Robust Multi-Kernel Nearest Neighborhood for Outlier Detection
IEEE Transactions on Knowledge and Data Engineering(2024)
摘要
Outlier detection methods based on distance measure have been used in numerous applications due to their effectiveness and interpretability. However, distances among instances heavily depend on the feature space in which they reside. For an outlier, distances from it to the normal instances may be extremely close in one feature space, failing to separate them from each other, while this situation is reversed in another space. Meanwhile, the distance measure is sensitive to a few “marginal instances” (i.e., normal instances located very close to outliers in the feature space) during the estimation of whether a test instance is an outlier or not. In this paper, we propose a robust multi-kernel nearest neighborhood (RMKN) method for outlier detection. Specifically, in the training phase, we only consider normal instances and transform them into a Polynomial kernel function weighted digraph to capture their geometric relationships in the original feature space. Then, we develop an objective function based on the weighted digraph to find a latent feature space via multi-kernel learning such that distances among normal instances in this latent feature space are as close as possible while preserving their original distributions. In the detecting phase, we design an outlying score based on the two-stage multi-kernel k-nearest nearest neighbors to detect outliers. Extensive experiments with ten datasets show that RMKN is effective and robust
更多查看译文
关键词
Outlier detection,multi-kernel learning,weighted digraph,nearest neighborhood
AI 理解论文
溯源树
样例
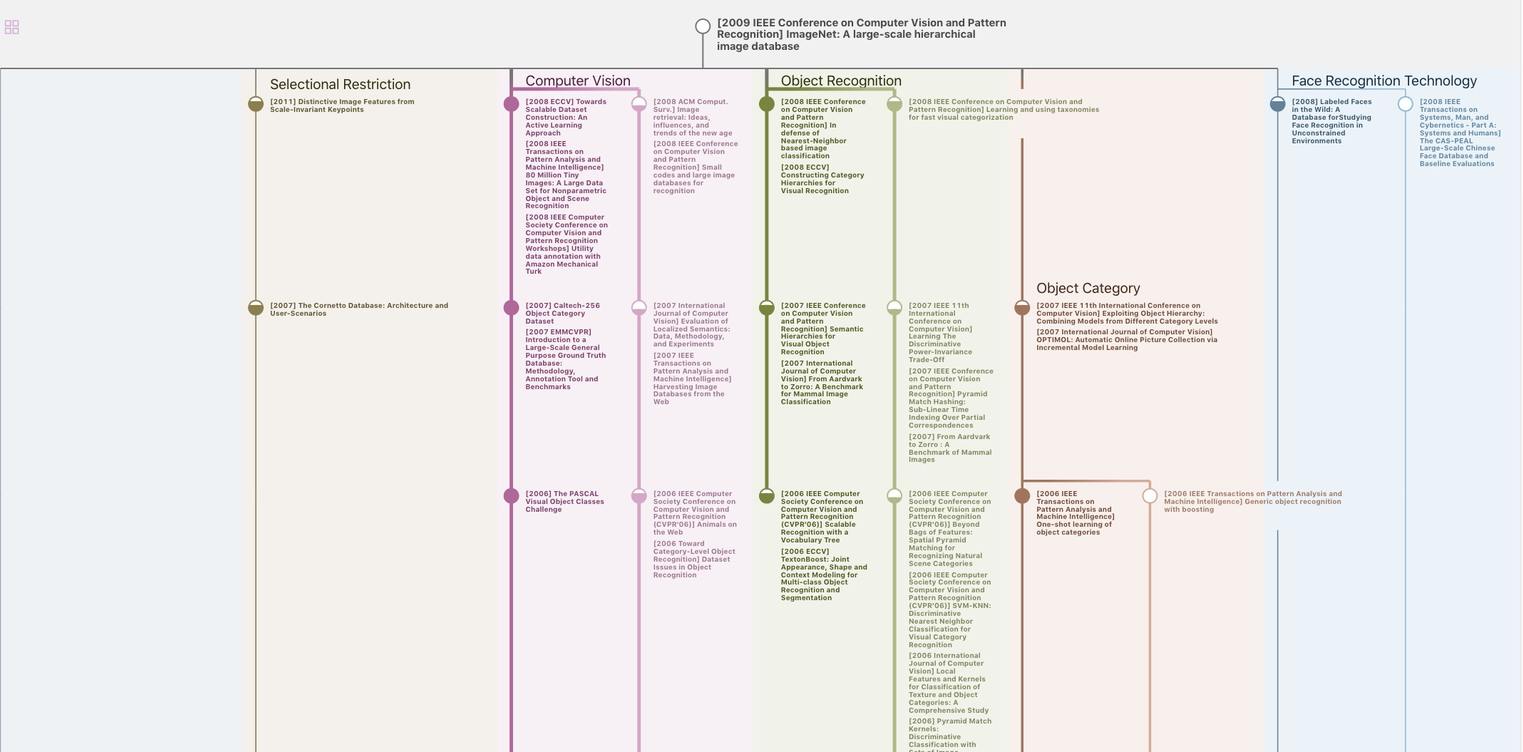
生成溯源树,研究论文发展脉络
Chat Paper
正在生成论文摘要