FedUC: A Unified Clustering Approach for Hierarchical Federated Learning
IEEE Transactions on Mobile Computing(2024)
Abstract
Federated learning (FL) is an effective approach to train models collaboratively among distributed edge nodes (i.e., workers) while facing three crucial challenges, edge heterogeneity, resource constraint, and Non-IID data. Under the parameter server (PS) architecture, a single parameter server may become the system bottleneck and cannot well deal with the edge heterogeneity, while the peer-to-peer (P2P) architecture causes significant communication consumption to achieve satisfactory training performance. To this end, hierarchical aggregation (HA) architecture is proposed to cluster workers to tackle the edge heterogeneity and reduce communication consumption for FL. However, the existing researches on HA architecture cannot provide a unified clustering approach for various inter-cluster aggregation patterns (e.g., centralized or decentralized structure, synchronous or asynchronous mode). In this paper, we explore the quantitative relationship between the convergence bounds of different inter-cluster patterns and several factors, e.g., data distribution, frequency of clusters participating in inter-cluster aggregation (for asynchronous modes), and inter-cluster topology (for decentralized structures). Based on the convergence bounds, we design a unified clustering algorithm FedUC to organize workers for different patterns. Experimental results on classical models and datasets show that FedUC can greatly accelerate the model training of different patterns by 1.79-7.39× compared with the state-of-the-art clustering methods.
MoreTranslated text
Key words
Federated learning,Edge computing,Clustering optimization,Heterogeneity,Resource constraint,Non-IID
AI Read Science
Must-Reading Tree
Example
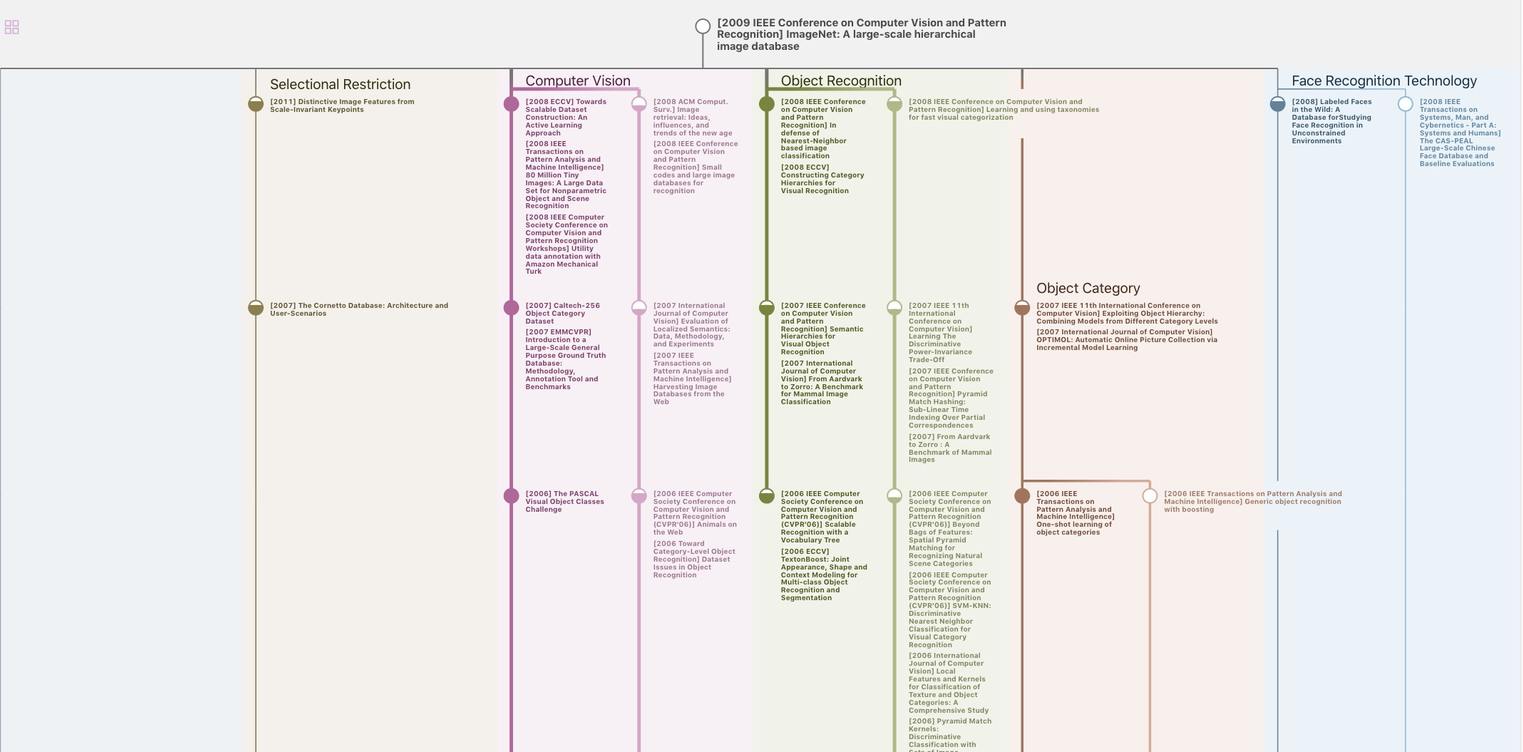
Generate MRT to find the research sequence of this paper
Chat Paper
Summary is being generated by the instructions you defined