AccuLiteFastNet: A Remote Sensing Object Detection Model Combining High Accuracy, Lightweight Design, and Fast Inference Speed
IEEE Geoscience and Remote Sensing Letters(2024)
摘要
This letter proposes AccuLiteFastNet, a novel object detection model tailored for aerial remote sensing scenarios constructed on the YOLOv8. AccuLiteFastNet is designed to enhance the precision in detecting small-size objects while maintaining a lightweight model framework and supporting fast inference speeds for deployment. Specifically, we use the Normalized Gaussian Wasserstein Distance (NGWD) as the loss metric to evaluate the similarity between predicted and ground-truth object bounding boxes, ensuring uniform sensitivity for objects of different sizes. Moreover, we replace the model backbone’s last feature extraction module with the self-designed Multi-Scale FasterNet (MSFN) module to reduce the model complexity while providing an expanded receptive field. Finally, we propose a novel module named ContA-C2f specifically designed to evaluate the informativeness of each pixel in the image. Across three open-source remote sensing datasets, AccuLiteFastNet demonstrates a significant improvement over the original YOLOv8, achieving a 4.5% increase in mean Average Precision (mAP). Comparisons with existing object detection models, AccuLiteFastNet strikes an optimal balance with high inference speed (58.14 FPS) and good accuracy (87.5% mAP) at lower computational costs (8.3 GFLOPs).
更多查看译文
关键词
Object detection,YOLOv8,Remote sensing
AI 理解论文
溯源树
样例
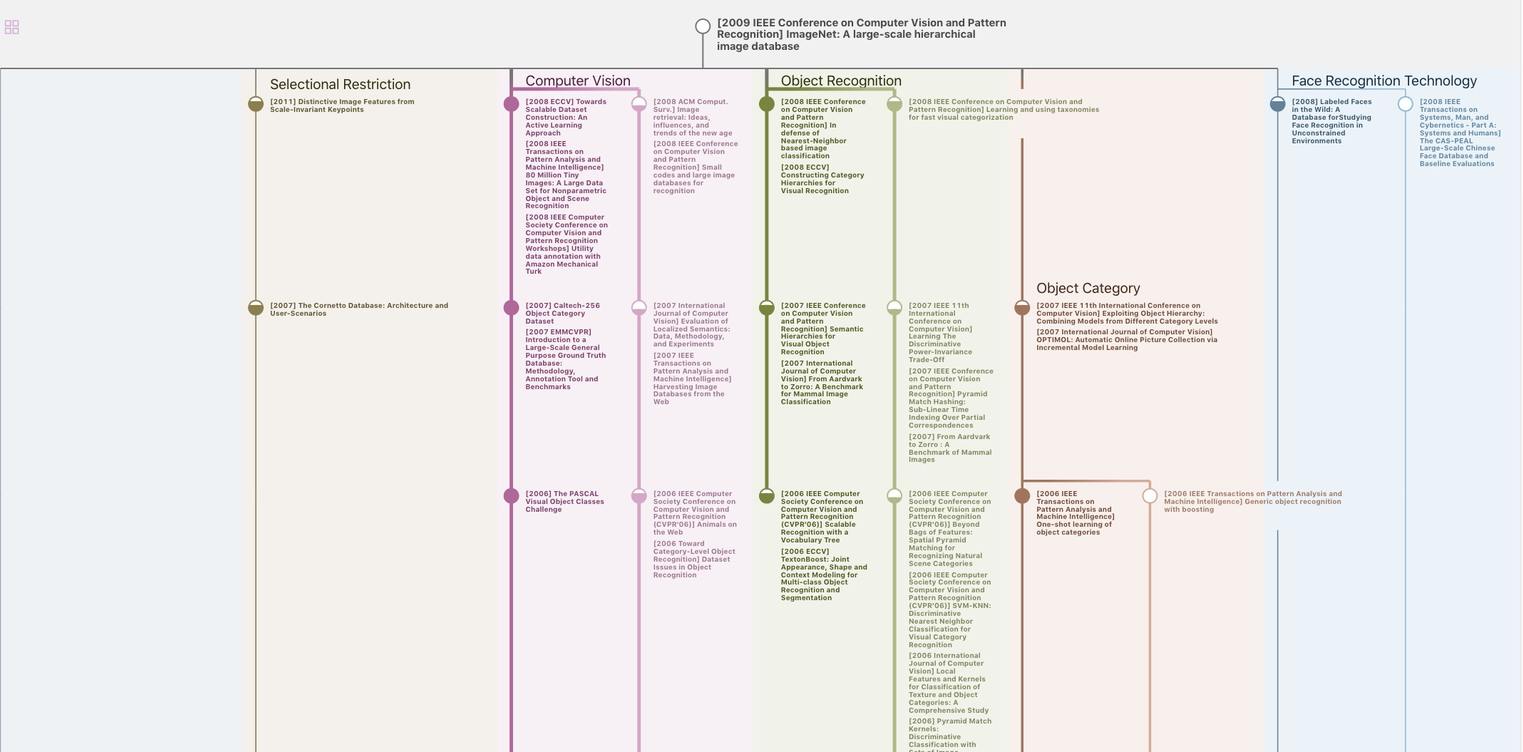
生成溯源树,研究论文发展脉络
Chat Paper
正在生成论文摘要