Generative Model Predictive Control: Approximating MPC Law With Generative Models
IEEE Transactions on Emerging Topics in Computational Intelligence(2024)
摘要
Model Predictive Control (MPC) is a useful solution for control problems with nonlinear characteristics and constraints. However, the online implementation of nonlinear MPC is challenging due to the computational complexity of solving a nonlinear optimization problem online. In this paper, we propose a novel generative approach to approximate a nonlinear MPC with constraints using a mixture density network-based generative model, which is called Generative Model Predictive Control (GMPC) in this paper. GMPC outputs the control input for any given initial state with the maximum likelihood that gives optimal control performance. Namely, the maximum likelihood point lies within the set determined by the nonlinear MPC control law. We provide a sampling-based statistical guarantee for the training of GMPC from the distribution of initial states. The advantages of GMPC over conventional neural network-based controllers are illustrated by numerical results.
更多查看译文
关键词
Generative models,neural networks,nonlinear control systems
AI 理解论文
溯源树
样例
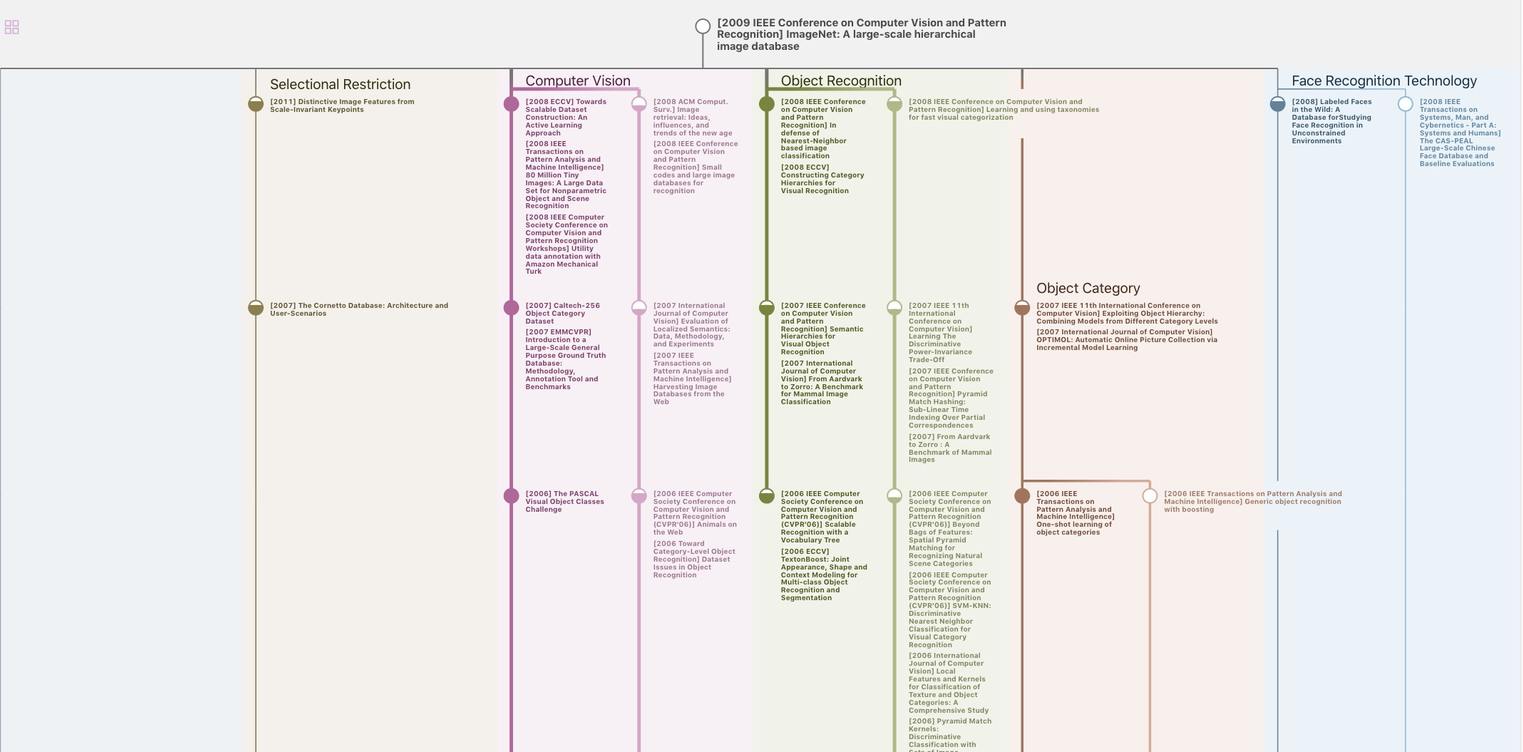
生成溯源树,研究论文发展脉络
Chat Paper
正在生成论文摘要