HAS-RL: A Hierarchical Approximate Scheme Optimized With Reinforcement Learning for NoC-Based NN Accelerators
IEEE TRANSACTIONS ON CIRCUITS AND SYSTEMS I-REGULAR PAPERS(2024)
摘要
Network-on-Chip (NoC) is a scalable on-chip communication architecture for the NN accelerator, but with the increase in the number of nodes, the communication delay becomes higher. Applications such as machine learning have a certain resilience to noisy/erroneous transmitted data. Therefore, approximate communication becomes a promising solution to improving performance by reducing traffic loads under the constraint of the acceptable maximum accuracy loss of neural networks. It is a key issue to balance the result quality and the communication delay for approximate NoC systems. The traditional approximate NoC only considers the node-to-node approximation-based dynamic traffic regulation. However, the dynamically changing traffic patterns across different nodes, different times, and different applications lead to a huge search space, which makes it hard to explore an optimal global approximation solution. In this paper, we propose a quality model for different neural networks, which presents the relationship between the quality loss and the data approximate rate. Then, a hierarchical approximate scheme optimized with reinforcement learning (HAS-RL) is proposed and we reduce the complexity of the HAS-RL by reducing the state space and action space, which will reduce the resource overhead as well. After that, we embed a global approximate controller in the NoC system, in which we deploy a policy network trained with the offline reinforcement learning algorithm to adjust the data approximate rates of each node at run time. Compared with the state-of-the-art method, the proposed scheme reduces the average network delay by 13.5% while their accuracies are similar. The proposed HAS-RL only causes an additional area overhead of 1.24% and power consumption of 0.77% compared with the traditional router design.
更多查看译文
关键词
Delays,Reinforcement learning,Heuristic algorithms,Approximation algorithms,Traffic control,Routing,Regulation,Offline reinforcement learning,neural network,approximate communication,network-on-chip
AI 理解论文
溯源树
样例
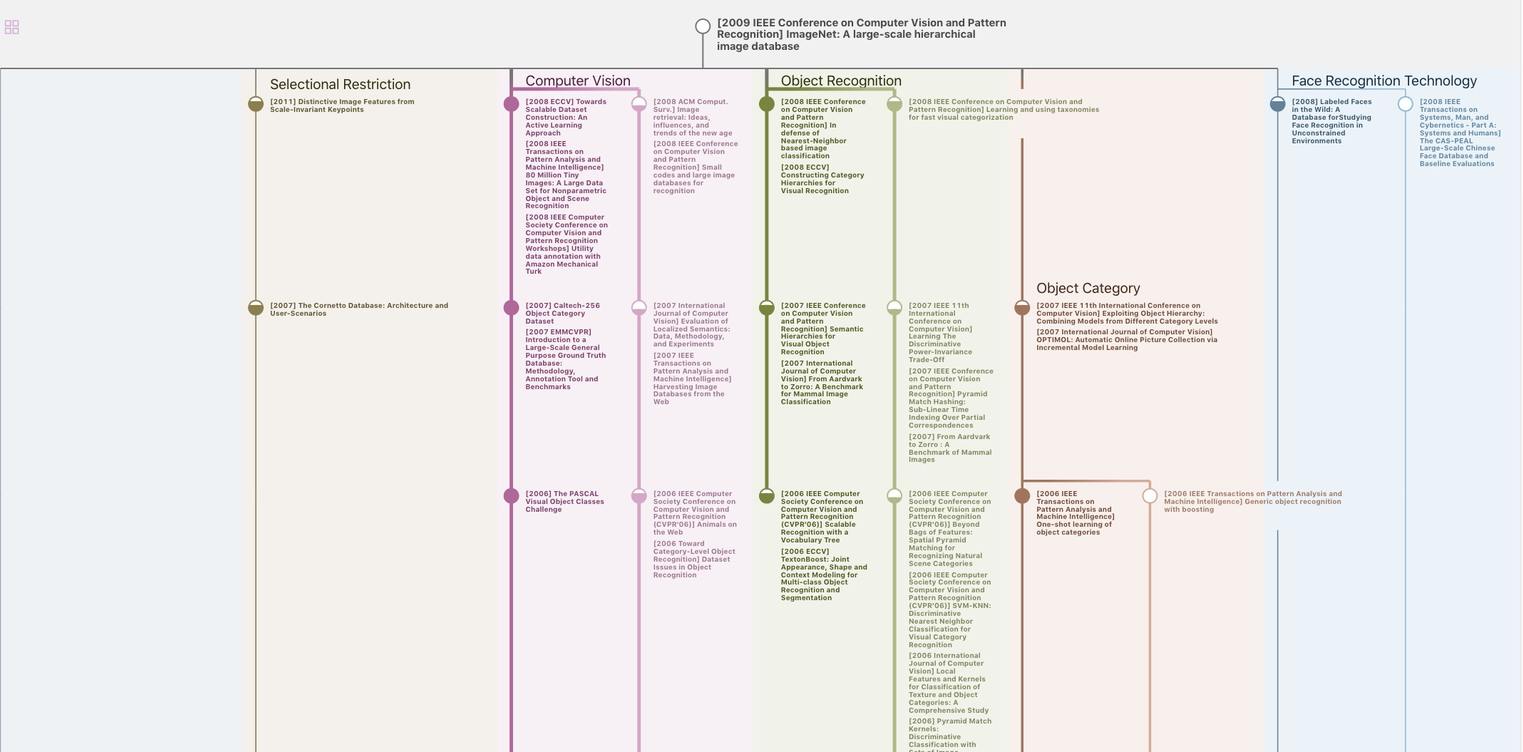
生成溯源树,研究论文发展脉络
Chat Paper
正在生成论文摘要