Joint Category Compactness and Disturbance Reduction for Cross-Domain Classification
IEEE TRANSACTIONS ON INSTRUMENTATION AND MEASUREMENT(2024)
Abstract
In cross-domain classification, the conventional methods typically focus on enhancing sample correlation to address domain shift. However, these methods often overlook a critical factor influencing classifier decisions, i.e., the discrimination between the nearest instances from different categories. To address this issue, this article proposes a novel joint category compactness and disturbance reduction (CCDR) method for cross-domain classification. CCDR aims to enhance the discriminative capability of transformed features by addressing two fundamental aspects, which are the reinforcement of intraclass compactness and the reduction of irrelevant feature interference, respectively. To enhance intraclass compactness, CCDR introduces two metrics, a locality metric and a cluster metric within categories. These metrics can facilitate a shift in the data distribution perspective from domain-centric to category-centric, thereby augmenting the tightness of intraclass data clusters. To reduce the influence of irrelevant information, CCDR proposes a nearest-class discriminant strategy. This strategy widens the margin between the nearest instances from different categories, thereby effectively minimizing classifier misjudgments. Additionally, CCDR adopts Mahalanobis distance metric instead of Euclidean distance matric to capture feature correlations more effectively. The experimental results on five datasets demonstrate that CCDR outperforms some state-of-the-art methods in terms of cross-domain classification accuracy. Additionally, t-test results reveal a significant difference between the experimental results of CCDR and those of most comparison methods.
MoreTranslated text
Key words
Cross-domain classification,domain adaptation,domain shift,metric learning,nearest neighbor (NN)
AI Read Science
Must-Reading Tree
Example
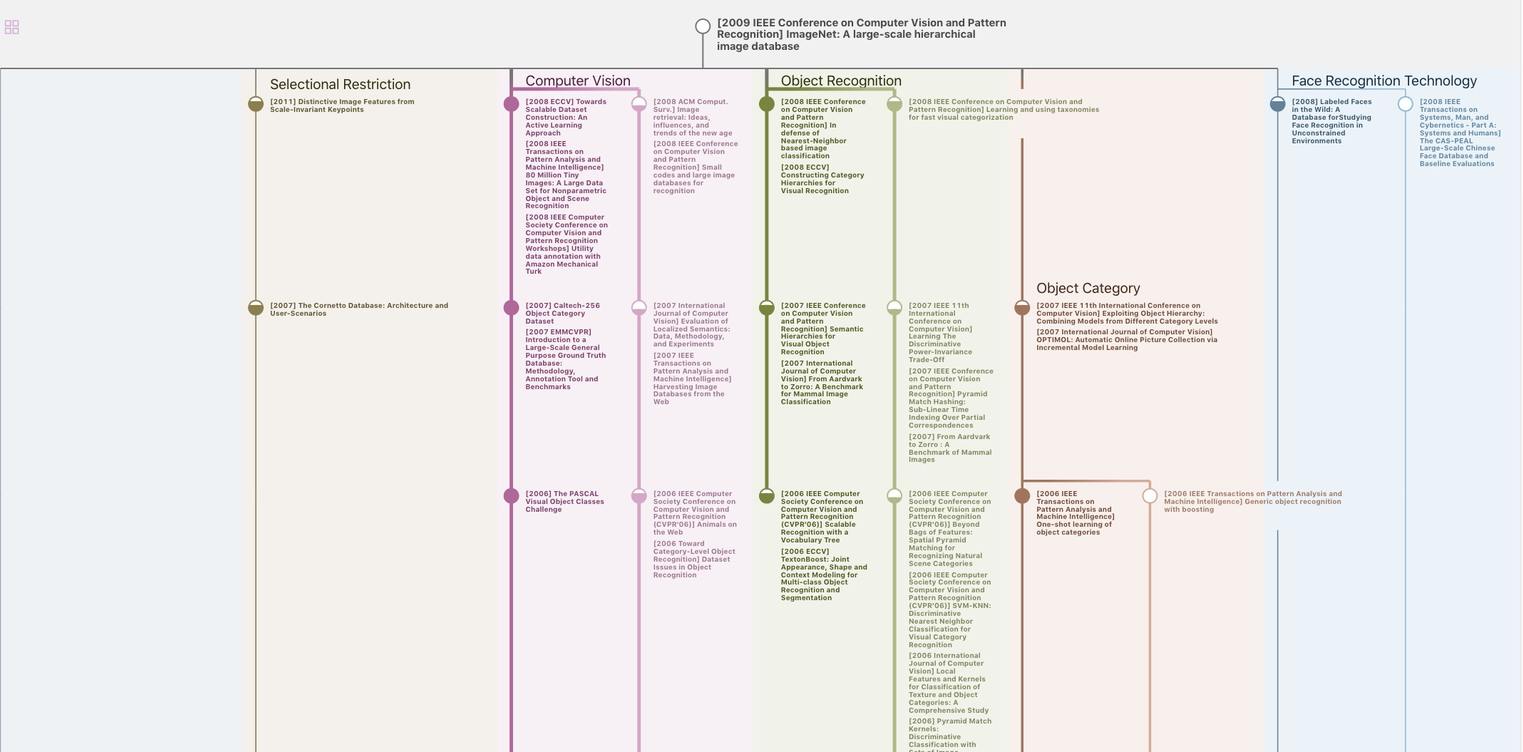
Generate MRT to find the research sequence of this paper
Chat Paper
Summary is being generated by the instructions you defined