Cross-Modal Hashing With Feature Semi-Interaction and Semantic Ranking for Remote Sensing Ship Image Retrieval.
IEEE Trans. Geosci. Remote. Sens.(2024)
摘要
Cross-modal hashing plays a pivotal role in large-scale remote sensing (RS) ship image retrieval. RS ship images often exhibit similar overall appearance with subtle differences. Existing hashing methods typically employ feature non-interaction strategies to generate common hash codes, which may not effectively capture the correlations between cross-modal ship images to reduce inter-modality discrepancies. To address this issue, we propose a novel cross-modal hashing approach based on Feature Semi-Interaction and Semantic Ranking (FSISR) for RS ship image retrieval. Our FSISR approach not only captures intricate correlations between different ship image modalities, but also enables the construction of hash tables for large-scale retrieval. FSISR comprises a feature semi-interaction module and a semantic ranking objective function. The semi-interaction module utilizes clustering centers from one modality to learn the correlations between two modalities and generate robust shared representations. The objective function optimizes these representations in a common Hamming space, consisting of a shared semantic alignment loss and a margin-free ranking loss. The alignment loss employs a shared semantic layer to preserve label-level similarity, while the ranking loss incorporates hard examples to establish a margin-free loss that captures similarity ranking relationships. We evaluate the performance of our method on benchmark datasets and demonstrate its effectiveness for cross-modal RS ship image retrieval. https://github.com/sunyuxi/FSISR.
更多查看译文
关键词
Remote sensing ship image retrieval,deep supervised hashing,cross-modal remote sensing ship images,multi-source remote sensing images,learning to hash
AI 理解论文
溯源树
样例
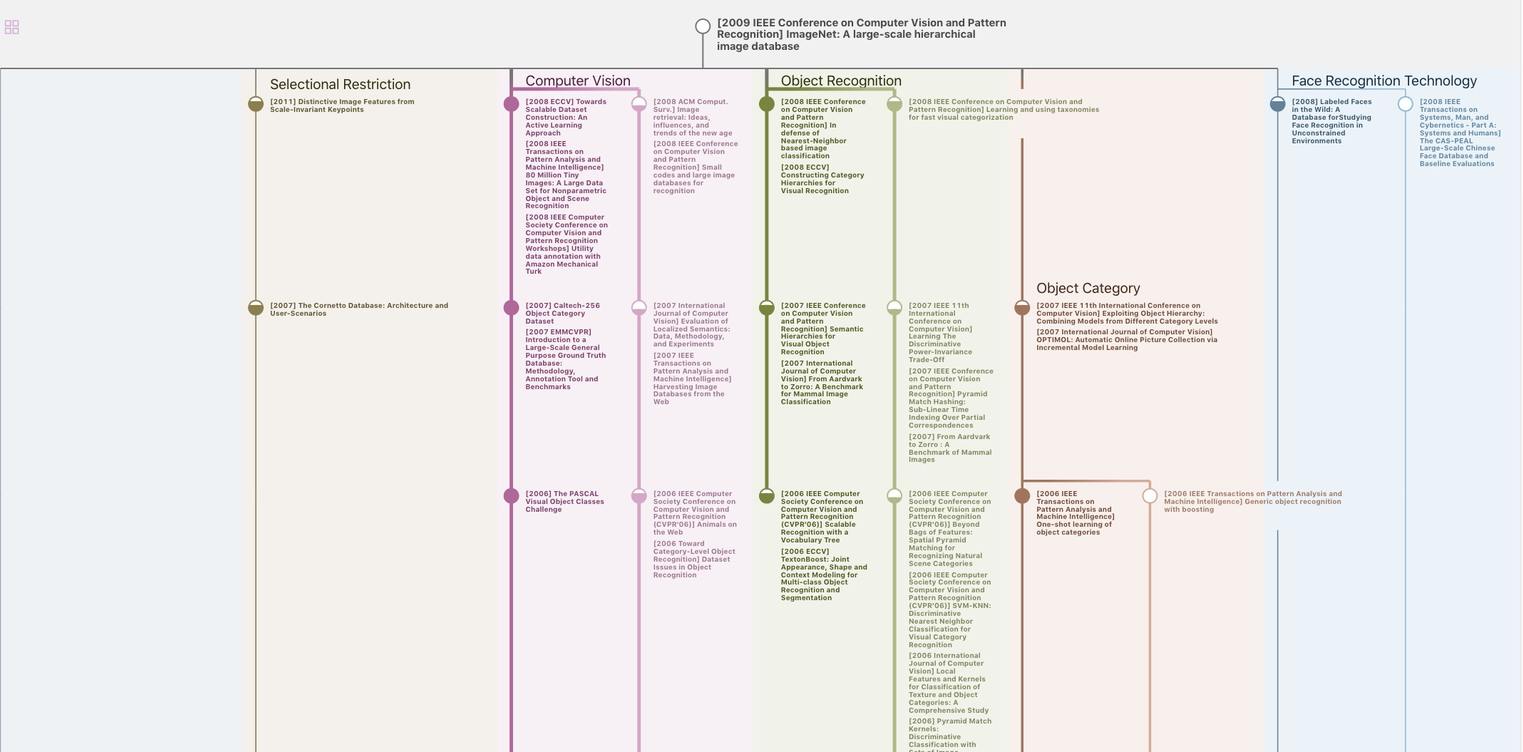
生成溯源树,研究论文发展脉络
Chat Paper
正在生成论文摘要