One network fits all: A self-organizing fuzzy neural network based explicit predictive control method for multimode process
IEEE Transactions on Fuzzy Systems(2024)
摘要
Modern industrial processes often exhibit complex and uncertain operating state fluctuations due to the diversification of production materials, the complexity of production processes, and the harsh production environment. To cope with the operating state fluctuations of industrial processes, fuzzy neural networks, as a form of human-inspired computing, have been introduced and widely applied. In contrast, model predictive control (MPC) typically employs a multi-model control strategy for the multimode process. However, ensuring control performance during the switching phase and meeting real-time control requirements poses challenges. To address the real-time control challenge in multimode processes, this article proposes a learning framework for fuzzy neural network explicit control for full operation conditions. The proposed framework includes two modules: the fuzzy neural network-based explicit control law (FNNECL) and the neural network-based predictive model (NNPM). By adjusting the structure and parameters of FNNECL, precise control of full operation conditions is achieved. Specifically, a novel truncated radial basis activation function is first presented, and the operation condition change measurement index of data coverage is proposed to identify the mismatch degree between current and historical operation conditions accurately. Then, for small-scale operation condition changes, an elastic weight consolidation (EWC) mechanism is introduced to ensure that FNNECL can learn control strategies for new operation conditions while maintaining control performance for historical operation conditions. Finally, to address large-scale operation condition changes, a radial basis function (RBF) neuron growth mechanism based on data coverage is proposed. This mechanism calculates the data coverage of fuzzy rules and selectively adds new neurons to the fixed structure FNNECL. This enables the explicit control law to fit large-scale changes and effectively learn control strategies for new operation conditions. It is worth noting that during the application of this method, there is no need to know the current operation conditions in advance. Based on a single FNNECL model, accurate control sequences can be rapidly obtained, overcoming the traditional online optimization problem of multiple models. This greatly expands the application scope and achieves precise control under full operation conditions. Extensive experiments including numerical simulation and industrial roasting process verified the feasibility and effectiveness of the proposed method.
更多查看译文
关键词
Multimode process,Fuzzy neural network,Explicit control law,Full operation conditions
AI 理解论文
溯源树
样例
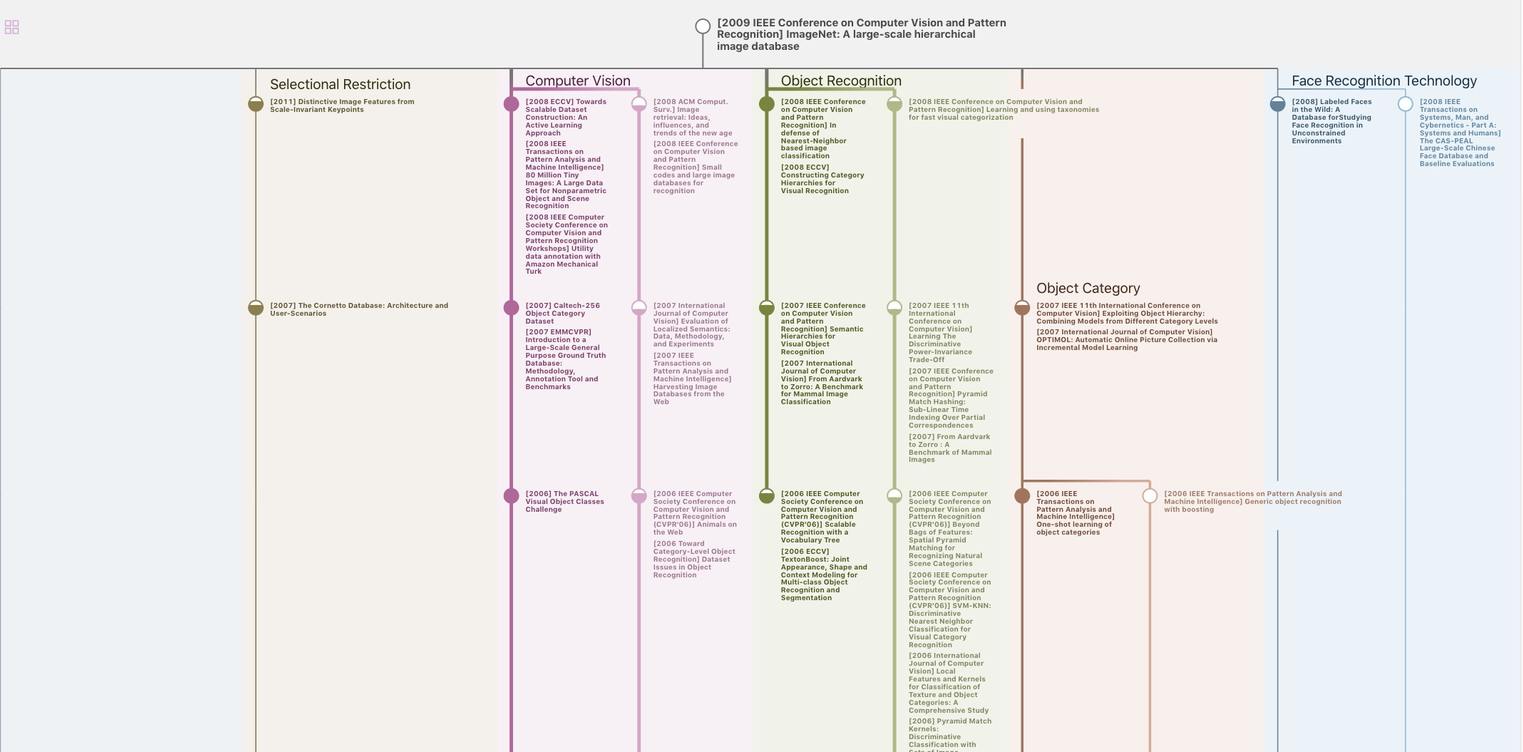
生成溯源树,研究论文发展脉络
Chat Paper
正在生成论文摘要