Wasserstein Embedding Learning for Deep Clustering: A Generative Approach.
IEEE Trans. Multim.(2024)
摘要
Deep learning-based clustering methods, especially those incorporating deep generative models, have recently shown noticeable improvement on many multimedia benchmark datasets. However, existing generative models still suffer from unstable training, and the gradient vanishes, which results in the inability to learn desirable embedded features for clustering. In this paper, we aim to tackle this problem by exploring the capability of Wasserstein embedding in learning representative embedded features and introducing a new clustering module for jointly optimizing embedding learning and clustering. To this end, we propose Wasserstein embedding clustering (WEC), which integrates robust generative models with clustering. By directly minimizing the discrepancy between the prior and marginal distribution, we transform the optimization problem of Wasserstein distance from the original data space into embedding space, which differs from other generative approaches that optimize in the original data space. Consequently, it naturally allows us to construct a joint optimization framework with the designed clustering module in the embedding layer. Due to the substitutability of the penalty term in Wasserstein embedding, we further propose two types of deep clustering models by selecting different penalty terms. Comparative experiments conducted on nine publicly available multimedia datasets with several state-of-the-art methods demonstrate the effectiveness of our method.
更多查看译文
关键词
Unsupervised learning,clustering analysis,Wasserstein embedding,generative models,auto-encoder
AI 理解论文
溯源树
样例
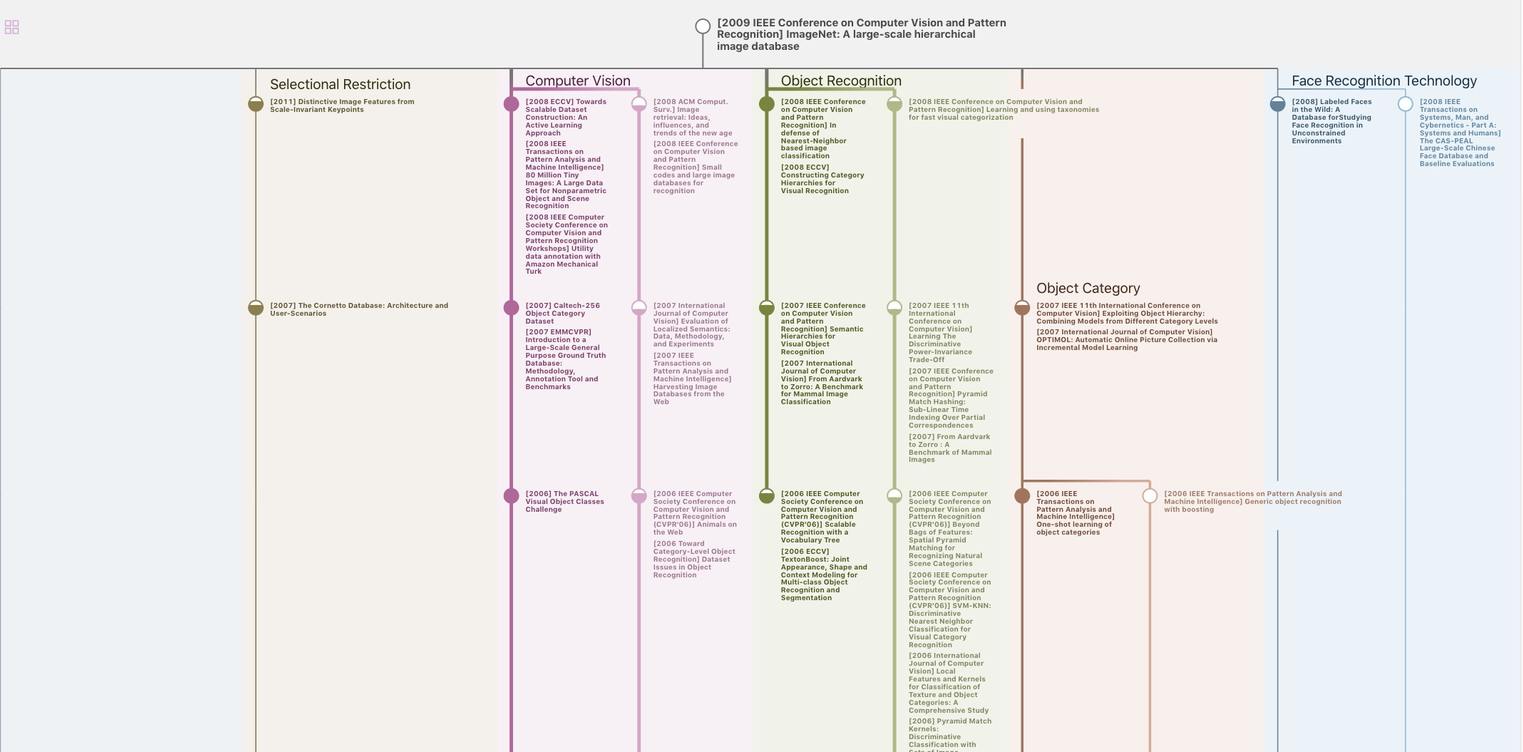
生成溯源树,研究论文发展脉络
Chat Paper
正在生成论文摘要