Predicting Water Quality With Non-stationarity: Event-Triggered Deep Fuzzy Neural Network
IEEE Transactions on Fuzzy Systems(2024)
摘要
Water quality prediction is an indispensable task in water environment and source management. The existing predictive models are mainly designed by data-driven artificial neural networks (ANNs), especially deep learning models for large-scale water quality prediction. However, the state of water environment is a dynamic process where the stationarity of water quality data suffers from time variation and human activities, which leads to a poor prediction accuracy because ANNs receive whole water quality data passively, including abnormal conditions. We consider such a tough problem in this article and propose an event-triggered deep fuzzy neural network (ET-DFNN) to pursue the better performance of water quality prediction in the complex water environment. First, a deep pretraining model is constructed to extract the effective features from raw water quality data. Second, we construct a DFNN model where the extracted effective features are considered as the input variables. Third, some events are defined to characterize the abnormal conditions of state evolution in water quality. The DFNN is trained and updated using different learning strategies only when the corresponding events are triggered, otherwise it ignores the current data sample and directly goes to the next data sample. The practical data-based experimental results show that the ET-DFNN achieves better prediction performance in accuracy and efficiency than its peers. Especially, the training efficiency of ET-DFNN is improved by 57.94% on total phosphorus prediction and 48.31% on biochemical oxygen demand prediction, respectively.
更多查看译文
关键词
Deep fuzzy neural network (DFNN),event-triggered strategy,nonstationarity,water environment,water quality prediction
AI 理解论文
溯源树
样例
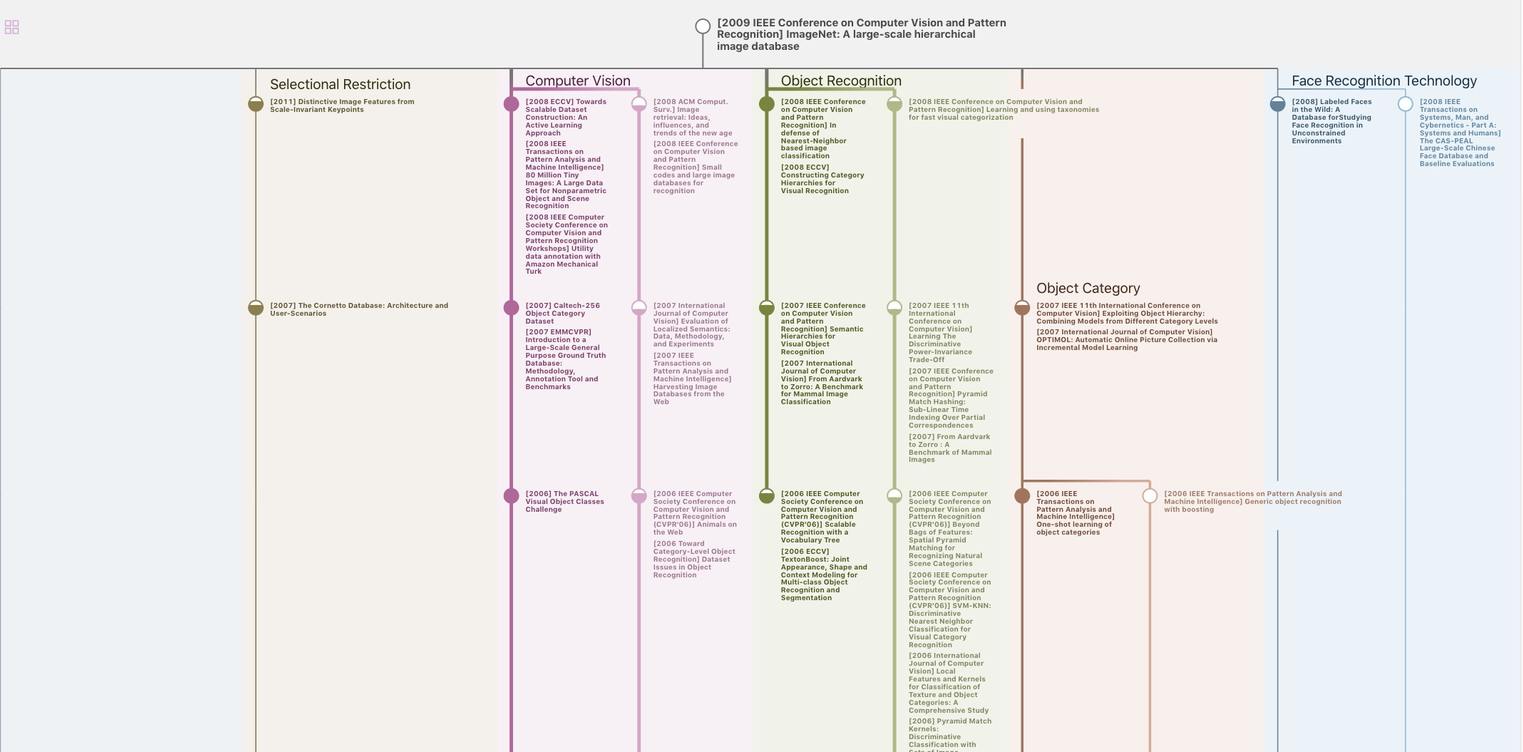
生成溯源树,研究论文发展脉络
Chat Paper
正在生成论文摘要