Gradient Guided Multi-scale Feature Collaboration Networks for Few-Shot Class-incremental Remote Sensing Scene Classification
IEEE Transactions on Geoscience and Remote Sensing(2024)
摘要
Few-shot class-incremental learning has recently received significant research focus in remote sensing scene classification (FSCIL-RSSC). The success of FSCIL-RSSC relies on the robustness of the feature backbone and classifiers. Existing works focus on improving classifier adaptation, but little attention is paid to the importance of backbone robustness on the recognition ability of new class samples’ embeddings. Due to the large distribution shift between old and new classes, FSCIL-RSSC utilizing high-layer (single-scale) features may not adapt flawlessly to new categories. To solve the issue, we put forward a Gradient Guided Multi-Scale Feature Collaboration Network (G-MFCN) for FSCIL-RSSC. Specifically, we introduce a parallel hierarchy strategy to simultaneously capture the multi-feature discriminative information of the same sample. Then, a gradient guide block is designed to automatically pick out the optimal values of different convolution blocks for multi-feature fusion. Finally, the classical feature pyramid network is introduced for multi-scale fusion to obtain more obvious discriminative features of RSSC. More importantly, our proposed G-MFCN is a simple and adaptable module, which can combine any existing FSCIL frameworks to further improve the optimized classifiers’ effectiveness for the FSCIL-RSSC scenario. Extensive experiments on four benchmarks demonstrate that the proposed G-MFCN achieves significant improvements in comparison to existing FSCIL-RSSC methods.
更多查看译文
关键词
Remote sensing scene classification,few-shot class-incremental learning,multi-scale feature collaboration
AI 理解论文
溯源树
样例
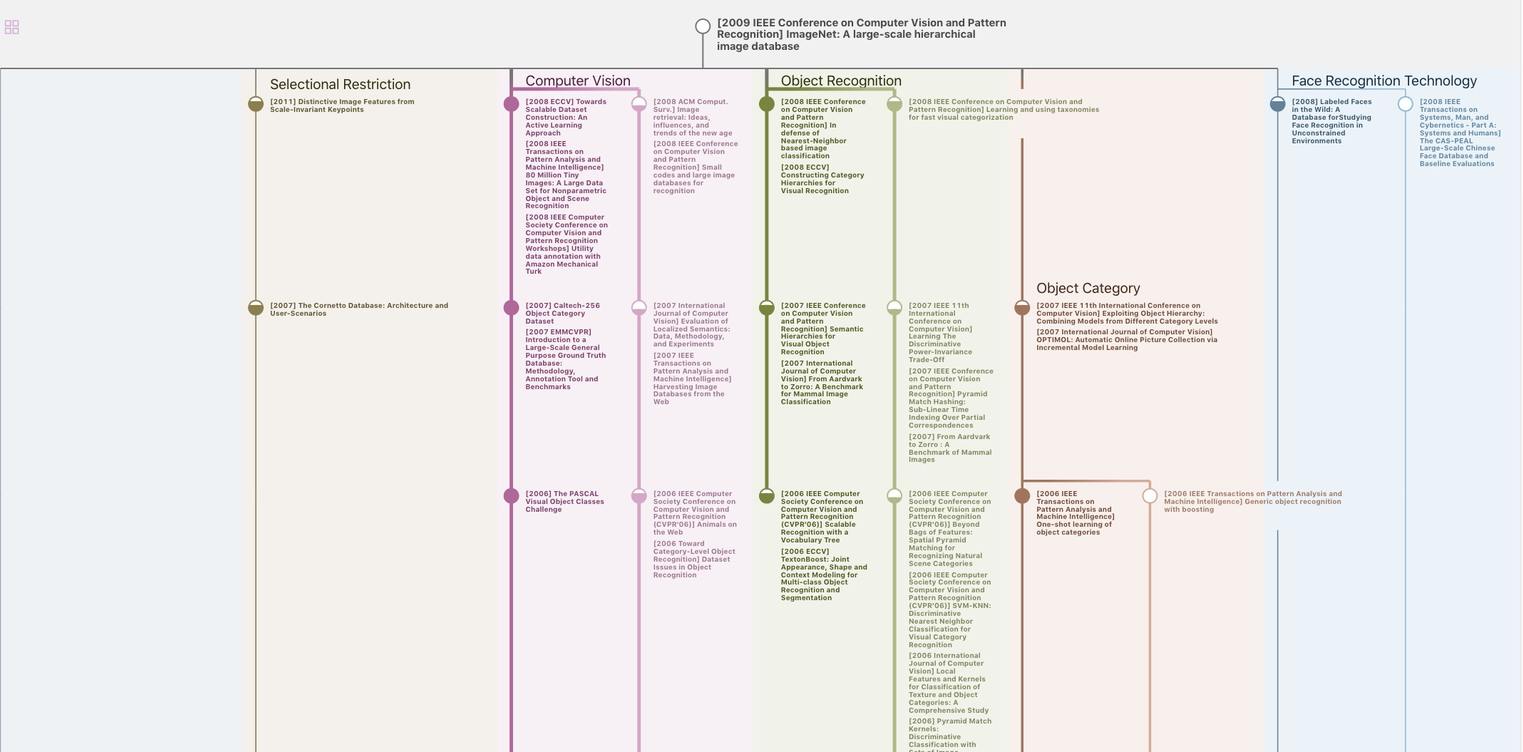
生成溯源树,研究论文发展脉络
Chat Paper
正在生成论文摘要