Transferring Meta-Policy From Simulation to Reality via Progressive Neural Network
IEEE ROBOTICS AND AUTOMATION LETTERS(2024)
摘要
Deep reinforcement learning has achieved great success in many challenging domains. However, sample efficiency and safety issues still prevent from applying deep reinforcement learning directly in robotics. Sim-to-real transfer learning is one feasible solution to tackle these problems and address the reality gap between simulation and reality. In this letter, we propose to combine meta-reinforcement learning and progressive neural network (PNN) by meta-training a policy for multiple source tasks and transferring it to the real-world robot via PNN (MetaPNN). We expect that training meta-policy over meta-tasks without considering dynamics discrepancy with our method can bridge the gap between simulation and reality with mismatched dynamics, and allow the agent to learn one single policy solving multiple tasks instead of using one policy network in PNN to solve one task. Meanwhile, the transferred meta-policy via PNN is expected to solve the target task and adapt to new situations at the same time. Our results in a variety of target tasks in AntPos and Reach with simulated manipulator show that MetaPNN can significantly improve the robot's learning efficiency and performance. Our further results in real-world Reach tasks with physical robot arm and a new task that is different from the meta-tasks show there might be a synergy between meta-learning and PNN.
更多查看译文
关键词
Task analysis,Robots,Adaptation models,Training,Neural networks,Feature extraction,Data models,Continual learning,meta-reinforcement learning,progressive neural network,sim2real,transfer learning
AI 理解论文
溯源树
样例
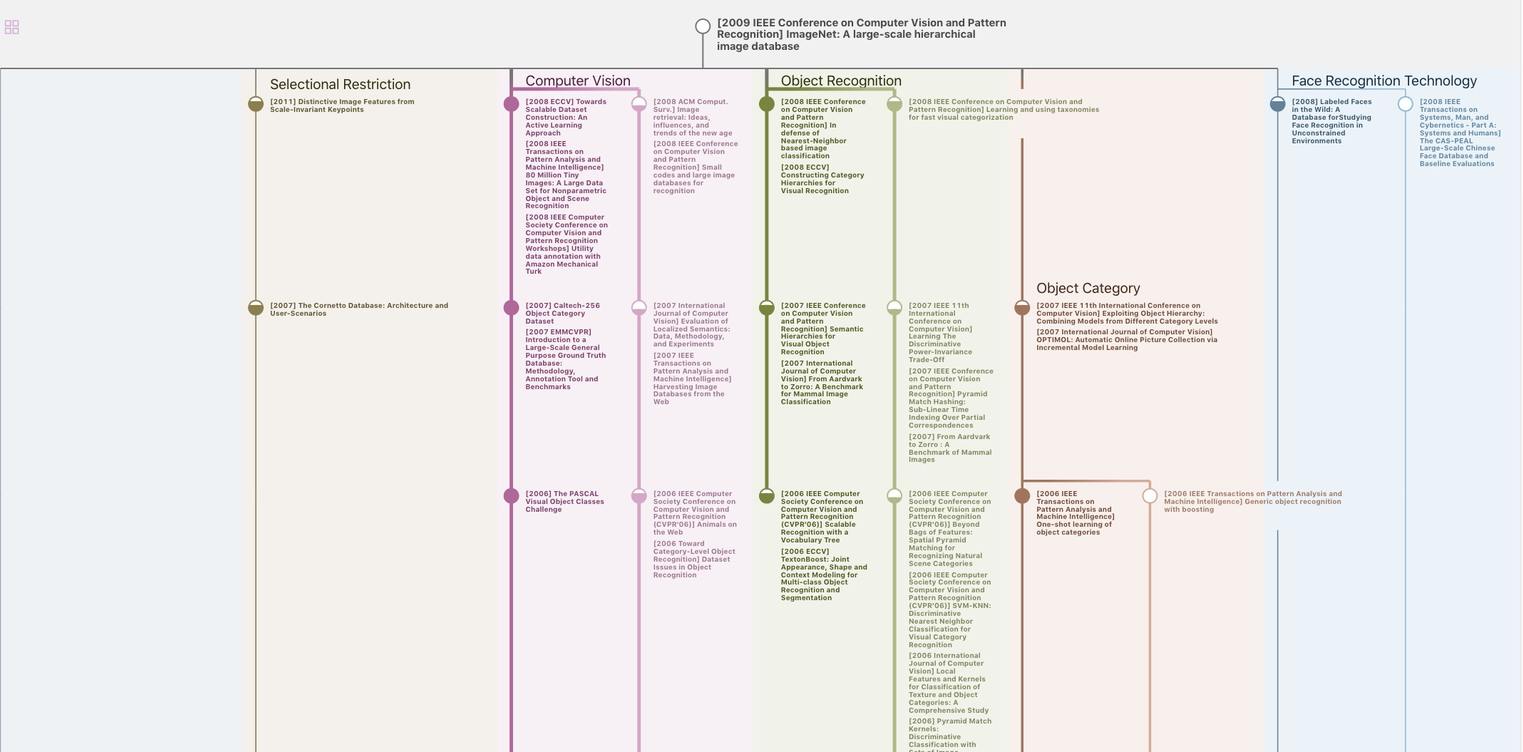
生成溯源树,研究论文发展脉络
Chat Paper
正在生成论文摘要