Dual-Scale Learning-Based Online Modeling of Nonlinear Distributed Parameter Systems Under Time-Varying Boundary Conditions
IEEE TRANSACTIONS ON INDUSTRIAL INFORMATICS(2024)
摘要
Distributed parameter systems (DPS) widely exist in many industrial processes. Traditional modeling methods are not suitable for complex DPS under time-varying boundary conditions. To handle dynamics at different scales in the spatial and temporal domains, a dual-scale incremental learning approach is proposed for the efficient modeling of the complex time-varying DPS. Under the space/time separation framework, spatial basis functions (SBF) are first designed and updated incrementally at a slow scale over a long period of time. Under the given SBF, the temporal model will be incrementally iterated in real time (fast scale). An optimal choice of the fast/slow ratio can further improve the modeling performance by better coordinating the dynamics at different scales. The experiments on the curing oven thermal process can demonstrate the effectiveness of the proposed method for modeling complex time-varying dynamics of DPS.
更多查看译文
关键词
Distributed parameter system (DPS),dual scale,Karhunen-Loeve (KL),spatiotemporal modeling,thermal process
AI 理解论文
溯源树
样例
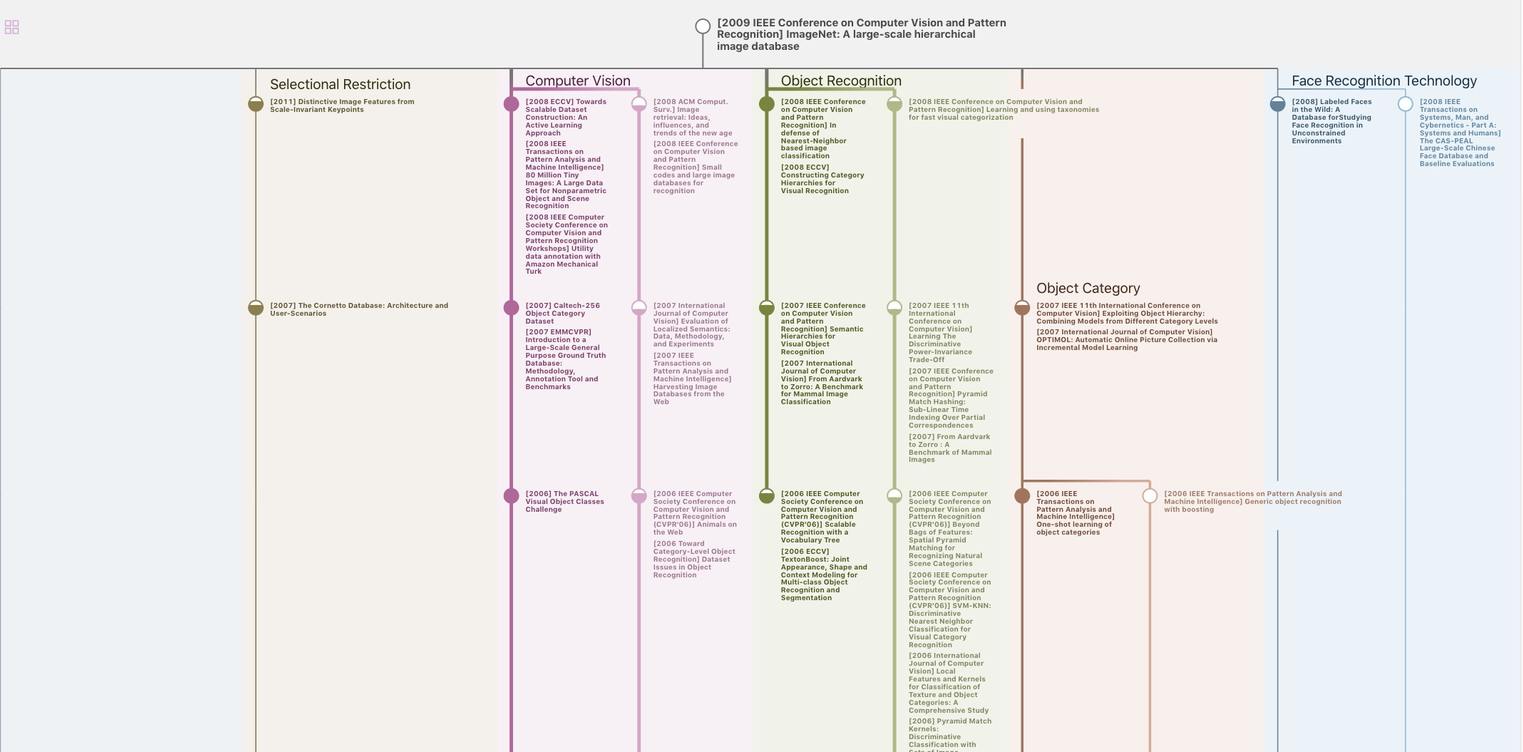
生成溯源树,研究论文发展脉络
Chat Paper
正在生成论文摘要