A Two-Stage Multiband Delay Estimation Scheme via Stochastic Particle-Based Variational Bayesian Inference.
IEEE Internet Things J.(2024)
摘要
Multiband fusion enhances delay estimation by jointly utilizing signals from multiple non-contiguous frequency bands. However, in the multiband signal model, there are many local optimums in the associated likelihood function due to the existence of high frequency component and phase distortion factors, posing challenges for high-accuracy parameter estimation. To address this, we propose a two-stage scheme equipped with different signal models derived from the original model, where the first-stage coarse estimation is performed using a weighted root MUSIC algorithm to narrow down the search range for the subsequent stage, and the second-stage refined estimation utilizes a Bayesian approach to avoid convergence to bad suboptimal solutions. Specifically, we apply the block stochastic successive convex approximation (SSCA) approach to derive a novel stochastic particle-based variational Bayesian inference (SPVBI) algorithm in the refined stage. Unlike conventional particle-based VBI (PVBI) that optimizes only particle probability and incurs exponential per-iteration complexity with particle count, our more flexible SPVBI algorithm optimizes both the position and probability of each particle. Additionally, it utilizes block SSCA to significantly improve sampling efficiency by averaging over iterations, making it suitable for high-dimensional problems. Extensive simulations demonstrate the superiority of our proposed algorithm over various baseline methods.
更多查看译文
关键词
Delay estimation,multiband fusion,variational Bayesian inference,stochastic successive convex approximation
AI 理解论文
溯源树
样例
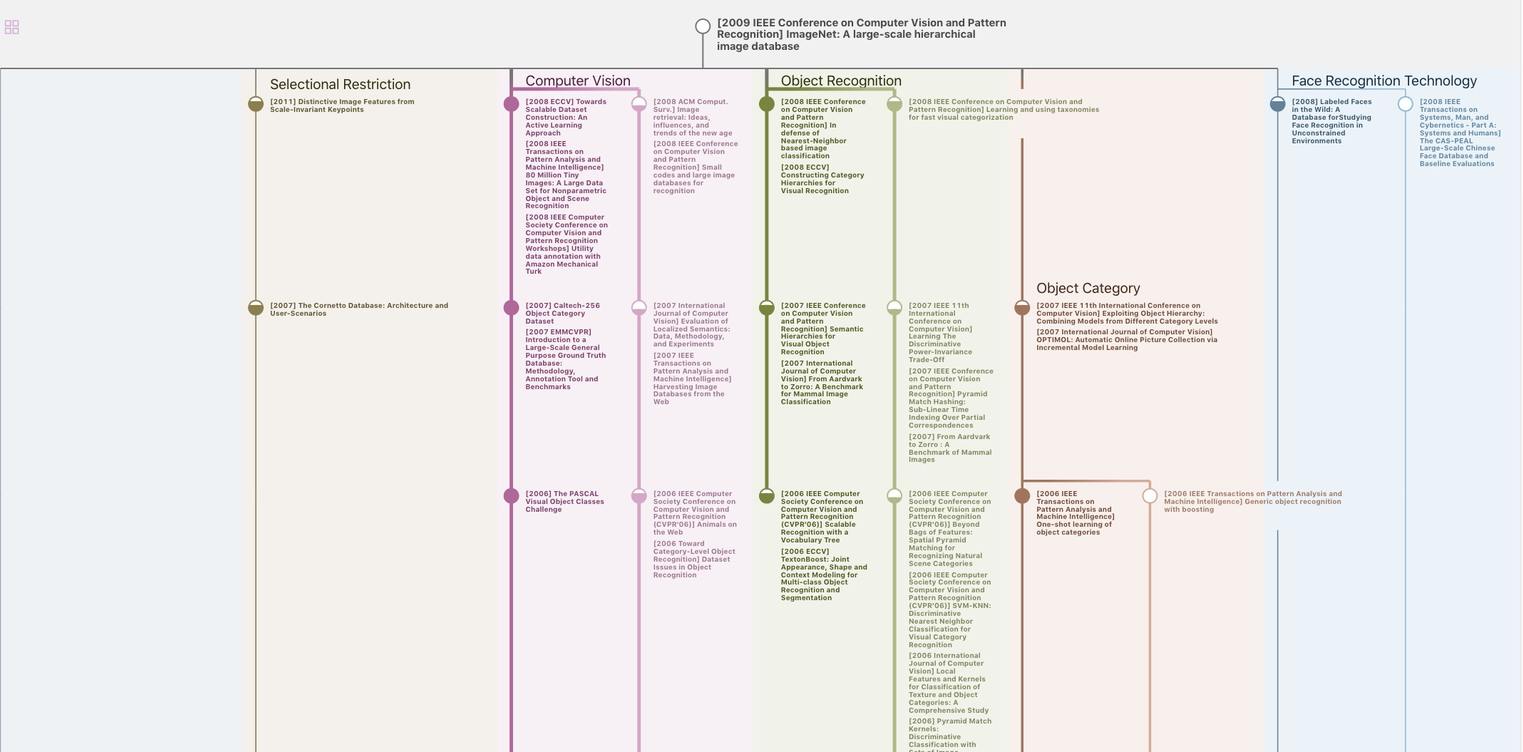
生成溯源树,研究论文发展脉络
Chat Paper
正在生成论文摘要