Learn to Defend: Adversarial Multi-Distillation for Automatic Modulation Recognition Models.
IEEE Trans. Inf. Forensics Secur.(2024)
摘要
Automatic modulation recognition (AMR) of radio signal is an important research topic in the area of non-cooperative communication and cognitive radio. Recently deep learning (DL) techniques enable significant progress in AMR. However, the techniques of adversarial machine learning cause the threats of adversarial attacks in DL-based AMR. In this paper, we aim to make AMR model robust, accurate and lightweight, thus propose a multi-distillation mechanism for robust training of DL-based AMR models, namely Adversarial Multi-Distillation (AMD). In the framework of AMD, by knowledge distillation, two powerful teacher models transfer the learned classification knowledge and defense knowledge, respectively, to the student model to form robust training. Our experiments with public dataset RML2016.10a show that the proposed method can significantly improve the defense of AMR models to against adversarial perturbations and keep relatively high classification accuracy, which enables robust decision making with lightweight models under adversarial attacks.
更多查看译文
关键词
Modulation Recognition,Adversarial Attacks,Adversarial Training,Knowledge Distillation
AI 理解论文
溯源树
样例
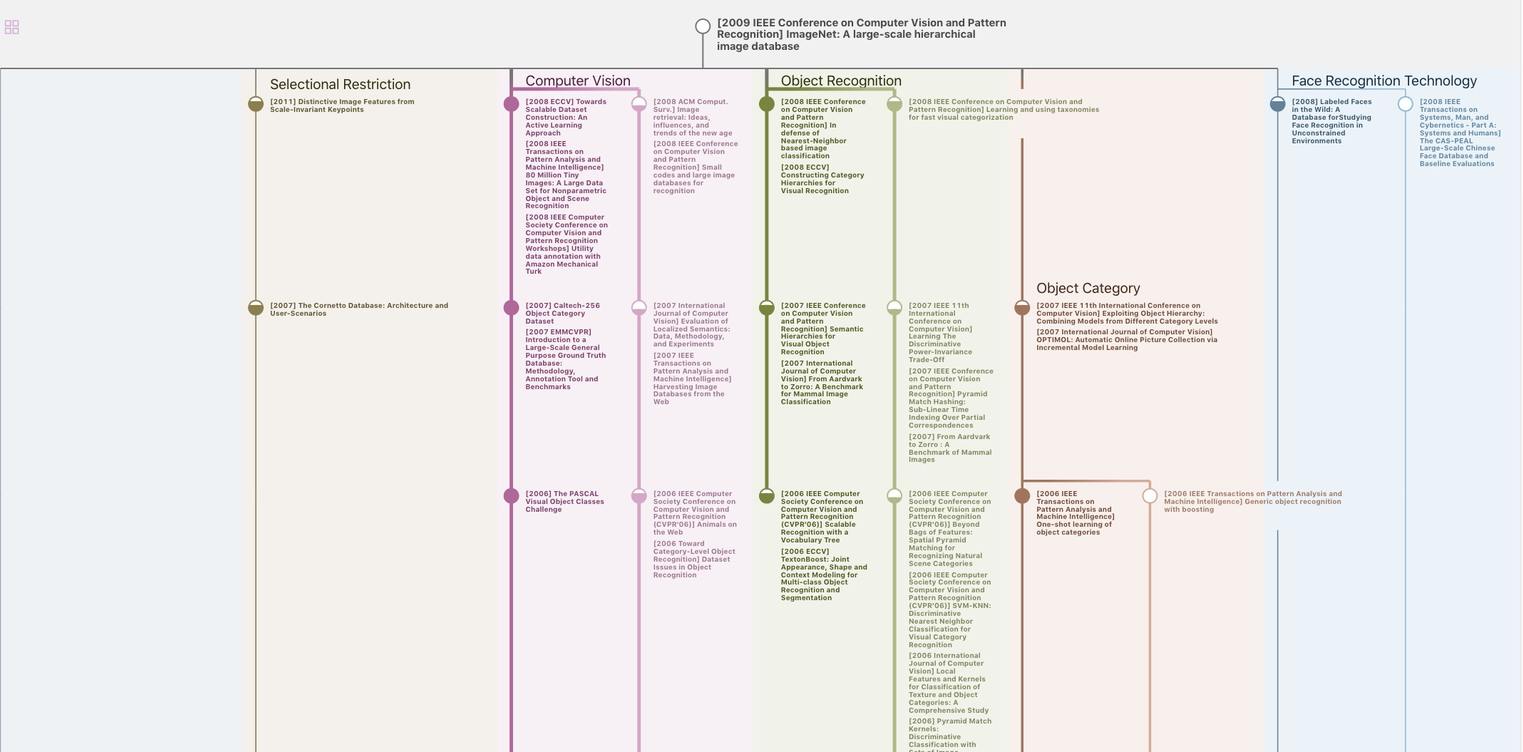
生成溯源树,研究论文发展脉络
Chat Paper
正在生成论文摘要