A New Horizon: Employing Map Clustering Similarity for LiDAR-based Place Recognition
IEEE Transactions on Intelligent Vehicles(2024)
Abstract
Lidar-based Place Recognition (LPR) is crucial for intelligent vehicle navigation. Existing methods generally create LiDAR descriptors for pairwise comparisons or employ prior maps for metric localization but face challenges in computational complexity, limited robustness, and excessive memory overhead. Thus, this paper offers a fresh perspective called Map Clustering Similarity (MCS), improving robustness while reducing memory and remarkably boosting efficiency. We start by treating the ground as potential vehicle locations, i.e., virtual points, and introduce a compact LiDAR descriptor called Occupancy Scan Context (OcSC) to capture environmental occupancy from a bird's-eye view. We then employ the point cloud map, virtual points, and k-means clustering to condense the map data into 4Kb cluster centers. Eventually, we devise a two-phase online search algorithm. In the first phase, we extract the OcSC's ring key from online single-frame data, gauge its resemblance to map cluster centers to derive a cluster descriptor, and search loop candidates using the Spearman loss. In the second phase, we propose an occupancy loss to compare all candidates' OcSC descriptors to find the optimal candidate. Our method introduces a novel framework and merges advantages from existing solutions. Experiments on the KITTI dataset and two self-collected indoor sequences showcase MCS-BF's superior performance over mainstream methods in place recognition recall,
F1
score, and memory consumption. Additionally, MCS successfully balances runtime with accuracy. The source code will be available in
https://github.com/ShiPC-AI/MCS.
MoreTranslated text
Key words
LiDAR-based place recognition,occupation scan context,cluster descriptor,ground segmentation,point cloud map
AI Read Science
Must-Reading Tree
Example
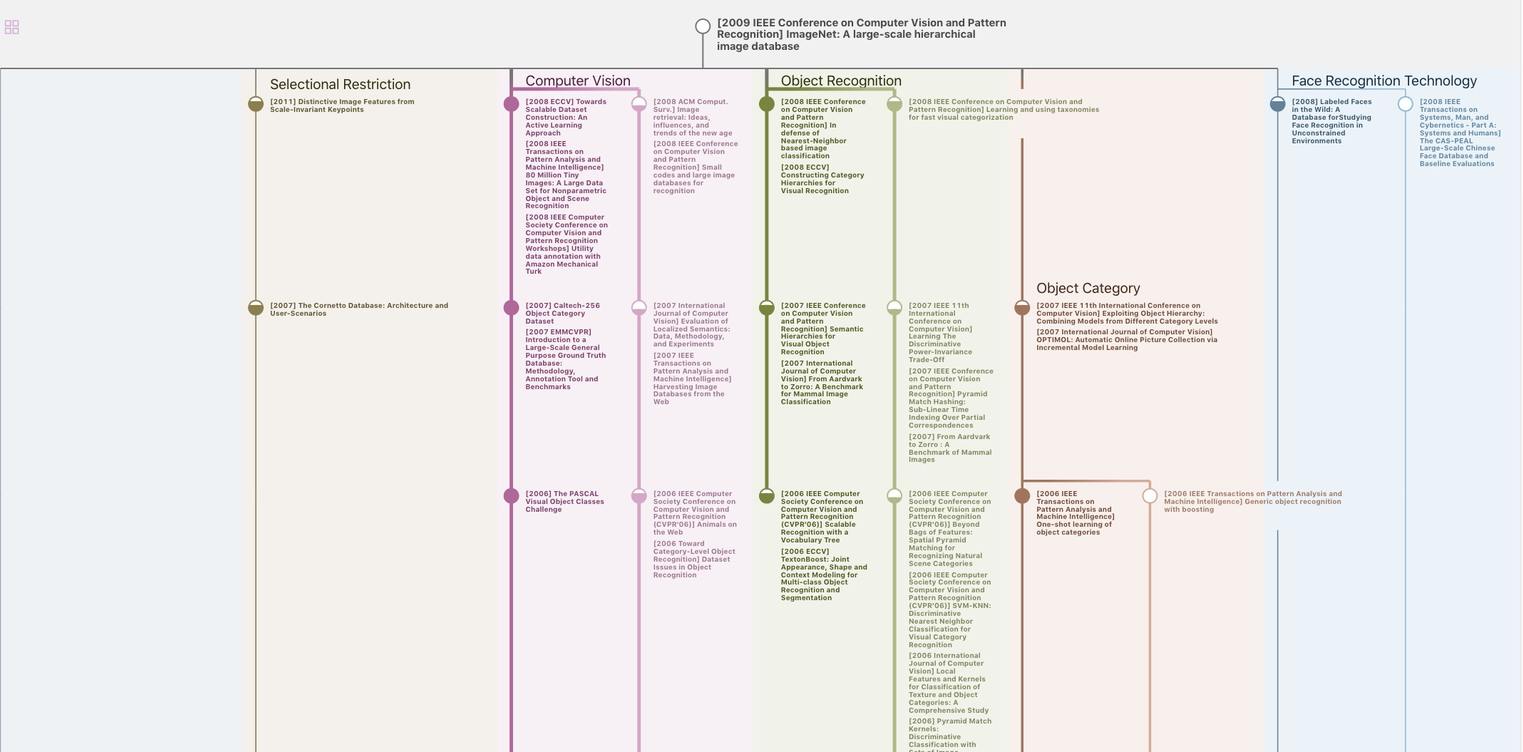
Generate MRT to find the research sequence of this paper
Chat Paper
Summary is being generated by the instructions you defined