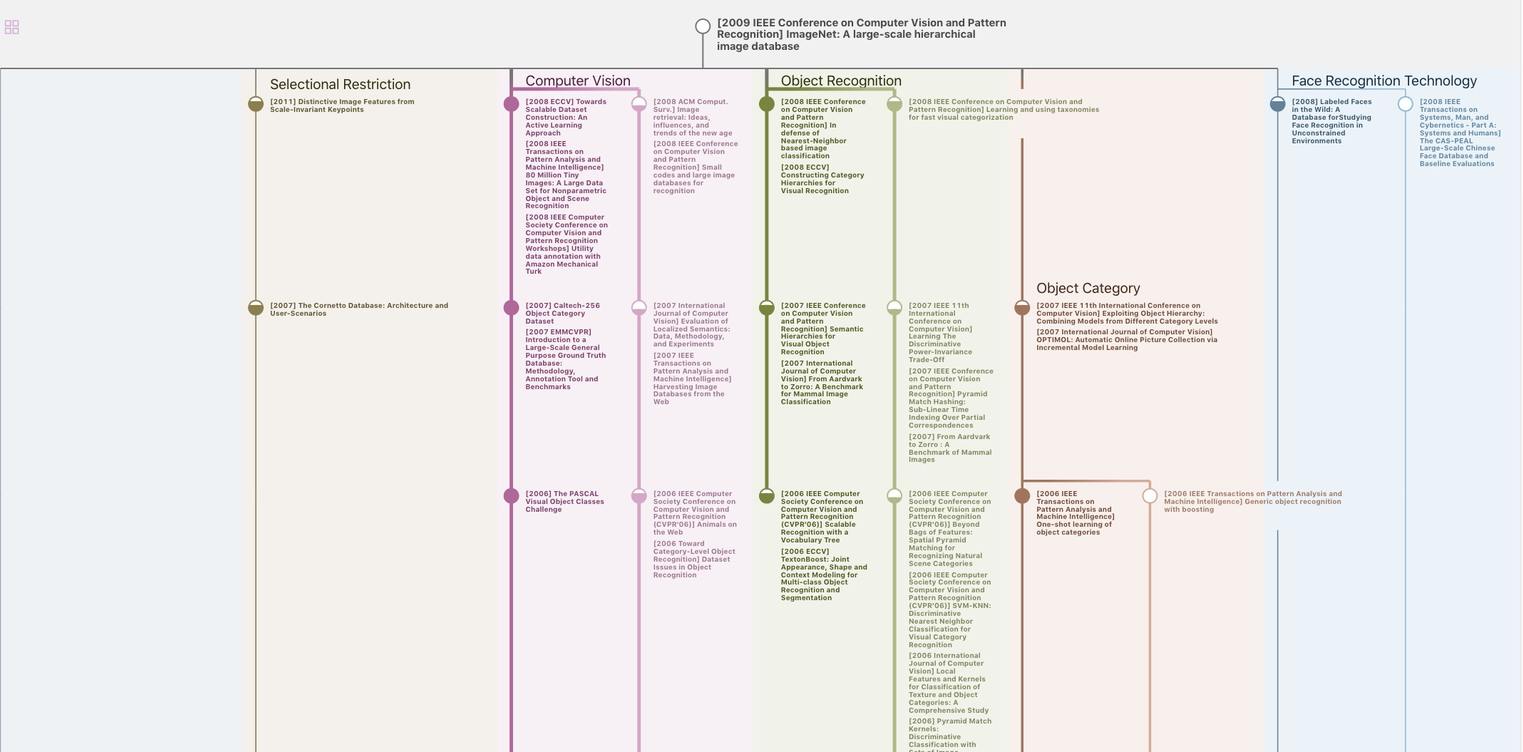
生成溯源树,研究论文发展脉络
Chat Paper
正在生成论文摘要