PT-Net: Pyramid Transformer Network for Feature Matching Learning
IEEE TRANSACTIONS ON INSTRUMENTATION AND MEASUREMENT(2024)
摘要
In this article, we propose a novel pyramid transformer network (PT-Net) for feature matching problems. Recent studies have used the dense motion field to transform unordered correspondences into ordered motion vectors and have used convolutional neural networks (CNNs) to extract deep features. However, the limited receptive field of CNNs restricts the ability of the network to capture global information within the motion field. To tackle this limitation, we devise a pyramid transformer (PT) block to enhance the models ability to extract both local and global information from the motion field, which fuses multiscale motion field information by constructing a pyramid-structured motion field. Furthermore, to alleviate the high memory demands of spatial attention in the transformer, we introduce dilated sparse attention (DSA), a novel attention block that reduces the computational difficulty of multihead self-attention (MHSA) through regular interval sampling and deconvolution operations and focuses on the essential regions to establish long-range dependencies between the correct motion vectors. The proposed PT-Net is effective in inferring the probabilities of correspondences belonging to either inliers or outliers, while simultaneously estimating the essential matrix. Extensive experiments demonstrate that PT-Net network outperforms state-of-the-art methods for outlier removal tasks and camera pose estimation on different datasets, including YFCC100M and SUN3D. The code is available at https://github.com/gongzhepeng/PT-Net.
更多查看译文
关键词
Vectors,Transformers,Feature extraction,Task analysis,Cameras,Pose estimation,Memory management,Camera pose estimation,deep learning,feature matching,outlier removal
AI 理解论文
溯源树
样例
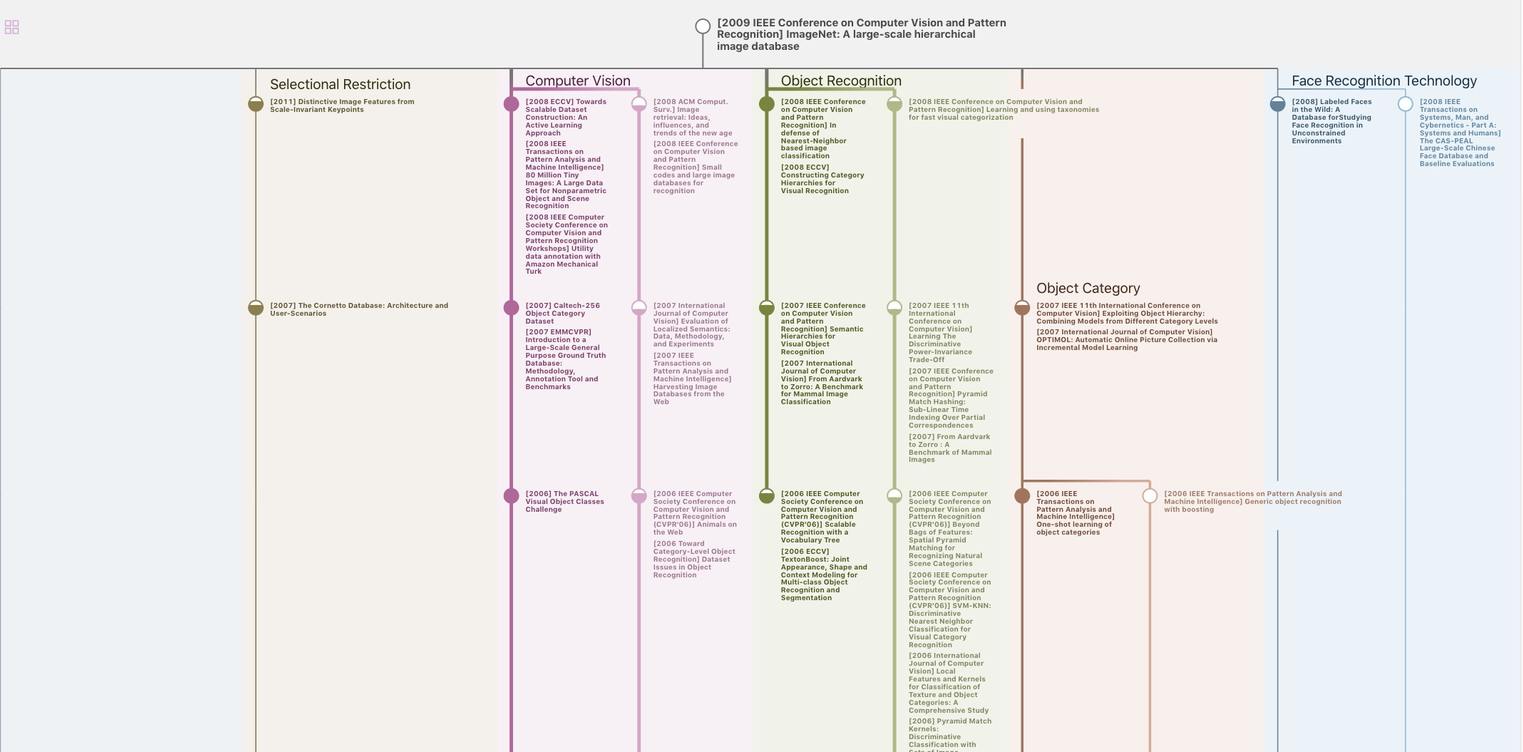
生成溯源树,研究论文发展脉络
Chat Paper
正在生成论文摘要