A Sample Augmentation Method for Side-Scan Sonar Full-Class Images That Can Be Used for Detection and Segmentation
IEEE TRANSACTIONS ON GEOSCIENCE AND REMOTE SENSING(2024)
Abstract
To solve the problems of small samples, acquisition difficulties, under representation and labeling difficulties in object detection, recognition, and segmentation tasks for underwater all-category targets based on sonar images and deep learning methods, we propose a side-scan sonar (SSS) full-class image sample augmentation method suitable for multitasking scenarios. Based on the superior image generation ability of the diffusion model, we use transfer learning to fine-tune the optical pretrained model to build a side-scan sonar image generation model. Then, for the object detection task and semantic segmentation task, we use the image content and target shape as guidance information to guide the generation results of the diffusion model, respectively. Meanwhile, proposed a mask synthesis method for SSS waterfall image generation based on the working principle of side-scan sonar. The synthesized mask images are used to guide the generation of side-scan sonar waterfall images. Finally, the underwater object detection and segmentation models are trained on the generated data. The experiment results show that training a model with generated data can be effective in improving accuracy.
MoreTranslated text
Key words
Guided generation,object detection,sample augmentation,semantic segmentation,side-scan sonar (SSS)
AI Read Science
Must-Reading Tree
Example
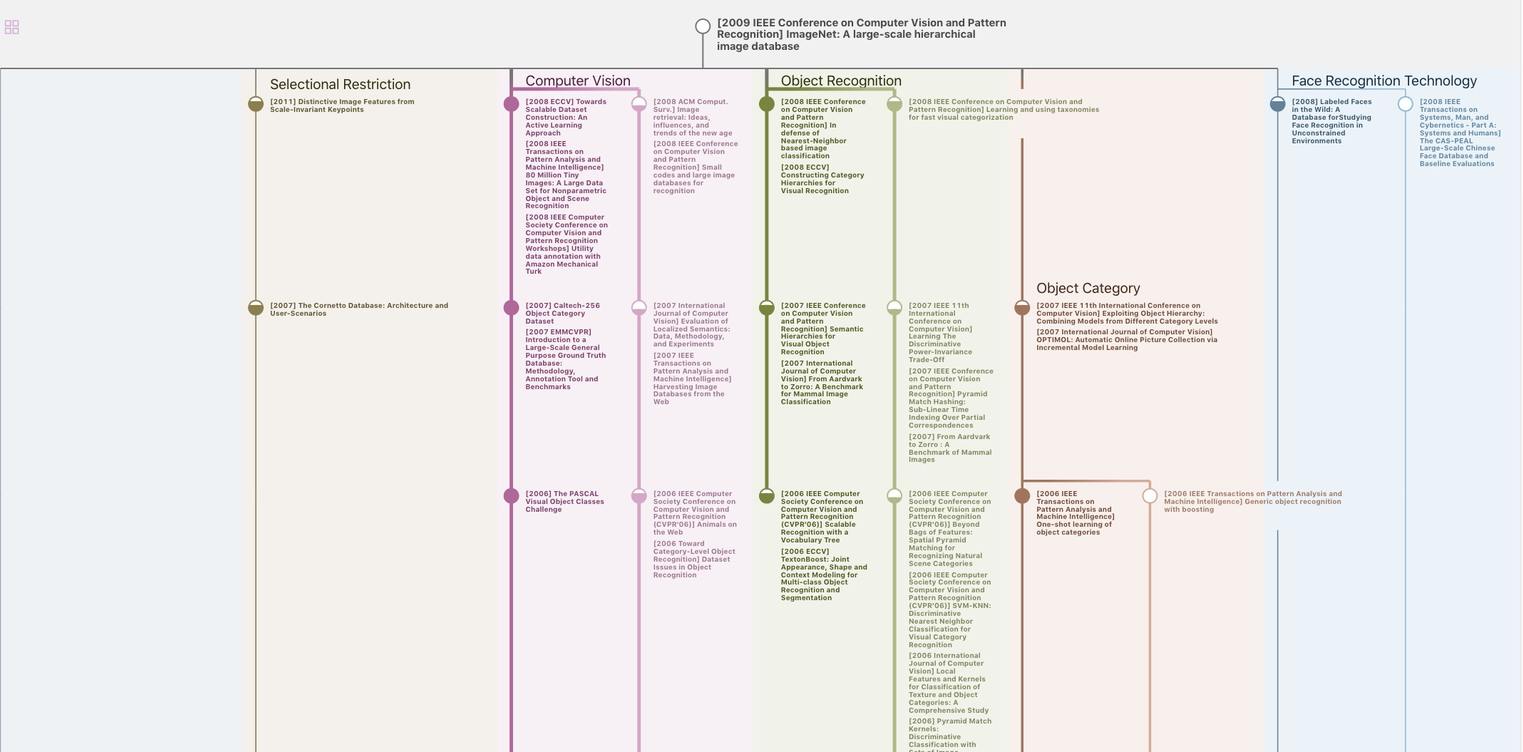
Generate MRT to find the research sequence of this paper
Chat Paper
Summary is being generated by the instructions you defined