Max-Min Fairness in RIS-assisted Anti-Jamming Communications: Optimization versus Deep Reinforcement Learning Approaches
IEEE Transactions on Communications(2024)
摘要
Wireless communication is vulnerable to malicious jamming attacks due to the inherent broadcasting nature of wireless channels. This paper investigates an anti-jamming communication system that employs a reconfigurable intelligent surface (RIS) to enhance desired signals and suppress jamming signals. To optimize the system performance while guaranteeing fairness, we maximize the minimum signal-to-interference-plus-noise ratio (SINR) at the legitimate user equipments by jointly optimizing the transmit beamforming vectors at the base station (BS) and the reflecting coefficients at the RIS, subject to the BS’s maximum transmit power constraint and the RIS’s reflecting coefficient constraints. To solve the non-convex max-min-fairness optimization problem, we propose an alternating-optimization (AO)-based approach that alternates between optimizing variables using a second-order-cone program and semi-definite relaxation techniques. Considering the piratical limitation of imperfect jammer-related channel state information (CSI), we also adopt the stochastic successive convex approximation technique for tackling imperfect CSI in the AO-based approach. Furthermore, we propose a deep-reinforcement-learning (DRL)-based solving approach that does not require the jammer-related CSI. Numerical results show that both approaches improve the minimum SINR performance significantly. Although the AO-based approach with real-time CSI slightly outperforms the DRL-based approach with historical CSI, the DRL-based approach uses the trained deep neural network to obtain the beamforming decision directly without solving optimization problems.
更多查看译文
关键词
Anti-jamming communications,reconfigurable intelligent surface,max-min fairness,alternating optimization,deep reinforcement learning
AI 理解论文
溯源树
样例
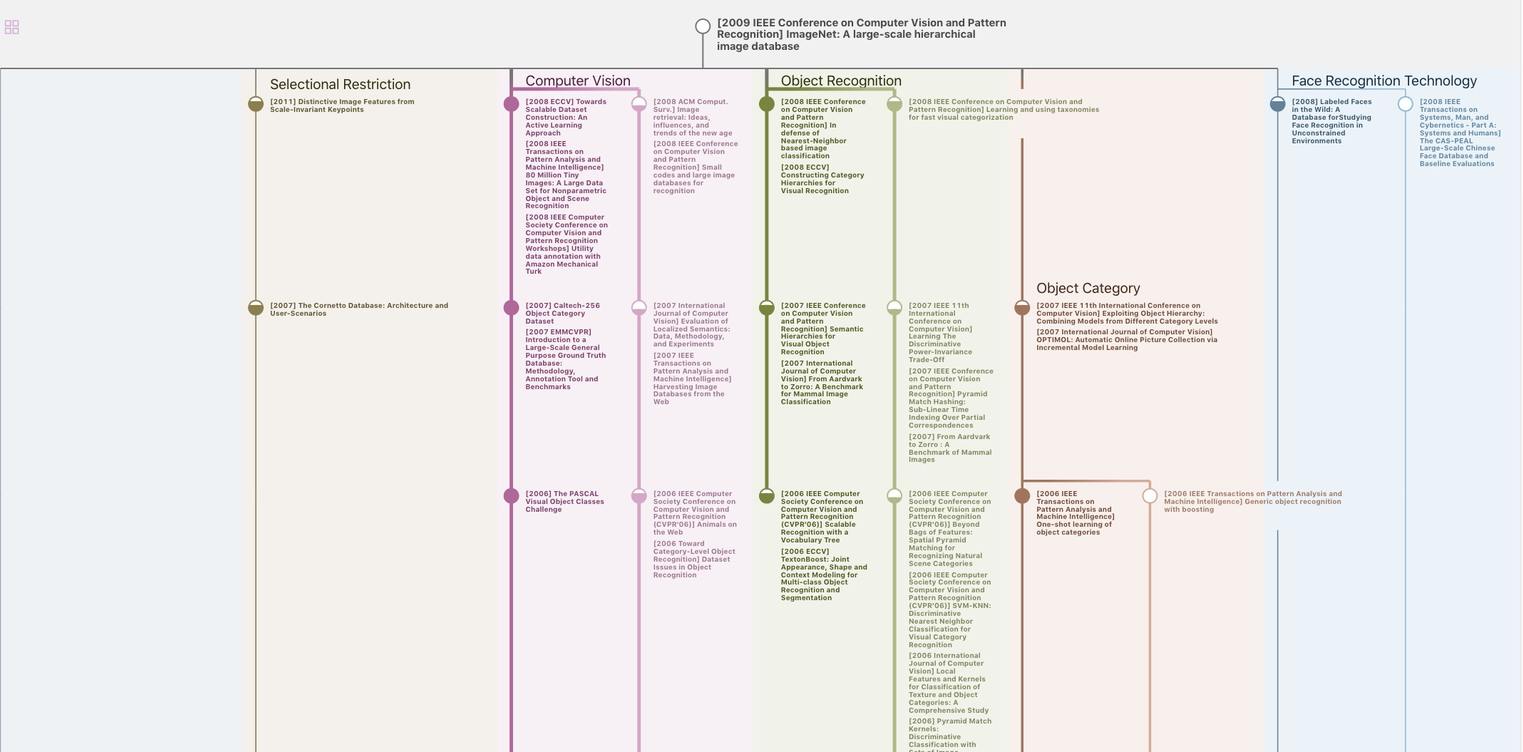
生成溯源树,研究论文发展脉络
Chat Paper
正在生成论文摘要