Anchor Graph-Based Feature Selection for One-Step Multi-View Clustering
IEEE Transactions on Multimedia(2024)
摘要
Recently, multi-view clustering methods have been widely used in handling multi-media data and have achieved impressive performances. Among the many multi-view clustering methods, anchor graph-based multi-view clustering has been proven to be highly efficient for large-scale data processing. However, most existing anchor graph-based clustering methods necessitate post-processing to obtain clustering labels and are unable to effectively utilize the information within anchor graphs. To address this issue, we draw inspiration from regression and feature selection to propose
A
nchor
G
raph-Based
F
eature
S
election for
O
ne-step
M
ulti-
V
iew
C
lustering (AGFS-OMVC). Our method combines embedding learning and sparse constraint to perform feature selection, allowing us to remove noisy anchor points and redundant connections in the anchor graph. This results in a clean anchor graph that can be projected into the label space, enabling us to obtain clustering labels in a single step without post-processing. Lastly, we employ the tensor Schatten
$p$
-norm as a tensor rank approximation function to capture the complementary information between different views, ensuring similarity between cluster assignment matrices. Experimental results on five real-world datasets demonstrate that our proposed method outperforms state-of-the-art approaches.
更多查看译文
关键词
Multi-view clustering,feature selection,sparse representation
AI 理解论文
溯源树
样例
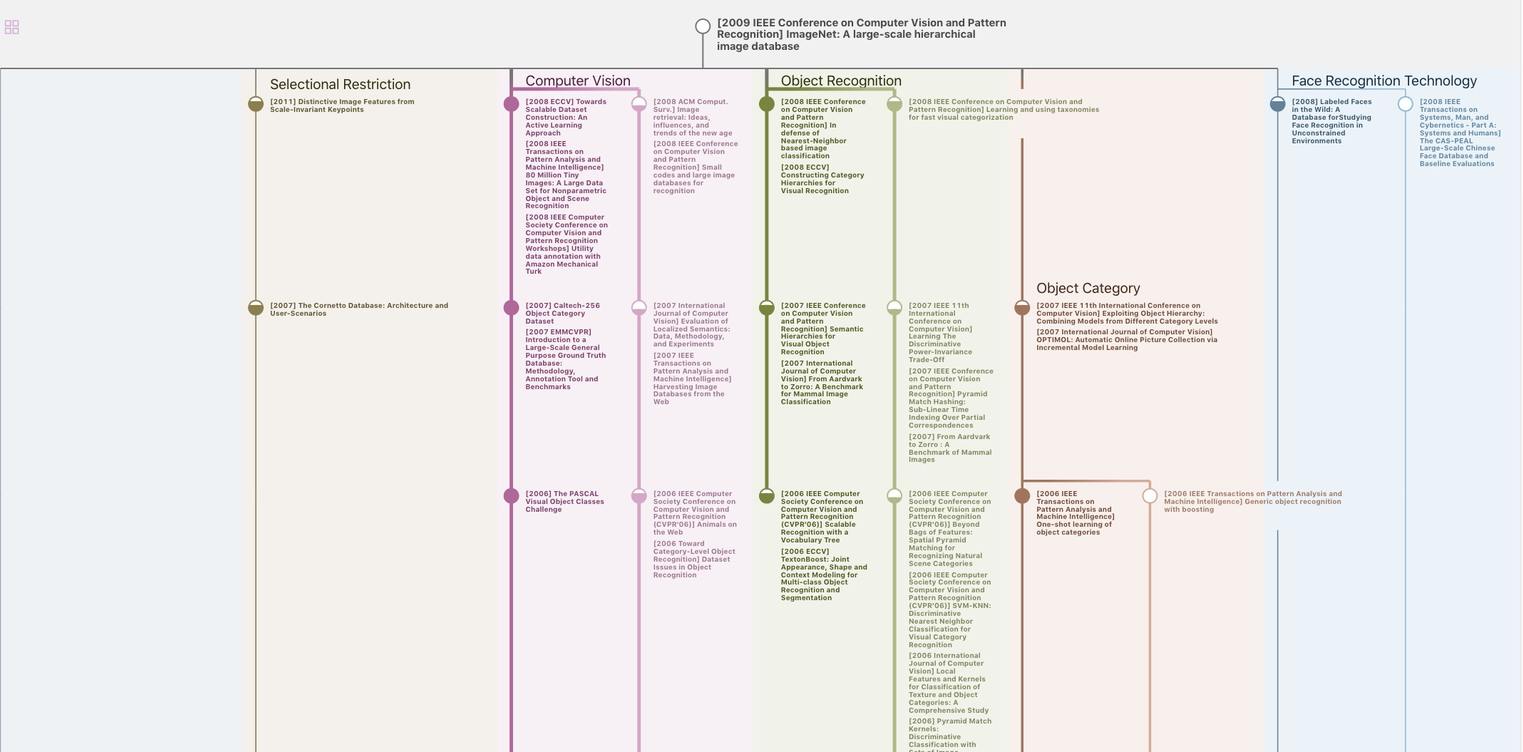
生成溯源树,研究论文发展脉络
Chat Paper
正在生成论文摘要