Online Video Sparse Noise Removing via Nonlocal Robust PCA.
IEEE Trans. Multim.(2024)
摘要
Online schemes and nonlocal similarity are two effective approaches for strengthening robust principal component analysis (RPCA) techniques in video denoising. However, their limitations are also evident. The online scheme is usually highly efficient but lacks consideration of regional appearance information, thus it cannot effectively handle videos with complex dynamics such as object movements. On the other hand, nonlocal similarity is used to better utilize regional information but incurs a heavy computational cost. Moreover, these two techniques are incompatible and challenging to work together. To overcome this barrier and harness the advantages of both approaches, this paper proposes a novel online nonlocal RPCA method. 1) A clustering based nonlocal strategy (ClusNonlocal) is adopted, which not only greatly reduces the computation cost, but also forms low-dimensional subspaces for online processing; 2) a new weighted RPCA model is proposed, which regards samples with different importances and improves the performance of subspace pursuit and video recovery; 3) a multi-level subspace updating scheme and weighted projection method is proposed, which keeps the performance of online video data processing at a high level at all time. A series of video denoising experiments are carried out to demonstrate the overall advantages of our procedure over several other ones, in terms of both visual quality and running speed.
更多查看译文
关键词
Robust PCA,Online scheme,Nonlocal similarity,Video denoising
AI 理解论文
溯源树
样例
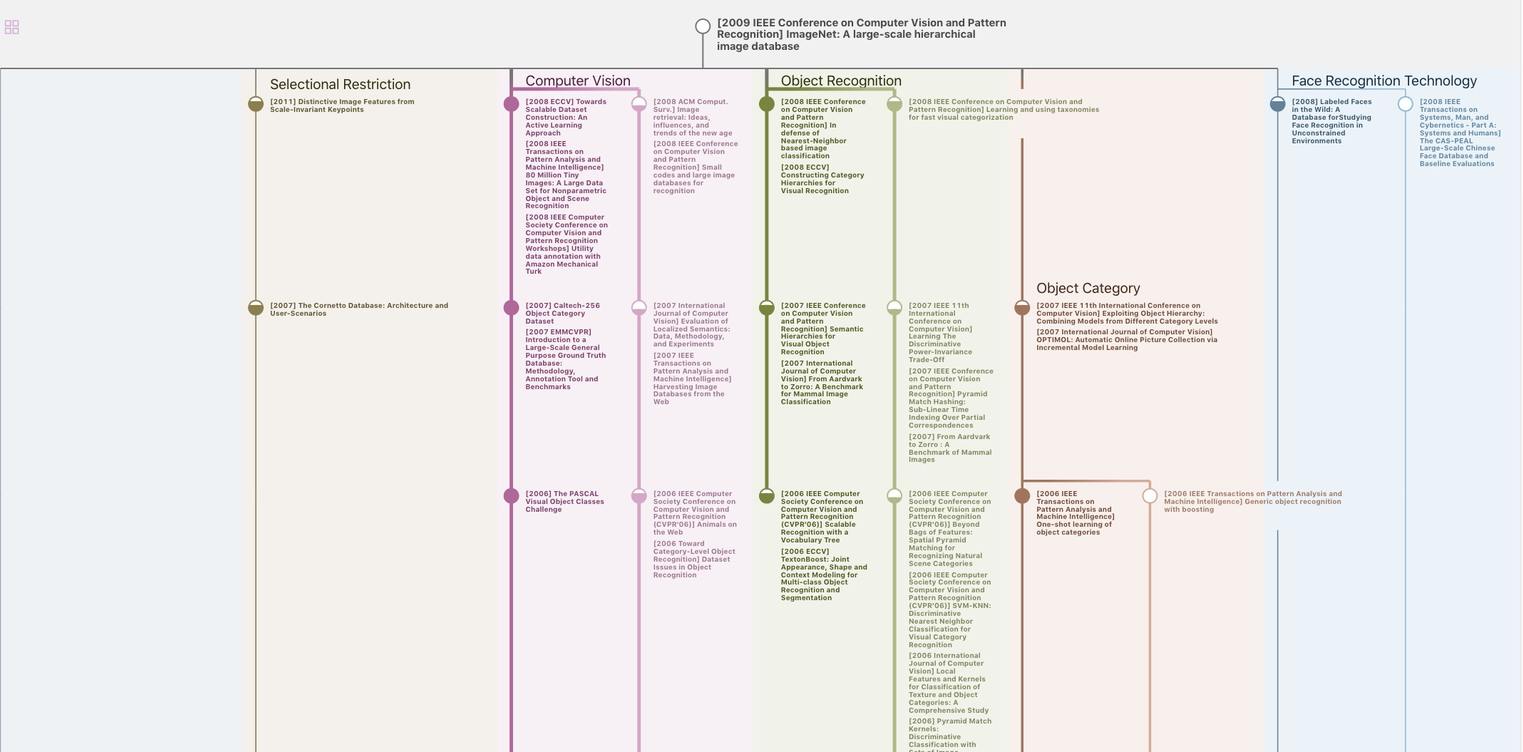
生成溯源树,研究论文发展脉络
Chat Paper
正在生成论文摘要