Using methods from dimensionality reduction for active learning with low query budget
IEEE Transactions on Knowledge and Data Engineering(2024)
摘要
Recently, it has been challenging to generate enough labeled data for supervised learning models from a large amount of free unlabeled data due to the high cost of the labeling process. Here, the active learning technique provides a solution by annotating a small but highly informative set of unlabeled data. This ensures high generalizability in space and improves classification performance with test data. The task is more challenging when the query budget is small, the data is imbalanced, multiple classes are present, and no predefined knowledge is available. To address these challenges, we present a novel active learner geometrically based on principal component analysis (PCA) and linear discriminant analysis (LDA). The proposed active learner consists of two phases: The PCA-inspired exploration phase, in which regions with high variances are explored, and the LDA-inspired exploitation phase, in which boundary points between classes are selected. The proposed geometric strategy improves the search capabilities of the active learner, allowing it to explore the space of minority classes even with multiple minority classes and a small query budget. Experiments on synthetic and real binary and multi-class imbalanced data show that the proposed algorithm has significant advantages over multiple known active learners.
更多查看译文
关键词
Active learning,Dimensionality reduction,PCA,LDA,Imbalanced data
AI 理解论文
溯源树
样例
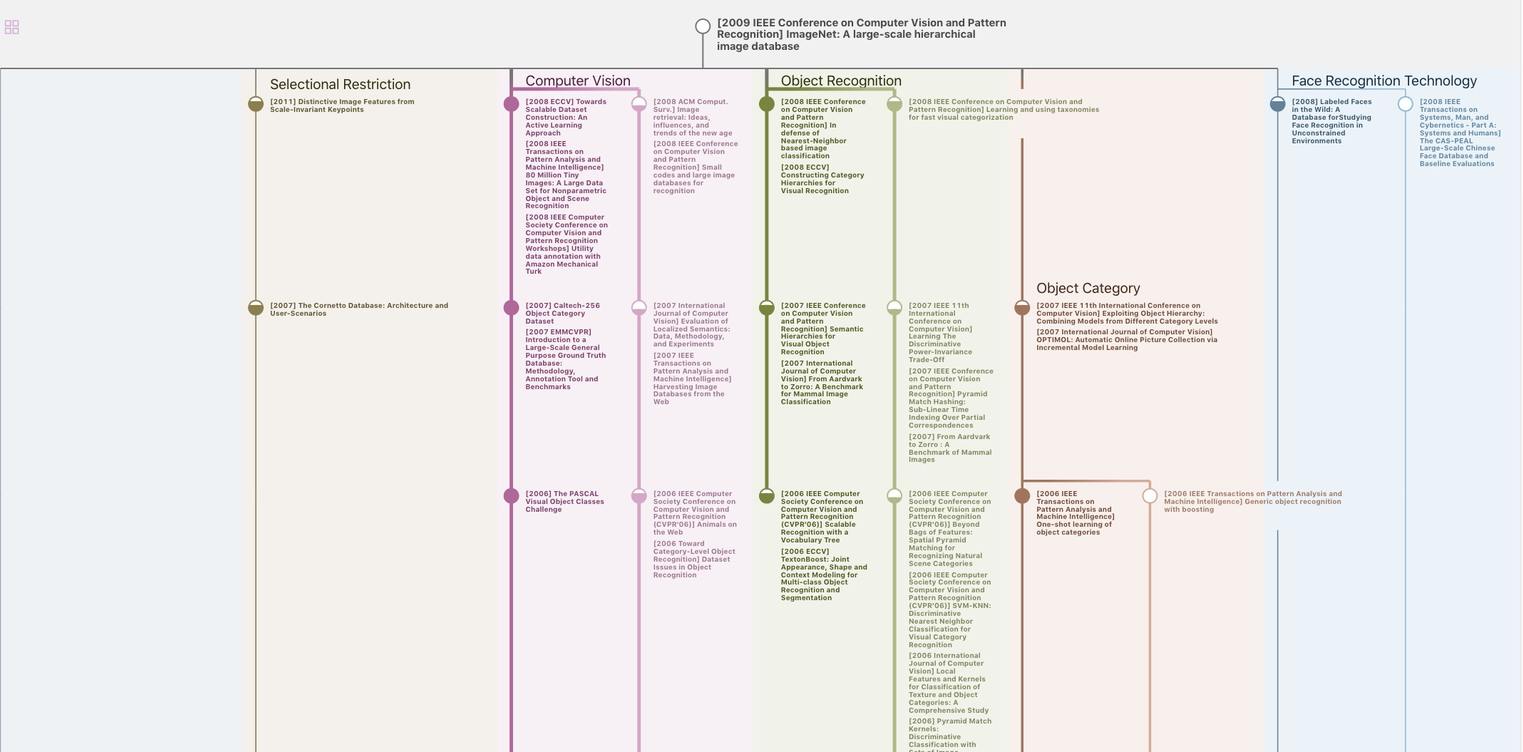
生成溯源树,研究论文发展脉络
Chat Paper
正在生成论文摘要