ECPS: Cross Pseudo Supervision Based on Ensemble Learning for Semi-Supervised Remote Sensing Change Detection.
IEEE Trans. Geosci. Remote. Sens.(2024)
摘要
Semi-supervised learning aims to exploit the potential of unlabeled data to enhance model performance, which makes it suitable for addressing the challenge of limited labeled data. As a popular technology, pseudo-label is widely applied in many semi-supervised remote sensing (RS) change detection methods. However, when facing limited labeled data, abundant low-quality pseudo-labels from a poorly-performing model hinder the effective enhancement of model performance. To address this issue, we propose a novel semi-supervised strategy, named ensemble cross pseudo supervision (ECPS). The utilization of ensemble learning to merge outputs from several change detection models enhances pseudo-label quality, leading to more accurate change information and a significant boost in model performance, even with limited labeled data. In this method, adopting crosswise supervision ensures that no additional inference costs caused by ensemble learning are consumed. This provides both high efficiency and effectiveness for identifying land-cover changes. On the other hand, a simple yet effective ensemble strategy is proposed, which allows to manually adjust the model’s tendency towards higher precision or recall for satisfying practical requirements. We conduct extensive experiments on four public RS change detection datasets, and the promising results demonstrate the superiority of the proposed method across various numbers of labeled samples. Our source codes are available at https://github.com/TangXu-Group/ECPS.
更多查看译文
关键词
Change detection,ensemble learning,remote sensing (RS),semi-supervised learning (SSL),pseudo-label
AI 理解论文
溯源树
样例
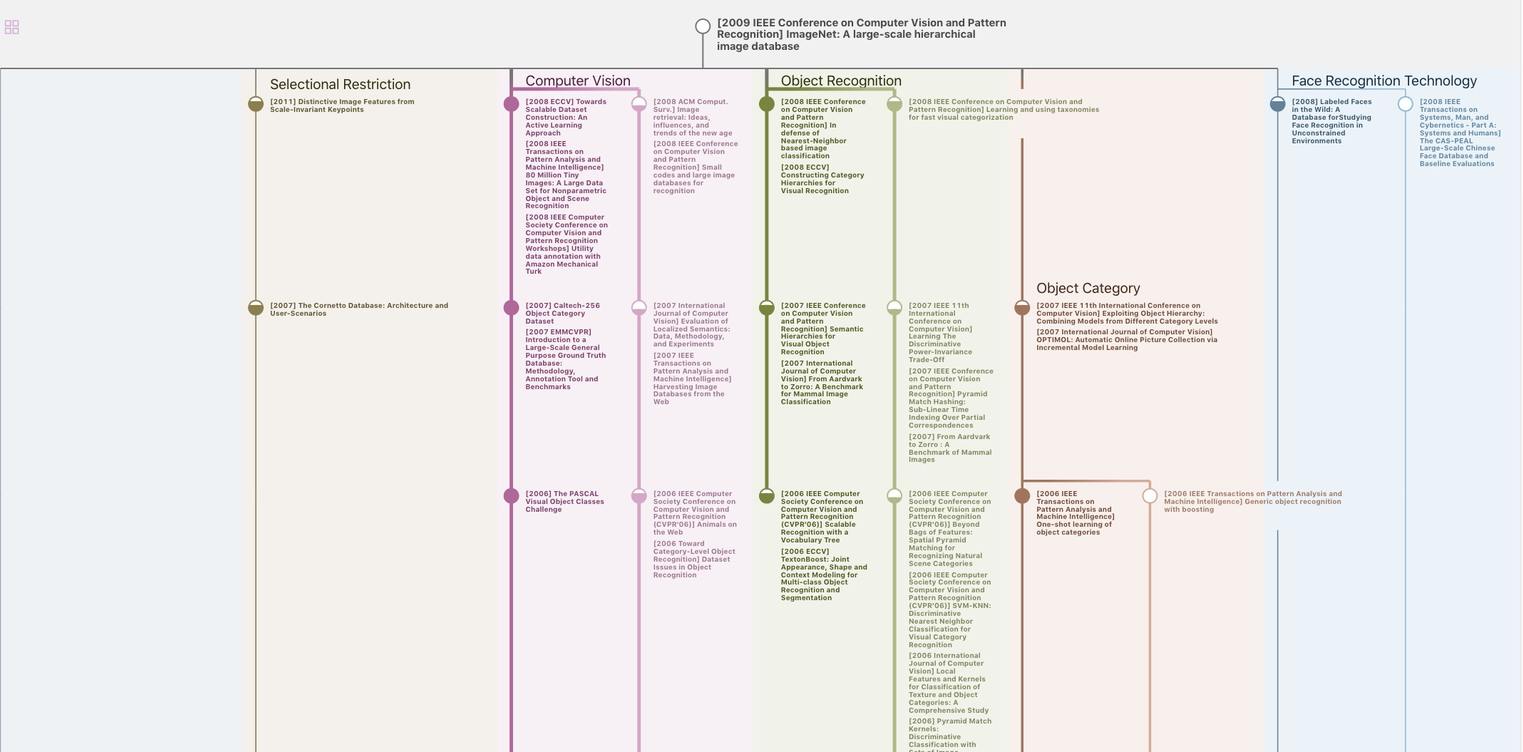
生成溯源树,研究论文发展脉络
Chat Paper
正在生成论文摘要