Coverage Path Planning for IoUAVs With Tiny Machine Learning in Complex Areas Based on Convex Decomposition.
IEEE Internet Things J.(2024)
Abstract
For Unmanned Aerial Vehicles (UAVs) with Tiny Machine Learning (TML), there is mutual exclusivity between the energy consumption for flight and the energy consumption to support their computation and processing. IoUAVs integrated with TML systems often consume substantial amounts of energy during flights, particularly when engaged in extended coverage and surveillance missions. The energy consumption of a UAV with TML performing long, wide-area coverage patrols and monitoring missions in complex areas is significant for the flight itself, and the energy required for the TML to perform calculations and processing is not guaranteed. Therefore, to better support TML computations, this study optimizes flight paths to reduce the energy consumption of UAVs while ensuring coverage. Specifically, in this study, the use of concave point elimination algorithms, enhanced convex decomposition algorithms, and determination of flight direction significantly reduced the frequency of UAV turns. The computational cost of obtaining a complete path is reduced by merging the subconvex regions and the weighted minimum traversal of the graph. This novel bidirectional forwarding path coverage path-planning (BFP-CPP) algorithm maximizes the reduction in the number of turns, reduces energy consumption, and achieves global coverage. The simulation experimental results show that compared with the existing methods without concave point elimination, the BFP-CPP algorithm can effectively reduce the number of subregions, minimize the number of drone turns, and lower energy consumption.
MoreTranslated text
Key words
Convex Decomposition,Internet of Unmanned Aerial Vehicles (IoUAV),minimum width sum,path planning,tiny machine learning,UAV
AI Read Science
Must-Reading Tree
Example
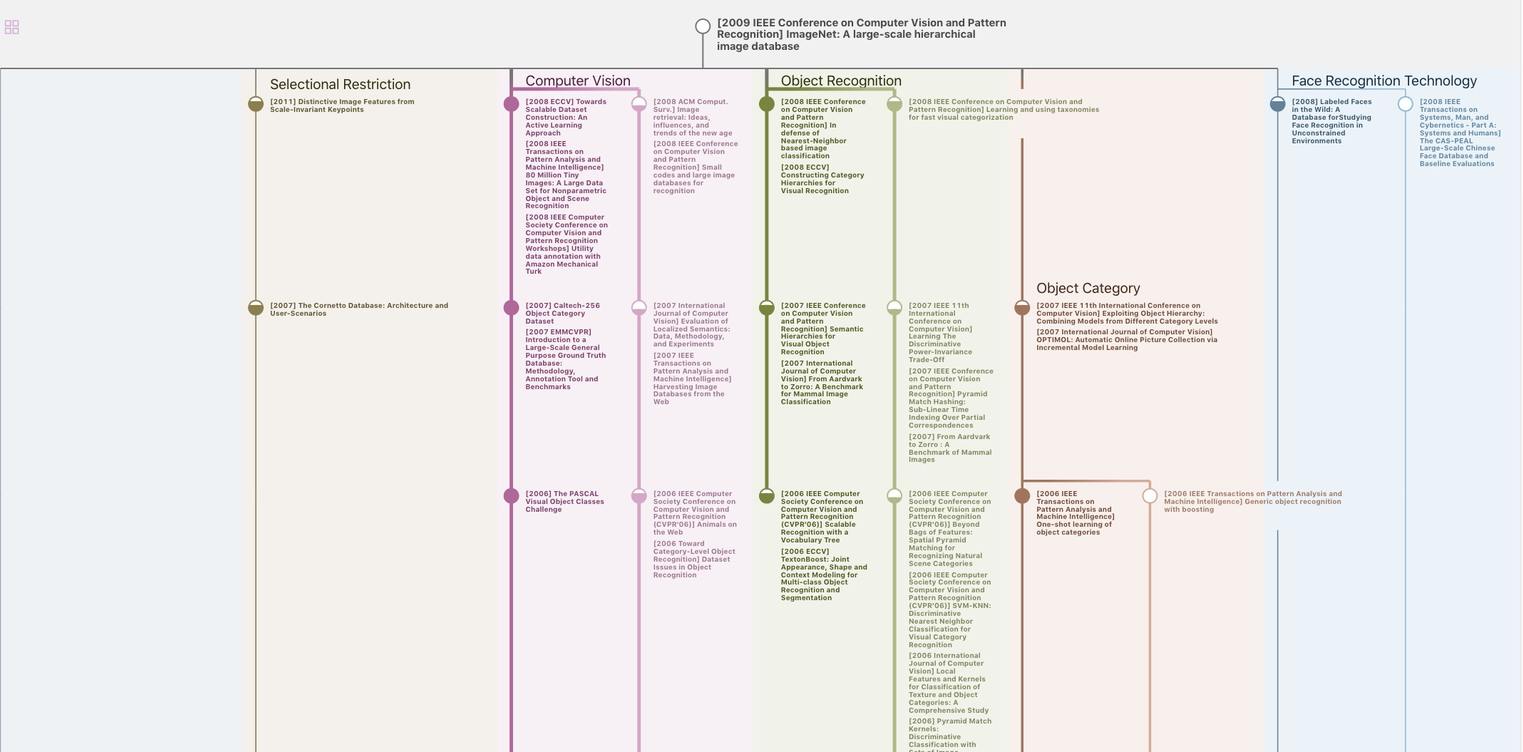
Generate MRT to find the research sequence of this paper
Chat Paper
Summary is being generated by the instructions you defined