Unbiased Visual Question Answering by Leveraging Instrumental Variable.
IEEE Trans. Multim.(2024)
Abstract
Existing unbiased VQA models reduce the spurious correlation between questions and answers to force the models to focus on visual information. However, the visual information captured by these unbiased models is irrelevant to the correct answer, resulting in leveraging spurious correlation to predict incorrect answers. This makes these unbiased methods fail to obtain critical visual information, thus performing poorly on questions dominated by the visual information. To capture the valuable visual information, this paper proposes a novel unbiased VQA model based on causal inference, leveraging Instrumental Variable (IVar) to increase the causal effect between visual features and answers. First, to obtain suitable instrumental variables, the noise generator is proposed according to the constraints of IVar. The generated noise can be regarded as IVar, which is used to pollute the original visual features. Then, this paper proposes IVar loss which utilizes the generated IVar to increase the causal effect between visual features and answers. When the visual feature is polluted by IVar, IVar loss guides the model to predict incorrect answers to enhance the correlation between IVar and the answer. Since the correlation between IVar and the answer is proportional to the causal effect between the visual feature and the answer, IVar loss enhances the importance of the visual information, thereby rectifying the model to capture critical visual information. The extensive experimental results on widely-used benchmarks demonstrate the advantages of the proposed method. The proposed method gains the best accuracy on answer type
Other
of VQA-CP v2. These results demonstrate the superiority of the proposed method in capturing critical visual information since most questions on the answer type
Other
are dominated by visual information.
MoreTranslated text
Key words
visual question answering,instrumental variable,causal inference,out of distribution
AI Read Science
Must-Reading Tree
Example
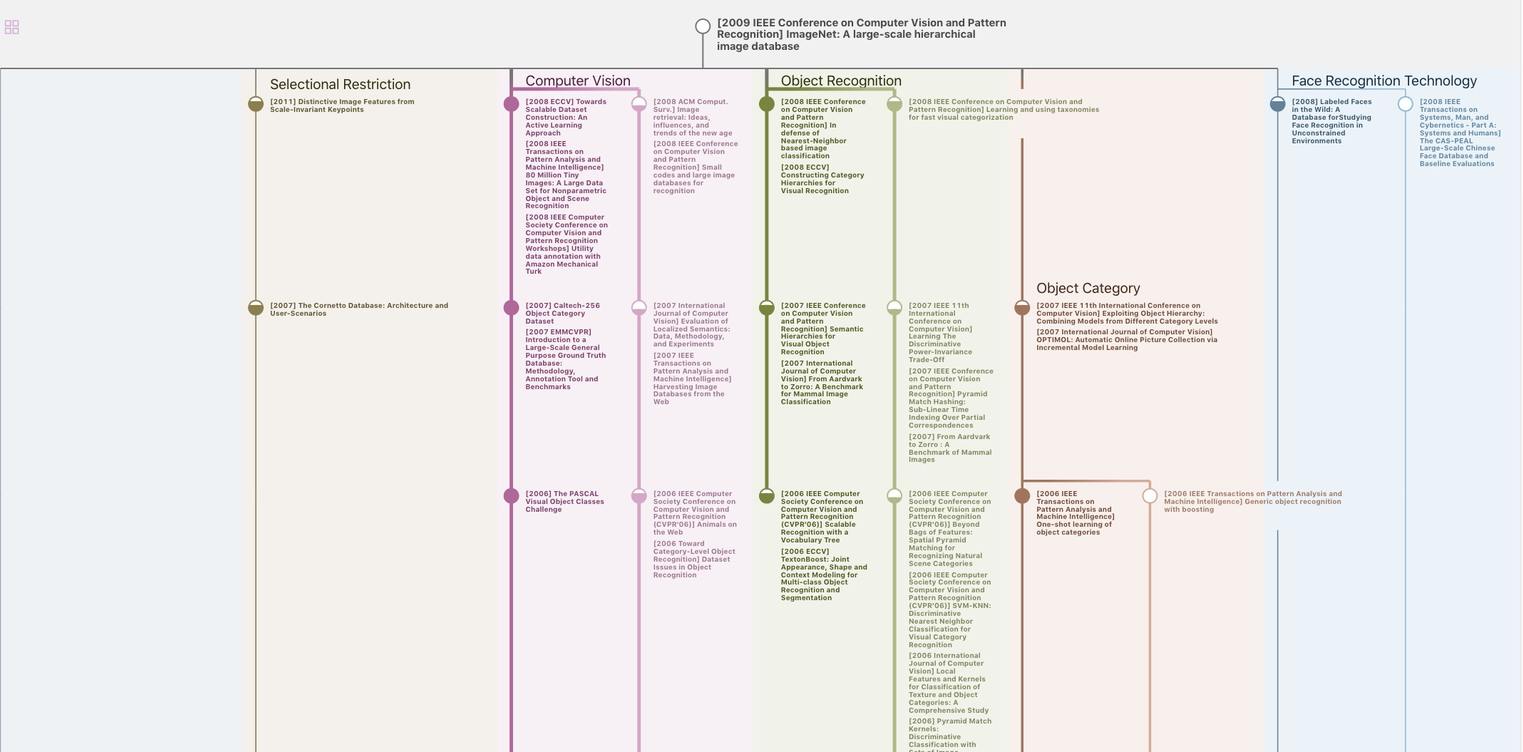
Generate MRT to find the research sequence of this paper
Chat Paper
Summary is being generated by the instructions you defined