A Low-Rank Bayesian Temporal Matrix Factorization for the Transfer Time Prediction Between Metro and Bus Systems
IEEE TRANSACTIONS ON INTELLIGENT TRANSPORTATION SYSTEMS(2024)
Abstract
Accurate transfer time prediction and future transfer time information are important for both public transport operators and passengers. However, existing studies cannot effectively manage high-dimensional transfer time data, capture the complex nonlinearity of transfer time, or provide accurate transfer time information. This study provides a reliable prediction model called low-rank Bayesian temporal matrix factorization (LBTMF) to address these challenges. First, on the basis of a high-dimensional spatiotemporal matrix of transfer time data, we develop a low-rank temporal-regularized matrix factorization-based imputation module to capture spatial and temporal characteristics to replace missing transfer time data. Second, to further predict the transfer time with the imputation of missing data, we propose the spatiotemporal-based Bayesian temporal matrix factorization prediction module to recover hourly and daily regular characteristics to predict the transfer time at different metro stations during various periods. Finally, the comprehensive experimental findings suggest that the LBTMF model outperforms other excellent approaches in terms of imputation efficiency, prediction accuracy, and robustness.
MoreTranslated text
Key words
time-series prediction methods,low-rank matrix factorization,spatiotemporal characteristics,high-dimensional transfer time,Public transportation
AI Read Science
Must-Reading Tree
Example
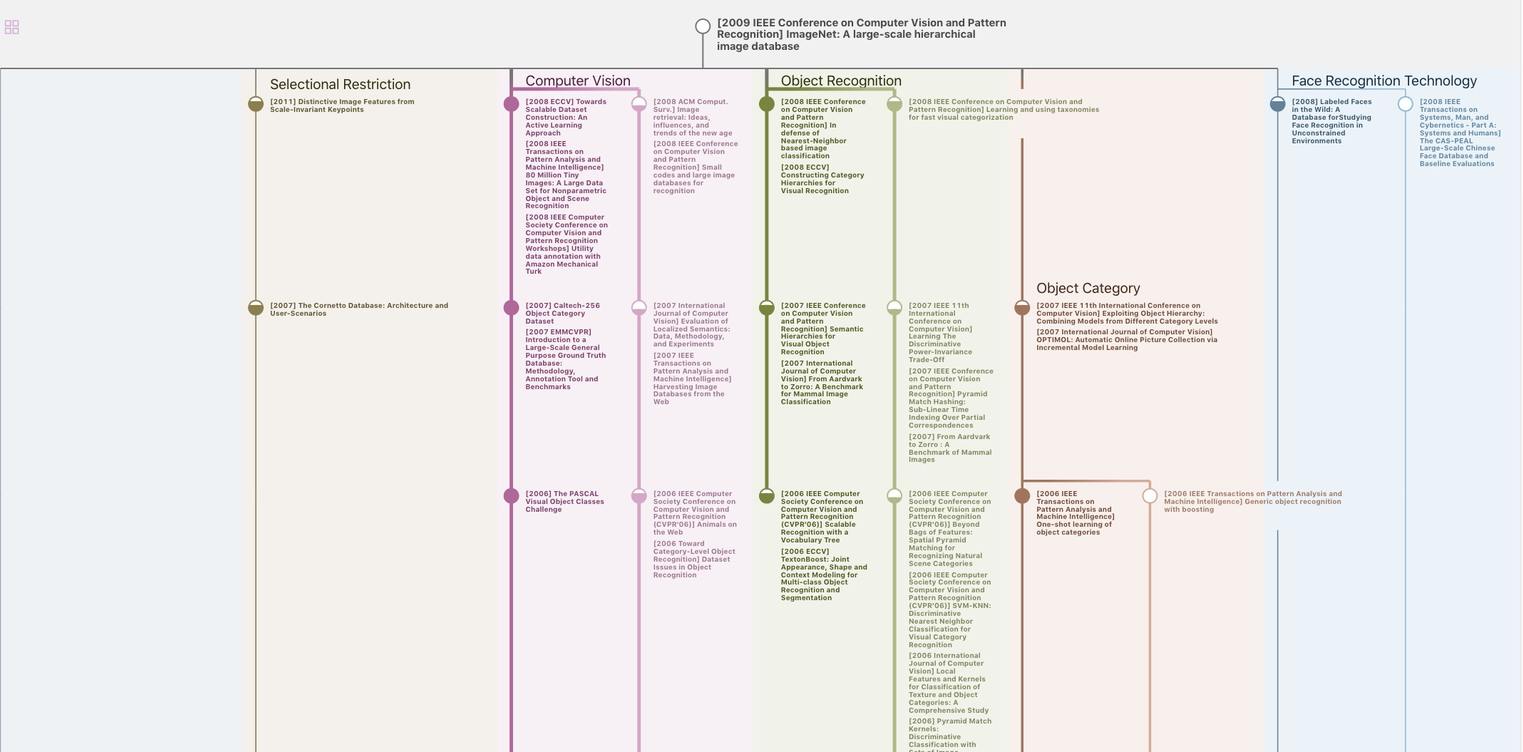
Generate MRT to find the research sequence of this paper
Chat Paper
Summary is being generated by the instructions you defined