Learned Optimizer for Online Approximate Query Processing in Data Exploration
IEEE Transactions on Knowledge and Data Engineering(2024)
摘要
In the interactive data exploration, approximate query processing (AQP) can be used to quickly return query results at the cost of accuracy. For online AQP, the sampler can be treated as an operator in the query plan. During the query optimization for AQP, heuristic rules are usually used to guide the sampler push-down. However, due to the complexity and changes of data distribution, the heuristic rule-based optimization methods cannot meet the users' query accuracy requirements. In this paper, we propose a learning-based query optimization method for online AQP. We first introduce the weak equivalence concept and propose a series of push-down rules to guide the sampler push-down during the query optimization. Then, to enable more queries to meet the users' query accuracy requirements, we propose a deep learning model to further optimize the query plan. By using this model during each push-down process of the sampler, we try to avoid the negative effect of inappropriate sampler push-down on query accuracy, especially when there is an inconsistency between the underlying and intermediate data distribution. Extensive experiments show that the method proposed in this paper can outperform the state-of-the-art online sampling-based AQP method by 1.2×-7.9× in query accuracy.
更多查看译文
关键词
Approximate query processing,interactive data exploration,sampling,query optimization
AI 理解论文
溯源树
样例
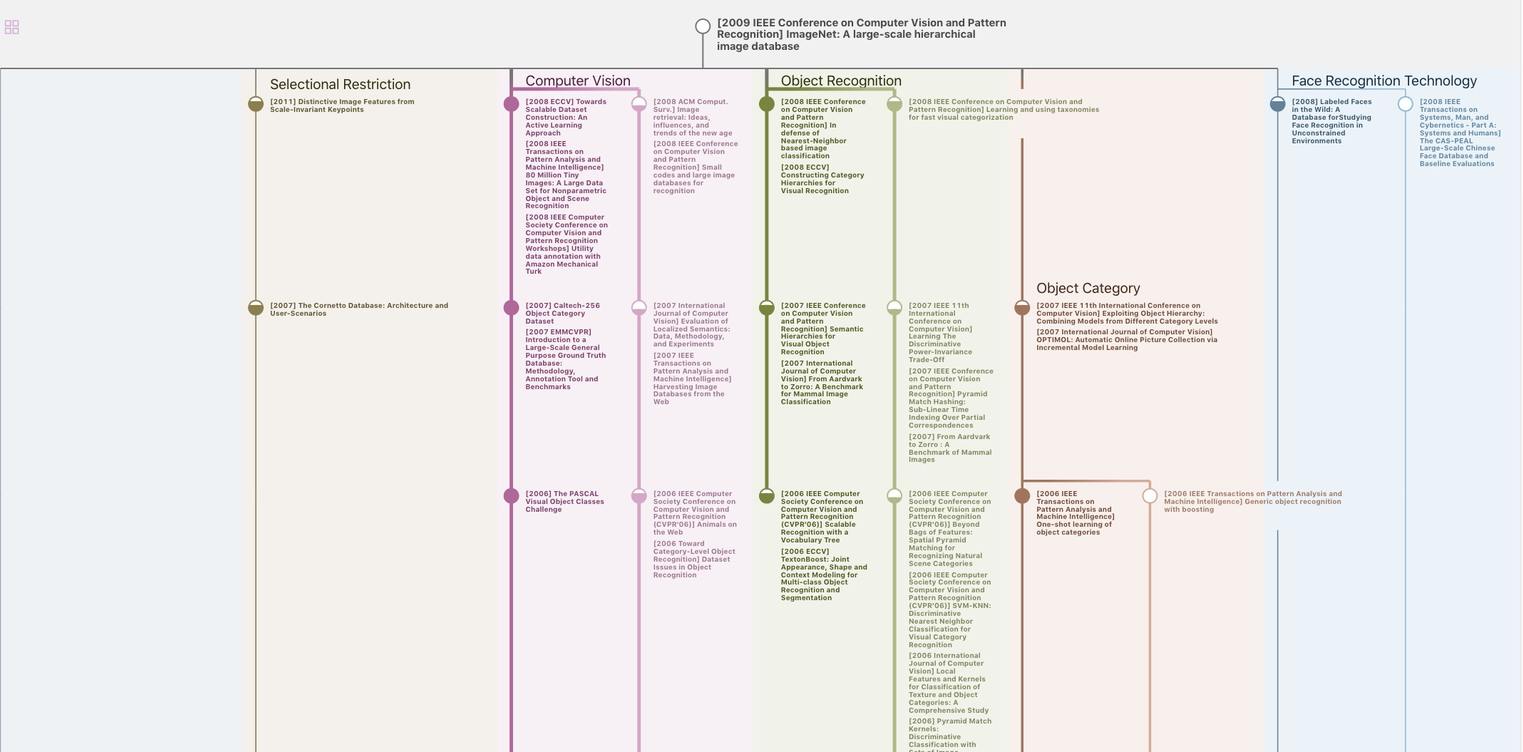
生成溯源树,研究论文发展脉络
Chat Paper
正在生成论文摘要