Spatio-Temporal Fusion Spiking Neural Network for Frame-Based and Event-Based Camera Sensor Fusion
IEEE TRANSACTIONS ON EMERGING TOPICS IN COMPUTATIONAL INTELLIGENCE(2024)
摘要
Traditional frame-based cameras capture high-resolution images at specific sampling rates while suffering from motion blur and uneven exposure. Emerging event-based cameras can address these issues with event-driven sampling, but fail to capture texture details. Additional information can be obtained by complementing the characteristics of frame- and event-based sensors. A spatio-temporal fusion spiking neural network (STF-SNN) is proposed here for fusing frame- and event-based information. STF-SNN achieves competitive recognition performance on popular datasets. For example, it achieves 95.77% accuracy on the fusion of CIFAR10 and DVS-CIFAR10, which is 5.01% and 19.27% higher than the non-fused SNN based only on the frame- or event-based information, respectively. To the best of our knowledge, this work first uses SNN to mine spatio-temporal information in the frame-event data stream. The main contributions of this work are: (1) it is proposed to fuse information in the spatio-temporal domain at the feature and decision levels, which yields great accuracy improvement; (2) a weight quantization method for STF-SNN is proposed, which well solves the parameter doubling problem caused by information fusion; (3) it is proposed to prepare data by weak correspondence between frame- and event-based data, which lowers the data preparation barrier of STF-SNN.
更多查看译文
关键词
Neuromorphic computing,sensor fusion,spiking neural network,weight quantization
AI 理解论文
溯源树
样例
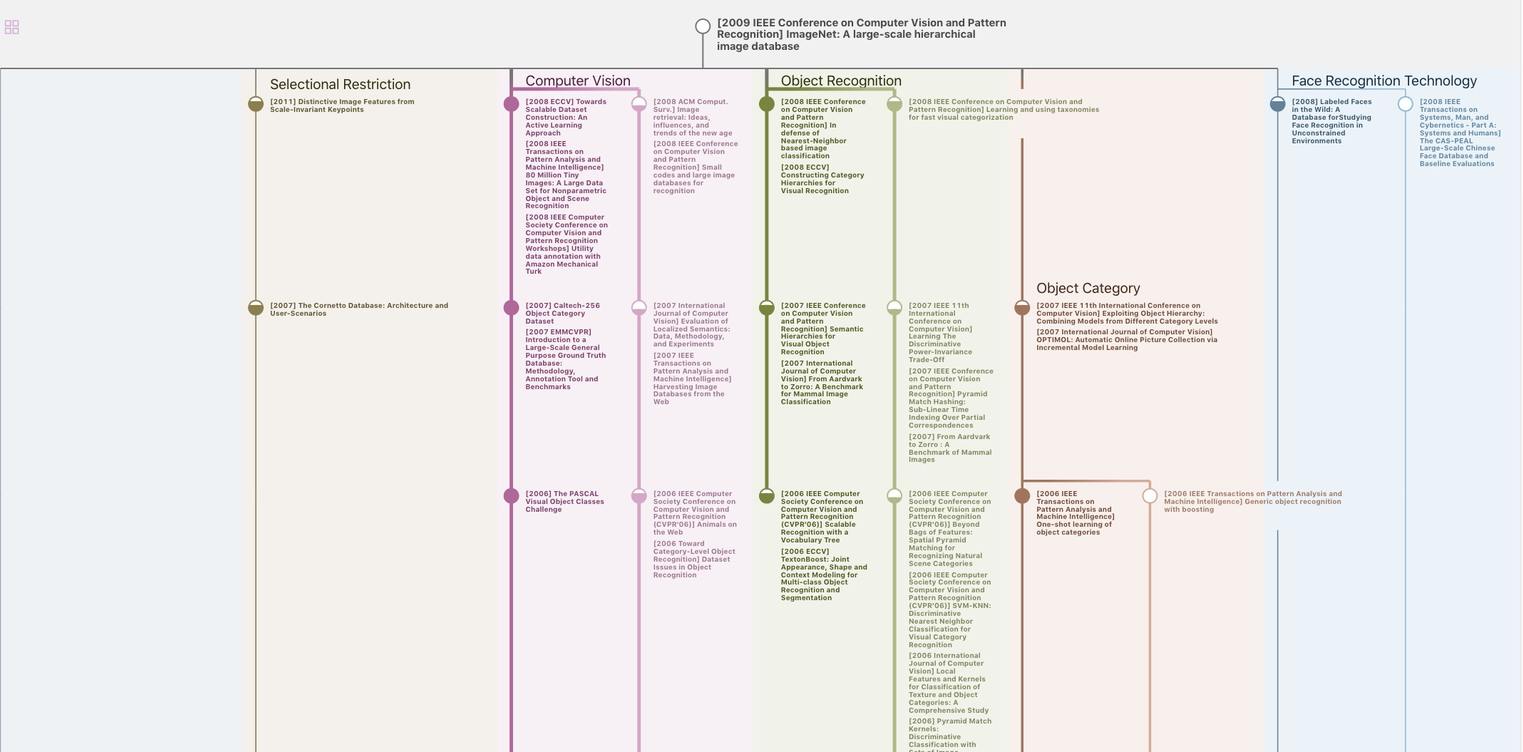
生成溯源树,研究论文发展脉络
Chat Paper
正在生成论文摘要