An Efficient Deep Reinforcement Learning-based Car-following Method via Rule-constrained Data Augmentation
IEEE Transactions on Intelligent Vehicles(2024)
摘要
This paper introduces a data augmentation method based on control theory and rule constraints, aiming to address the insufficient coverage issue in existing vehicle trajectory datasets. Grounded in control theory, the method combines the motion and safety rules of driving vehicles for data augmentation. It exhibits high interpretability, credibility, a minimal and controllable set of parameters, while maintaining physical feasibility. Additionally, a one-step Markov Decision Process is introduced to model the car following task as a short-term decision problem, adapting to unpredictable variations in the generated trajectories. Experimental results demonstrate that the proposed rule-constrained data augmentation method significantly enhances the model's performance in addressing car following issues, showcasing strong adaptability and generalization capabilities.
更多查看译文
关键词
Car Following,Deep Reinforcement Learning,Data Augmentation,One-step MDP
AI 理解论文
溯源树
样例
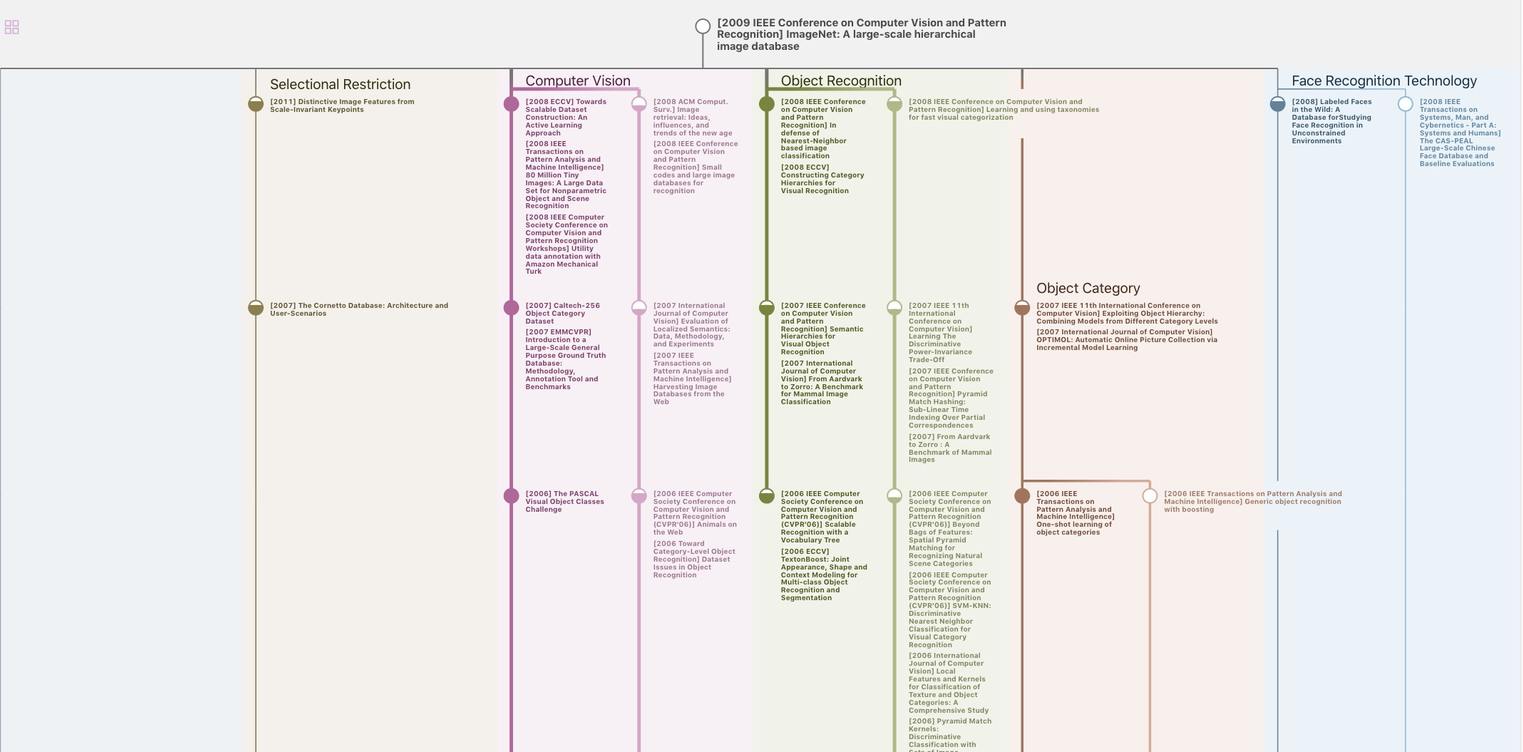
生成溯源树,研究论文发展脉络
Chat Paper
正在生成论文摘要