Mobile Traffic Prediction in Consumer Applications: A Multimodal Deep Learning Approach
IEEE Transactions on Consumer Electronics(2024)
摘要
Mobile traffic prediction is an important yet challenging problem in consumer applications because of the dynamic nature of user behavior, varying application quality of service (QoS) requirements, network congestion, and proliferation of diverse mobile devices. The mobile traffic prediction problem with multiple services, e.g., SMS, call, and Internet, is defined as the mapping from historical traffic data to future traffic prediction. Both grid and graph-based mobile traffic prediction formulations have been extensively considered in the literature. However, an effective multimodal deep learning approach with both grid and graph modals has not yet been fully considered. This study proposes a multimodal convolutional neural network (CNN)-graph neural network (GNN) hybrid framework for single-step mobile traffic prediction, in which the information extracted from SMS, call and Internet services are fused to make a precise prediction for future traffic consumption of consumers in the next hour. The CNN module is built using ConvLSTM, the GNN module is built using adaptive graph convolutional network (AGCN), and a fusion layer is designed to combine the outputs from the CNN and GNN modules. Numerical experiments based on a real-world dataset demonstrate the effectiveness of the proposed framework, which achieves a prediction error lower than ten baselines.
更多查看译文
关键词
Consumer Electronics,Deep Learning,Convolutional Neural Networks,Graph Neural Networks
AI 理解论文
溯源树
样例
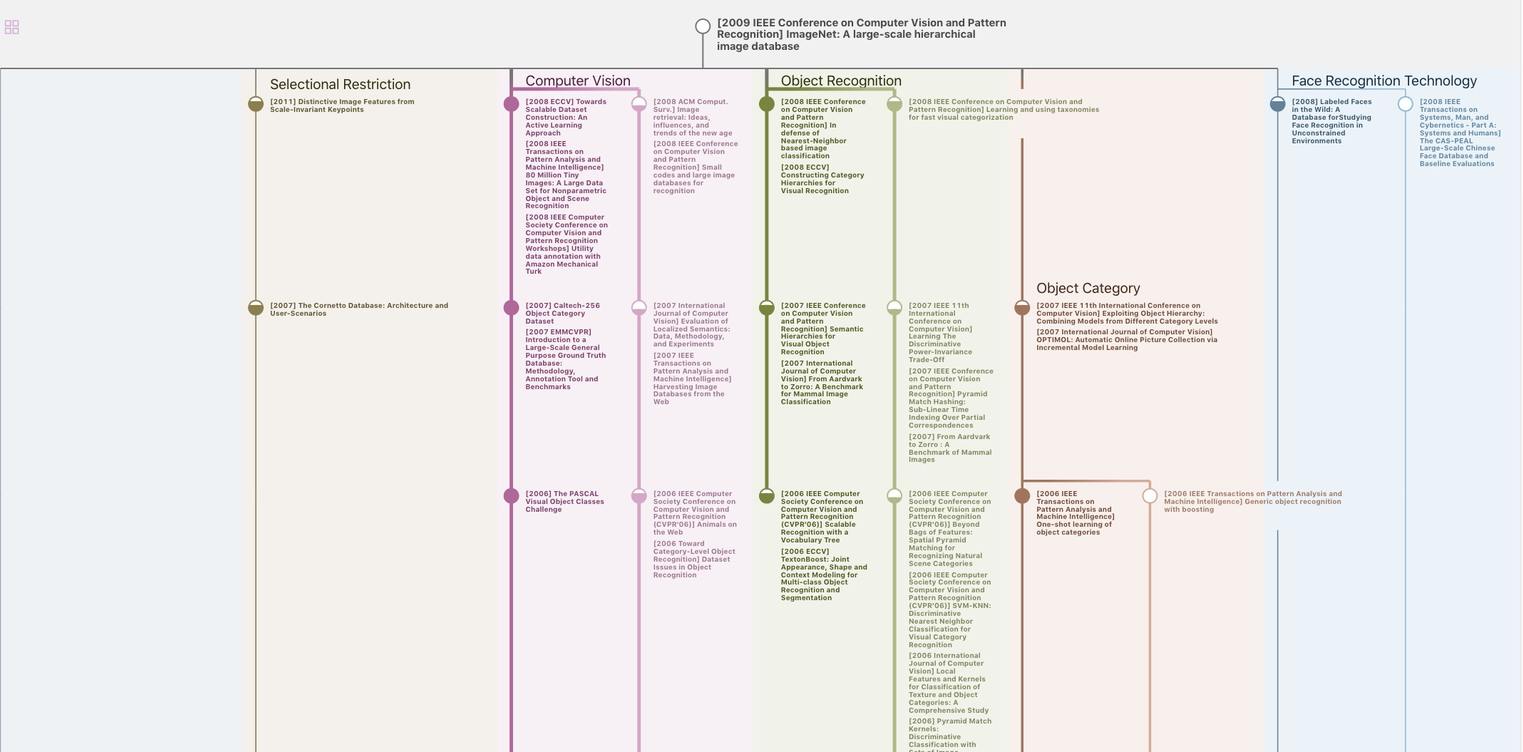
生成溯源树,研究论文发展脉络
Chat Paper
正在生成论文摘要