Video Offloading in Mobile Edge Computing: Dealing with Uncertainty
IEEE Transactions on Mobile Computing(2024)
摘要
Videos are projected to account for roughly 80% of global mobile data traffic by 2028. Many camera-equipped mobile devices, such as surveillance drones, require realtime video analytics, encompassing tasks like object detection and action recognition. These devices, restricted by limited resource constraints, need to offload videos to Mobile Edge Computing (MEC) to simultaneously optimize video analytics performance and minimize delay. However, MEC is facing significant challenges in providing efficient video offloading solutions, especially due to uncertainties caused by dynamic device mobility and the associated trade offs in selecting video quality for offloading. Offloading high-quality videos enhances video analytics performance, such as object detection accuracy. Yet, as a mobile device relocates, lower video quality or serving by a different MEC cloudlet may be required (triggering a service migration) to maintain a satisfactory service performance. In this paper, we study the Video Offloading Problem (VOP) in MEC to address these challenges. We propose two uncertainty-aware approaches that model the uncertainties in the environment to solve VOP. Our first approach, focusing on system side, is based on Two-stage Stochastic Program and proposing a unique clustering-based Sample Average Approximation to effectively solve TSP-VOP. The second approach, focusing on device side, employs an online learning algorithm based on a multi-armed bandit to learn and select the optimal offloading solution online. Through extensive experiments, we show that our proposed approaches significantly enhance video offloading decisions, with high video quality and reduced service migration costs under uncertain device mobility, compared to other benchmarks.
更多查看译文
关键词
Mobile Edge Computing,Video Offloading,Mobility,Video Quality,Stochastic Program,Sample Average Approximation,Clustering,Multi-Armed Bandit,Online Learning
AI 理解论文
溯源树
样例
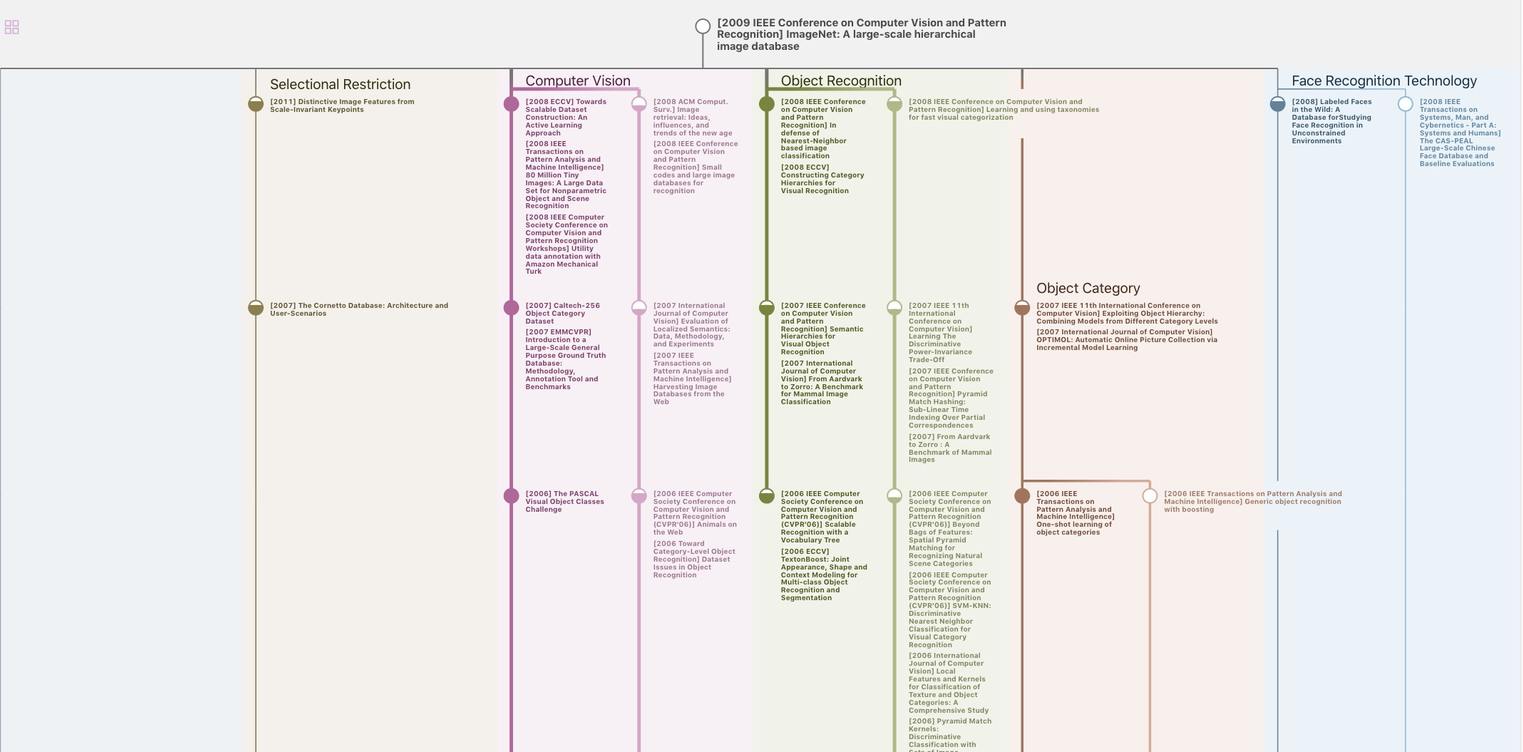
生成溯源树,研究论文发展脉络
Chat Paper
正在生成论文摘要